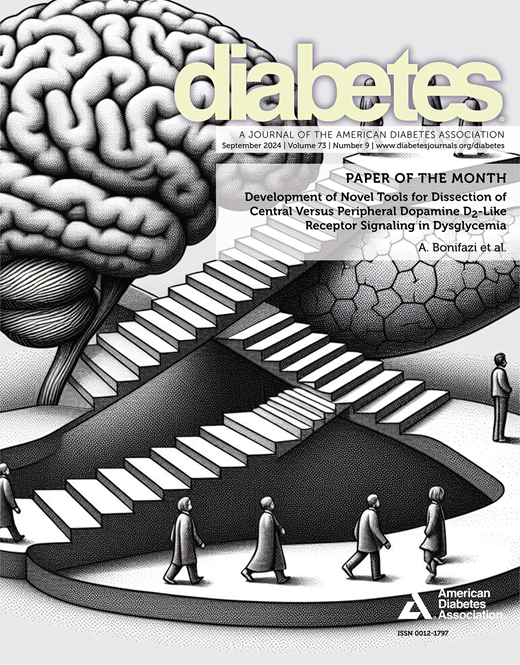
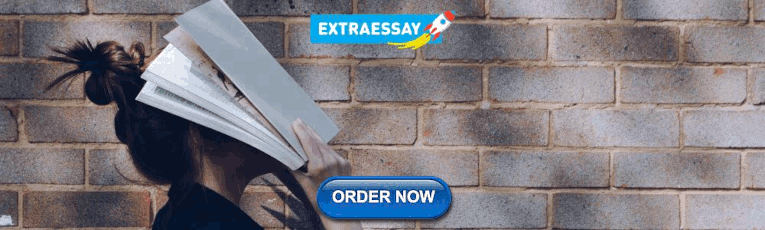
About the Journal
Diabetes publishes original research about the physiology and pathophysiology of diabetes mellitus. Submitted manuscripts can report any aspect of laboratory, animal, or human research. More About Diabetes
Editor in Chief
David A. D'Alessio, MD Duke University
Impact Factor
Journal Statistics
Current Issue Highlights
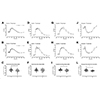
The Longitudinal Effect of Diabetes-Associated Variation in TCF7L2 on Islet Function in Humans
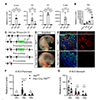
Trefoil Factor 2 Expressed by the Murine Pancreatic Acinar Cells Is Required for the Development of Islets and for β-Cell Function During Aging
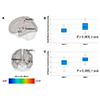
Increased Thalamocortical Functional Connectivity on Discontinuation of Treatment in Painful Diabetic Peripheral Neuropathy
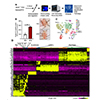
The Role of Activator Protein-1 Complex in Diabetes-Associated Atherosclerosis: Insights From Single-Cell RNA Sequencing
Access the complete Table of Contents .
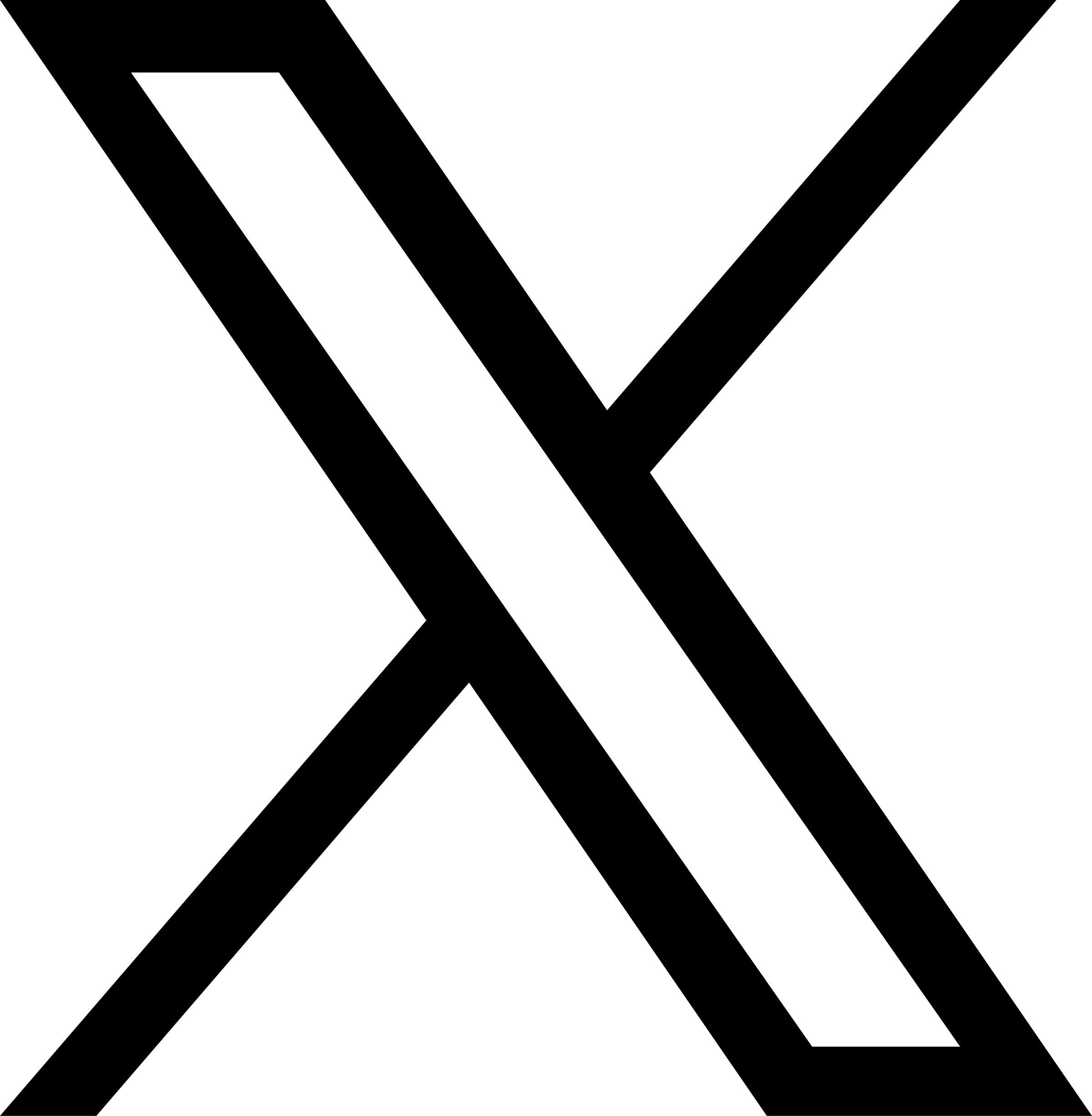
Follow Diabetes on X
Join us on X @Diabetes_ADA to connect with the diabetes research community and share ideas with fellow professionals. Find the newest published articles, latest podcasts, new professional books, and up-to-date diabetes news. You can also follow ADA Publications on Facebook @adaPublications .
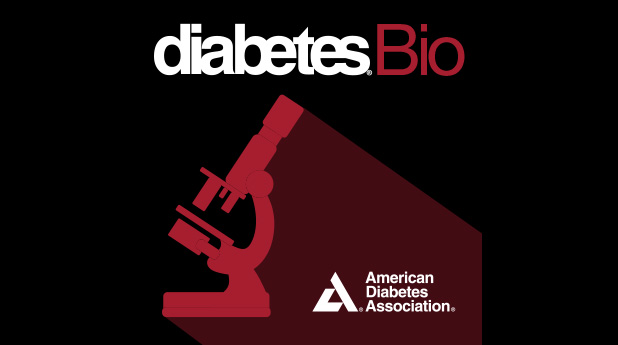
New Podcast: DiabetesBio
DiabetesBio, the American Diabetes Association's monthly podcast, features Drs. Kirk Habegger, Darleen Sandoval, and Kevin Williams interviewing authors of key articles from the journal Diabetes .
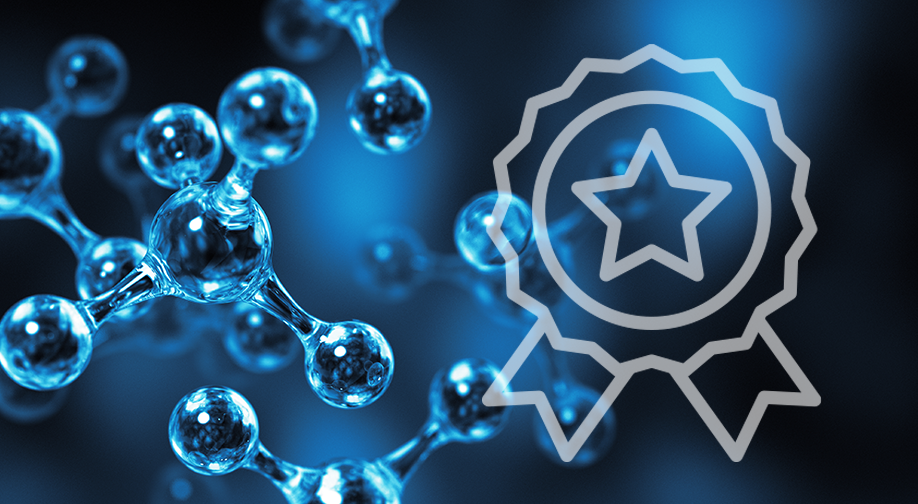
Paper of the Month
Each month, the Editors of Diabetes select an original research article to designate Paper of the Month. View these exclusive articles in a freely accessible special article collection.
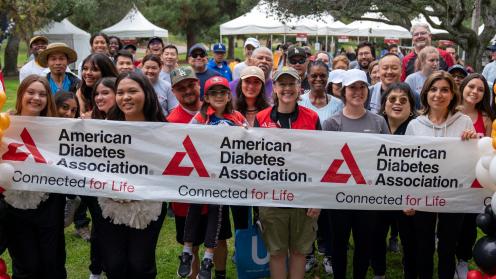
Pathway to Stop Diabetes
The ADA vision: find a new generation of scientists at the peak of their creativity and provide them the freedom, autonomy, and financial and professional resources for breakthrough discoveries.
- Online ISSN 1939-327X
- Print ISSN 0012-1797
- Diabetes Care
- Clinical Diabetes
- Diabetes Spectrum
- Standards of Medical Care in Diabetes
- Scientific Sessions Abstracts
- BMJ Open Diabetes Research & Care
- ShopDiabetes.org
- ADA Professional Books
Clinical Compendia
- Clinical Compendia Home
- Latest News
- DiabetesPro SmartBrief
- Special Collections
- DiabetesPro®
- Diabetes Food Hub™
- Insulin Affordability
- Know Diabetes By Heart™
- About the ADA
- Journal Policies
- For Reviewers
- Advertising in ADA Journals
- Reprints and Permission for Reuse
- Copyright Notice/Public Access Policy
- ADA Professional Membership
- ADA Member Directory
- Diabetes.org
- X (Twitter)
- Cookie Policy
- Accessibility
- Terms & Conditions
- Get Adobe Acrobat Reader
- © Copyright American Diabetes Association
This Feature Is Available To Subscribers Only
Sign In or Create an Account
HYPOTHESIS AND THEORY article
Type 2 diabetes mellitus: a pathophysiologic perspective.
- Department of Medicine, Duke University, Durham, NC, United States
Type 2 Diabetes Mellitus (T2DM) is characterized by chronically elevated blood glucose (hyperglycemia) and elevated blood insulin (hyperinsulinemia). When the blood glucose concentration is 100 milligrams/deciliter the bloodstream of an average adult contains about 5–10 grams of glucose. Carbohydrate-restricted diets have been used effectively to treat obesity and T2DM for over 100 years, and their effectiveness may simply be due to lowering the dietary contribution to glucose and insulin levels, which then leads to improvements in hyperglycemia and hyperinsulinemia. Treatments for T2DM that lead to improvements in glycemic control and reductions in blood insulin levels are sensible based on this pathophysiologic perspective. In this article, a pathophysiological argument for using carbohydrate restriction to treat T2DM will be made.
Introduction
Type 2 Diabetes Mellitus (T2DM) is characterized by a persistently elevated blood glucose, or an elevation of blood glucose after a meal containing carbohydrate ( 1 ) ( Table 1 ). Unlike Type 1 Diabetes which is characterized by a deficiency of insulin, most individuals affected by T2DM have elevated insulin levels (fasting and/or post glucose ingestion), unless there has been beta cell failure ( 2 , 3 ). The term “insulin resistance” (IR) has been used to explain why the glucose levels remain elevated even though there is no deficiency of insulin ( 3 , 4 ). Attempts to determine the etiology of IR have involved detailed examinations of molecular and intracellular pathways, with attribution of cause to fatty acid flux, but the root cause has been elusive to experts ( 5 – 7 ).

Table 1 . Definition of type 2 diabetes mellitus.
How Much Glucose Is in the Blood?
Keeping in mind that T2DM involves an elevation of blood glucose, it is important to understand how much glucose is in the blood stream to begin with, and then the factors that influence the blood glucose—both exogenous and endogenous factors. The amount of glucose in the bloodstream is carefully controlled—approximately 5–10 grams in the bloodstream at any given moment, depending upon the size of the person. To calculate this, multiply 100 milligrams/deciliter × 1 gram/1,000 milligrams × 10 deciliters/1 liter × 5 liters of blood. The “zeros cancel” and you are left with 5 grams of glucose if the individual has 5 liters of blood. Since red blood cells represent about 40% of the blood volume, and the glucose is in equilibrium, there may be an extra 40% glucose because of the red blood cell reserve ( 8 ). Adding the glucose from the serum and red blood cells totals about 5–10 grams of glucose in the entire bloodstream.
Major Exogenous Factors That Raise the Blood Glucose
Dietary carbohydrate is the major exogenous factor that raises the blood glucose. When one considers that it is common for an American in 2021 to consume 200–300 grams of carbohydrate daily, and most of this carbohydrate is digested and absorbed as glucose, the body absorbs and delivers this glucose via the bloodstream to the cells while attempting to maintain a normal blood glucose level. Thinking of it in this way, if 200–300 grams of carbohydrates is consumed in a day, the bloodstream that holds 5–10 grams of glucose and has a concentration of 100 milligrams/deciliter, is the conduit through which 200,000–300,000 milligrams (200 grams = 200,000 milligrams) passes over the course of a day.
Major Endogenous Factors That Raise the Blood Glucose
There are many endogenous contributors that raise the blood glucose. There are at least 3 different hormones that increase glucose levels: glucagon, epinephrine, and cortisol. These hormones increase glucose levels by increasing glycogenolysis and gluconeogenesis ( 9 ). Without any dietary carbohydrate, the normal human body can generate sufficient glucose though the mechanism of glucagon secretion, gluconeogenesis, glycogen storage and glycogenolysis ( 10 ).
Major Exogenous Factors That Lower the Blood Glucose
A reduction in dietary carbohydrate intake can lower the blood glucose. An increase in activity or exercise usually lowers the blood glucose ( 11 ). There are many different medications, employing many mechanisms to lower the blood glucose. Medications can delay sucrose and starch absorption (alpha-glucosidase inhibitors), slow gastric emptying (GLP-1 agonists, DPP-4 inhibitors) enhance insulin secretion (sulfonylureas, meglitinides, GLP-1 agonists, DPP-4 inhibitors), reduce gluconeogenesis (biguanides), reduce insulin resistance (biguanides, thiazolidinediones), and increase urinary glucose excretion (SGLT-2 inhibitors). The use of medications will also have possible side effects.
Major Endogenous Factors That Lower the Blood Glucose
The major endogenous mechanism to lower the blood glucose is to deliver glucose into the cells (all cells can use glucose). If the blood glucose exceeds about 180 milligrams/deciliter, then loss of glucose into the urine can occur. The blood glucose is reduced by cellular uptake using glut transporters ( 12 ). Some cells have transporters that are responsive to the presence of insulin to activate (glut4), others have transporters that do not require insulin for activation. Insulin-responsive glucose transporters in muscle cells and adipose cells lead to a reduction in glucose levels—especially after carbohydrate-containing meals ( 13 ). Exercise can increase the glucose utilization in muscle, which then increases glucose cellular uptake and reduce the blood glucose levels. During exercise, when the metabolic demands of skeletal muscle can increase more than 100-fold, and during the absorptive period (after a meal), the insulin-responsive glut4 transporters facilitate the rapid entry of glucose into muscle and adipose tissue, thereby preventing large fluctuations in blood glucose levels ( 13 ).
Which Cells Use Glucose?
Glucose can used by all cells. A limited number of cells can only use glucose, and are “glucose-dependent.” It is generally accepted that the glucose-dependent cells include red blood cells, white blood cells, and cells of the renal papilla. Red blood cells have no mitochondria for beta-oxidation, so they are dependent upon glucose and glycolysis. White blood cells require glucose for the respiratory burst when fighting infections. The cells of the inner renal medulla (papilla) are under very low oxygen tension, so therefore must predominantly use glucose and glycolysis. The low oxygen tension is a result of the countercurrent mechanism of urinary concentration ( 14 ). These glucose-dependent cells have glut transporters that do not require insulin for activation—i.e., they do not need insulin to get glucose into the cells. Some cells can use glucose and ketones, but not fatty acids. The central nervous system is believed to be able to use glucose and ketones for fuel ( 15 ). Other cells can use glucose, ketones, and fatty acids for fuel. Muscle, even cardiac muscle, functions well on fatty acids and ketones ( 16 ). Muscle cells have both non-insulin-responsive and insulin-responsive (glut4) transporters ( 12 ).
Possible Dual Role of an Insulin-Dependent Glucose-Transporter (glut4)
A common metaphor is to think of the insulin/glut transporter system as a key/lock mechanism. Common wisdom states that the purpose of insulin-responsive glut4 transporters is to facilitate glucose uptake when blood insulin levels are elevated. But, a lock serves two purposes: to let someone in and/or to keep someone out . So, one of the consequences of the insulin-responsive glut4 transporter is to keep glucose out of the muscle and adipose cells, too, when insulin levels are low. The cells that require glucose (“glucose-dependent”) do not need insulin to facilitate glucose entry into the cell (non-insulin-responsive transporters). In a teleological way, it would “make no sense” for cells that require glucose to have insulin-responsive glut4 transporters. Cells that require glucose have glut1, glut2, glut3, glut5 transporters—none of which are insulin-responsive (Back to the key/lock metaphor, it makes no sense to have a lock on a door that you want people to go through). At basal (low insulin) conditions, most glucose is used by the brain and transported by non-insulin-responsive glut1 and glut3. So, perhaps one of the functions of the insulin-responsive glucose uptake in muscle and adipose to keep glucose OUT of the these cells at basal (low insulin) conditions, so that the glucose supply can be reserved for the tissue that is glucose-dependent (blood cells, renal medulla).
What Causes IR and T2DM?
The current commonly espoused view is that “Type 2 diabetes develops when beta-cells fail to secrete sufficient insulin to keep up with demand, usually in the context of increased insulin resistance.” ( 17 ). Somehow, the beta cells have failed in the face of insulin resistance. But what causes insulin resistance? When including the possibility that the environment may be part of the problem, is it possible that IR is an adaptive (protective) response to excess glucose availability? From the perspective that carbohydrate is not an essential nutrient and the change in foods in recent years has increased the consumption of refined sugar and flour, maybe hyperinsulinemia is the cause of IR and T2DM, as cells protect themselves from excessive glucose and insulin levels.
Insulin Is Already Elevated in IR and T2DM
Clinical experience of most physicians using insulin to treat T2DM over time informs us that an escalation of insulin dose is commonly needed to achieve glycemic control (when carbohydrate is consumed). When more insulin is given to someone with IR, the IR seems to get worse and higher levels of insulin are needed. I have the clinical experience of treating many individuals affected by T2DM and de-prescribing insulin as it is no longer needed after consuming a diet without carbohydrate ( 18 ).
Diets Without Carbohydrate Reverse IR and T2DM
When dietary manipulation was the only therapy for T2DM, before medications were available, a carbohydrate-restricted diet was used to treat T2DM ( 19 – 21 ). Clinical experience of obesity medicine physicians and a growing number of recent studies have demonstrated that carbohydrate-restricted diets reverse IR and T2DM ( 18 , 22 , 23 ). Other methods to achieve caloric restriction also have these effects, like calorie-restricted diets and bariatric surgery ( 24 , 25 ). There may be many mechanisms by which these approaches may work: a reduction in glucose, a reduction in insulin, nutritional ketosis, a reduction in metabolic syndrome, or a reduction in inflammation ( 26 ). Though there may be many possible mechanisms, let's focus on an obvious one: a reduction in blood glucose. Let's assume for a moment that the excessive glucose and insulin leads to hyperinsulinemia and this is the cause of IR. On a carbohydrate-restricted diet, the reduction in blood glucose leads to a reduction in insulin. The reduction in insulin leads to a reduction in insulin resistance. The reduction in insulin leads to lipolysis. The resulting lowering of blood glucose, insulin and body weight reverses IR, T2DM, AND obesity. These clinical observations strongly suggest that hyperinsulinemia is a cause of IR and T2DM—not the other way around.
What Causes Atherosclerosis?
For many years, the metabolic syndrome has been described as a possible cause of atherosclerosis, but there are no RCTs directly targeting metabolic syndrome, and the current drug treatment focuses on LDL reduction, so its importance remains controversial. A recent paper compared the relative importance of many risk factors in the prediction of the first cardiac event in women, and the most powerful predictors were diabetes, metabolic syndrome, smoking, hypertension and BMI ( 27 ). The connection between dietary carbohydrate and fatty liver is well-described ( 28 ). The connection between fatty liver and atherosclerosis is well-described ( 29 ). It is very possible that the transport of excess glucose to the adipose tissue via lipoproteins creates the particles that cause the atherosclerotic damage (small LDL) ( Figure 1 ) ( 30 – 32 ). This entire process of dietary carbohydrate leading to fatty liver, leading to small LDL, is reversed by a diet without carbohydrate ( 26 , 33 , 34 ).
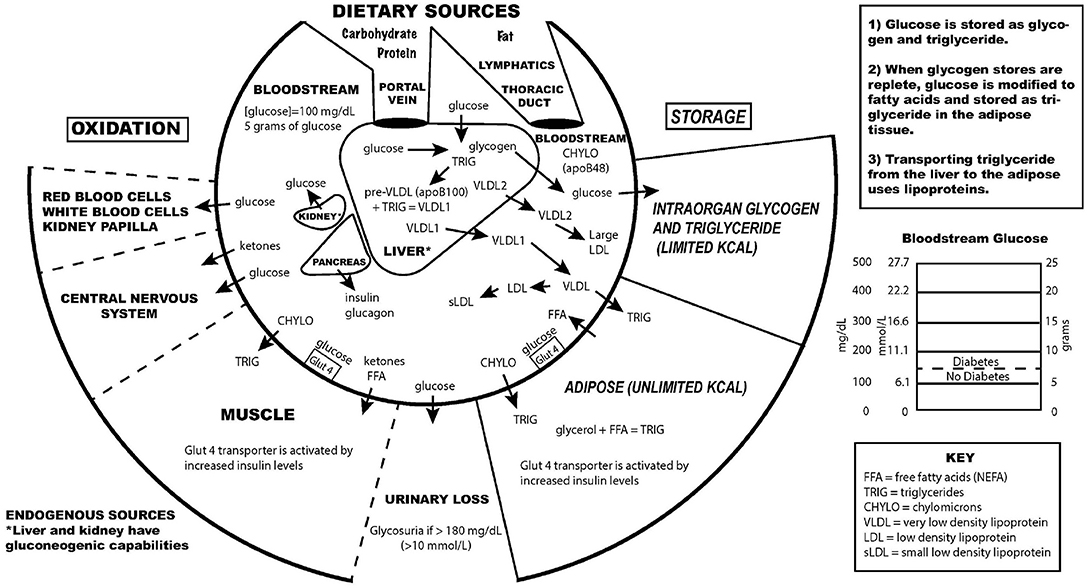
Figure 1 . Key aspects of the interconnection between glucose and lipoprotein metabolism.
Reducing dietary carbohydrate in the context of a low carbohydrate, ketogenic diet reduces hyperglycemia and hyperinsulinemia, IR and T2DM. In the evaluation of an individual for a glucose abnormality, measure the blood glucose and insulin levels. If the insulin level (fasting or after a glucose-containing meal) is high, do not give MORE insulin—instead, use an intervention to lower the insulin levels. Effective ways to reduce insulin resistance include lifestyle, medication, and surgical therapies ( 23 , 35 ).
The search for a single cause of a complex problem is fraught with difficulty and controversy. I am not hypothesizing that excessive dietary carbohydrate is the only cause of IR and T2DM, but that it is a cause, and quite possibly the major cause. How did such a simple explanation get overlooked? I believe it is very possible that the reductionistic search for intracellular molecular mechanisms of IR and T2DM, the emphasis on finding pharmaceutical (rather than lifestyle) treatments, the emphasis on the treatment of high total and LDL cholesterol, and the fear of eating saturated fat may have misguided a generation of researchers and clinicians from the simple answer that dietary carbohydrate, when consumed chronically in amounts that exceeds an individual's ability to metabolize them, is the most common cause of IR, T2DM and perhaps even atherosclerosis.
While there has historically been a concern about the role of saturated fat in the diet as a cause of heart disease, most nutritional experts now cite the lack of evidence implicating dietary saturated fat as the reason for lack of concern of it in the diet ( 36 ).
The concept of comparing medications that treat IR by insulin-sensitizers or by providing insulin itself was tested in the Bari-2D study ( 37 ). Presumably in the context of consuming a standard American diet, this study found no significant difference in death rates or major cardiovascular events between strategies of insulin sensitization or insulin provision.
While lifestyle modification may be ideal to prevent or cure IR and T2DM, for many people these changes are difficult to learn and/or maintain. Future research should be directed toward improving adherence to all effective lifestyle or medication treatments. Future research is also needed to assess the effect of carbohydrate restriction on primary or secondary prevention of outcomes of cardiovascular disease.
Data Availability Statement
The original contributions presented in the study are included in the article/supplementary material, further inquiries can be directed to the corresponding author/s.
Author Contributions
The author confirms being the sole contributor of this work and has approved it for publication.
Conflict of Interest
EW receives royalties from popular diet books and is founder of a company based on low-carbohydrate diet principles (Adapt Your Life, Inc.).
Publisher's Note
All claims expressed in this article are solely those of the authors and do not necessarily represent those of their affiliated organizations, or those of the publisher, the editors and the reviewers. Any product that may be evaluated in this article, or claim that may be made by its manufacturer, is not guaranteed or endorsed by the publisher.
1. American Diabetes Association. Classification and diagnosis of diabetes. Diabetes Care . (2016) 39 (Suppl. 1):S13–22. doi: 10.2337/dc16-S005
PubMed Abstract | CrossRef Full Text | Google Scholar
2. Bogardus C, Lillioja S, Howard BV, Reaven G, Mott D. Relationships between insulin secretion, insulin action, and fasting plasma glucose concentration in nondiabetic and noninsulin-dependent diabetic subjects. J Clin Invest. (1984) 74:1238–46. doi: 10.1172/JCI111533
3. Reaven GM. Compensatory hyperinsulinemia and the development of an atherogenic lipoprotein profile: the price paid to maintain glucose homeostasis in insulin-resistant individuals. Endocrinol Metab Clin North Am. (2005) 34:49–62. doi: 10.1016/j.ecl.2004.12.001
4. DeFronzo RA, Ferrannini E. Insulin resistance. A multifaceted syndrome responsible for NIDDM, obesity, hypertension, dyslipidemia, and atherosclerotic cardiovascular disease. Diabetes Care. (1991) 14:173–94. doi: 10.2337/diacare.14.3.173
5. Eckel RH, Grundy SM, Zimmet PZ. The metabolic syndrome. Lancet. (2005) 365:1415–28. doi: 10.1016/S0140-6736(05)66378-7
6. Yaribeygi H, Farrokhi FR, Butler AE, Sahebkar A. Insulin resistance: review of the underlying molecular mechanisms. J Cell Physiol. (2019) 234:8152–61. doi: 10.1002/jcp.27603
7. Shulman GI. Cellular mechanisms of insulin resistance. J Clin Invest. (2000) 106:171–6. doi: 10.1172/JCI10583
8. Guizouarn H, Allegrini B. Erythroid glucose transport in health and disease. Pflugers Arch. (2020) 472:1371–83. doi: 10.1007/s00424-020-02406-0
9. Petersen MC, Vatner DF, Shulman GI. Regulation of hepatic glucose metabolism in health and disease. Nat Rev Endocrinol. (2017) 13:572–87. doi: 10.1038/nrendo.2017.80
10. Tondt J, Yancy WS, Westman EC. Application of nutrient essentiality criteria to dietary carbohydrates. Nutr Res Rev. (2020) 33:260–70. doi: 10.1017/S0954422420000050
11. Colberg SR, Hernandez MJ, Shahzad F. Blood glucose responses to type, intensity, duration, and timing of exercise. Diabetes Care. (2013) 36:e177. doi: 10.2337/dc13-0965
12. Mueckler M, Thorens B. The SLC2 (GLUT) family of membrane transporters. Mol Aspects Med. (2013) 34:121–38. doi: 10.1016/j.mam.2012.07.001
13. Bryant NJ, Govers R, James DE. Regulated transport of the glucose transporter GLUT4. Nat Rev Mol Cell Biol. (2002) 3:267–77. doi: 10.1038/nrm782
14. Epstein FH. Oxygen and renal metabolism. Kidney Int. (1997) 51:381–5. doi: 10.1038/ki.1997.50
15. Cahill GF. Fuel metabolism in starvation. Annu Rev Nutr. (2006) 26:1–22. doi: 10.1146/annurev.nutr.26.061505.111258
16. Murashige D, Jang C, Neinast M, Edwards JJ, Cowan A, Hyman MC, et al. Comprehensive quantification of fuel use by the failing and nonfailing human heart. Science. (2020) 370:364–8. doi: 10.1126/science.abc8861
17. Skyler JS, Bakris GL, Bonifacio E, Darsow T, Eckel RH, Groop L, et al. Differentiation of diabetes by pathophysiology, natural history, and prognosis. Diabetes. (2017) 66:241–55. doi: 10.2337/db16-0806
18. Westman EC, Yancy WS, Mavropoulos JC, Marquart M, McDuffie JR. The effect of a low-carbohydrate, ketogenic diet versus a low-glycemic index diet on glycemic control in type 2 diabetes mellitus. Nutr Metab. (2008) 5:36. doi: 10.1186/1743-7075-5-36
CrossRef Full Text | Google Scholar
19. Allen F. The treatment of diabetes. Boston Med Surg J. (1915) 172:241–7. doi: 10.1056/NEJM191502181720702
20. Osler W, McCrae T. The Principles and Practice of Medicine . 9th ed. New York and London: Appleton & Company (1923).
21. Lennerz BS, Koutnik AP, Azova S, Wolfsdorf JI, Ludwig DS. Carbohydrate restriction for diabetes: rediscovering centuries-old wisdom. J Clin Invest. (2021) 131:e142246. doi: 10.1172/JCI142246
22. Steelman GM, Westman EC. Obesity: Evaluation and Treatment Essentials . 2nd ed. Boca Raton: CRC Press, Taylor & Francis Group (2016). 340 p.
23. Athinarayanan SJ, Adams RN, Hallberg SJ, McKenzie AL, Bhanpuri NH, Campbell WW, et al. Long-term effects of a novel continuous remote care intervention including nutritional ketosis for the management of type 2 diabetes: a 2-year non-randomized clinical trial. Front Endocrinol. (2019) 10:348. doi: 10.3389/fendo.2019.00348
24. Lim EL, Hollingsworth KG, Aribisala BS, Chen MJ, Mathers JC, Taylor R. Reversal of type 2 diabetes: normalisation of beta cell function in association with decreased pancreas and liver triacylglycerol. Diabetologia. (2011) 54:2506–14. doi: 10.1007/s00125-011-2204-7
25. Isbell JM, Tamboli RA, Hansen EN, Saliba J, Dunn JP, Phillips SE, et al. The importance of caloric restriction in the early improvements in insulin sensitivity after Roux-en-Y gastric bypass surgery. Diabetes Care. (2010) 33:1438–42. doi: 10.2337/dc09-2107
26. Bhanpuri NH, Hallberg SJ, Williams PT, McKenzie AL, Ballard KD, Campbell WW, et al. Cardiovascular disease risk factor responses to a type 2 diabetes care model including nutritional ketosis induced by sustained carbohydrate restriction at 1 year: an open label, non-randomized, controlled study. Cardiovasc Diabetol. (2018) 17:56. doi: 10.1186/s12933-018-0698-8
27. Dugani SB, Moorthy MV, Li C, Demler OV, Alsheikh-Ali AA, Ridker PM, et al. Association of lipid, inflammatory, and metabolic biomarkers with age at onset for incident coronary heart disease in women. JAMA Cardiol. (2021) 6:437–47. doi: 10.1001/jamacardio.2020.7073
28. Duwaerts CC, Maher JJ. Macronutrients and the adipose-liver axis in obesity and fatty liver. Cell Mol Gastroenterol Hepatol. (2019) 7:749–61. doi: 10.1016/j.jcmgh.2019.02.001
29. Zhang L, She Z-G, Li H, Zhang X-J. Non-alcoholic fatty liver disease: a metabolic burden promoting atherosclerosis. Clin Sci Lond Engl. (1979) 134:1775–99. doi: 10.1042/CS20200446
30. Horton TJ, Drougas H, Brachey A, Reed GW, Peters JC, Hill JO. Fat and carbohydrate overfeeding in humans: different effects on energy storage. Am J Clin Nutr. (1995) 62:19–29. doi: 10.1093/ajcn/62.1.19
31. Packard C, Caslake M, Shepherd J. The role of small, dense low density lipoprotein (LDL): a new look. Int J Cardiol. (2000) 74 (Suppl. 1):S17–22. doi: 10.1016/S0167-5273(99)00107-2
32. Borén J, Chapman MJ, Krauss RM, Packard CJ, Bentzon JF, Binder CJ, et al. Low-density lipoproteins cause atherosclerotic cardiovascular disease: pathophysiological, genetic, and therapeutic insights: a consensus statement from the European Atherosclerosis Society Consensus Panel. Eur Heart J. (2020) 41:2313–30. doi: 10.1093/eurheartj/ehz962
33. Yancy WS, Olsen MK, Guyton JR, Bakst RP, Westman EC. A low-carbohydrate, ketogenic diet versus a low-fat diet to treat obesity and hyperlipidemia: a randomized, controlled trial. Ann Intern Med. (2004) 140:769. doi: 10.7326/0003-4819-140-10-200405180-00006
34. Tendler D, Lin S, Yancy WS, Mavropoulos J, Sylvestre P, Rockey DC, et al. The effect of a low-carbohydrate, ketogenic diet on nonalcoholic fatty liver disease: a pilot study. Dig Dis Sci. (2007) 52:589–93. doi: 10.1007/s10620-006-9433-5
35. Pories WJ, Swanson MS, MacDonald KG, Long SB, Morris PG, Brown BM, et al. Who would have thought it? An operation proves to be the most effective therapy for adult-onset diabetes mellitus. Ann Surg. (1995) 222:339–50. doi: 10.1097/00000658-199509000-00011
36. Astrup A, Magkos F, Bier DM, Brenna JT, de Oliveira Otto MC, Hill JO, et al. Saturated fats and health: a reassessment and proposal for food-based recommendations: JACC state-of-the-art review. J Am Coll Cardiol. (2020) 76:844–57. doi: 10.1016/j.jacc.2020.05.077
37. A randomized trial of therapies for type 2 diabetes and coronary artery disease. N Engl J Med . (2009) 360:2503–15. doi: 10.1056/NEJMoa0805796
Keywords: type 2 diabetes, insulin resistance, pre-diabetes, carbohydrate-restricted diets, hyperinsulinemia, hyperglycemia
Citation: Westman EC (2021) Type 2 Diabetes Mellitus: A Pathophysiologic Perspective. Front. Nutr. 8:707371. doi: 10.3389/fnut.2021.707371
Received: 09 May 2021; Accepted: 20 July 2021; Published: 10 August 2021.
Reviewed by:
Copyright © 2021 Westman. This is an open-access article distributed under the terms of the Creative Commons Attribution License (CC BY) . The use, distribution or reproduction in other forums is permitted, provided the original author(s) and the copyright owner(s) are credited and that the original publication in this journal is cited, in accordance with accepted academic practice. No use, distribution or reproduction is permitted which does not comply with these terms.
*Correspondence: Eric C. Westman, ewestman@duke.edu
Disclaimer: All claims expressed in this article are solely those of the authors and do not necessarily represent those of their affiliated organizations, or those of the publisher, the editors and the reviewers. Any product that may be evaluated in this article or claim that may be made by its manufacturer is not guaranteed or endorsed by the publisher.
Javascript is currently disabled in your browser. Several features of this site will not function whilst javascript is disabled.
- Why Publish With Us?
- Editorial Policies
- Author Guidelines
- Peer Review Guidelines
- Open Outlook
- Submit New Manuscript

- Sustainability
- Press Center
- Testimonials
- Favored Author Program
- Permissions
- Pre-Submission

open access to scientific and medical research
A part of Taylor & Francis Group
- Authors are invited to browse Collections that are currently open for submissions Read more
Latest Research on Diabetes
Diabetes, a chronic disease that occurs either when the pancreas does not produce enough insulin or when the body cannot effectively use the insulin it produces, is recognized by the World Health Organization as a major cause of mortality around the world.
At Dove Medical Press, we have curated the most recent diabetes research from our diverse range of journals. Thank you to our community of authors and peer reviewers who persistently contribute to the fight against this disease.
This content is free to access and updated daily.
- 469 records -
A Novel Inflammatory Marker: Relationship Between Red Cell Distribution Width/Albumin Ratio and Vascular Complications in Patients with Type 2 Diabetes Mellitus
Yu M, Pei L, Liu H, Wang J, Wen Y, Yang X, Ma C, Zhang X, Wu L, Wang L
Journal of Inflammation Research 2024 , 17:6265-6276
Published Date: 10 September 2024
Exploring the Biological Effects of Anti-Diabetic Vanadium Compounds in the Liver, Heart and Brain
Dayanand Y, Pather R, Xulu N, Booysen I, Sibiya N, Khathi A, Ngubane P
Diabetes, Metabolic Syndrome and Obesity 2024 , 17:3267-3278
Published Date: 4 September 2024
Causes of Excess Mortality in Diabetes Patients Without Coronary Artery Disease: A Cohort Study Revealing Endocrinologic Contributions
Birindwa G, Maeng M, Thrane PG, Gyldenkerne C, Thomsen RW, Olesen KKW
Clinical Epidemiology 2024 , 16:571-585
Published Date: 3 September 2024

Comparing the Self-Reported Acceptability of Discrete Choice Experiment and Best-Worst Scaling: An Empirical Study in Patients with Type 2 Diabetes Mellitus
Li F, Liu S, Gu Y, Li S, Tao Y, Wei Y, Chen Y
Patient Preference and Adherence 2024 , 18:1803-1813
Published Date: 30 August 2024
Evaluation of Vicarious Somatosensory Experience in Diabetes Mellitus: Bases for Empathy and Social Cognition
Amin SN, El-Akabawy G, Baker Saleh L, Sulaiman AS, Alsharif AA, Ahmed Qamoum M, Basheer Fahmawi M, Al-Matrouk A, Taha H, Ismail AA
Risk Management and Healthcare Policy 2024 , 17:1975-1986
Published Date: 15 August 2024
Correlation Between Growth Differentiation Factor-15 and Peripheral Neuropathy in Patients with Type 2 Diabetes Mellitus
Li Y, Wang Y, Cao Y, Zhang X, Dai W, Zhao Y, Zhang L, Han X
Diabetes, Metabolic Syndrome and Obesity 2024 , 17:3019-3028
Published Date: 14 August 2024
Lower Visceral Fat is Related to Diabetic Peripheral Neuropathy
Sun L, Zhang X, Yang J, Yuan J, Lei X
Diabetes, Metabolic Syndrome and Obesity 2024 , 17:2967-2974
Published Date: 8 August 2024
Integrated Transcriptomics and Proteomics Identified CMPK1 as a Potential Biomarker for Type 2 Diabetes Mellitus
Zhao K, Mao R, Yi W, Ren Z, Liu Y, Yang H, Wang S, Feng Z
Diabetes, Metabolic Syndrome and Obesity 2024 , 17:2923-2934
Published Date: 1 August 2024
Impact of Lipids on Insulin Resistance: Insights from Human and Animal Studies
Elkanawati RY, Sumiwi SA, Levita J
Drug Design, Development and Therapy 2024 , 18:3337-3360
Published Date: 31 July 2024
Patient Characteristics and Outcomes of Hospitalized Chronic Kidney Disease Patients with and without Type 2 Diabetes Mellitus: Observations from the German Claims Data-Based Cohort of the CaReMe-CKD Multinational Study

Leiner J, Pellissier V, König S, Stellmacher L, Hohenstein S, Schanner C, Kwast S, Kuhlen R, Bollmann A
Clinical Epidemiology 2024 , 16:487-500
Published Date: 22 July 2024

Exploring the Interplay of Diabetes, Deaf Patient Reported Outcomes, and Cancer Screening in Deaf and Hard of Hearing Women
Perrodin-Njoku E, Rao SR, Wang RM, Moreland CJ, Kushalnagar P
International Journal of Women's Health 2024 , 16:1235-1248
Published Date: 12 July 2024
Assessment of Cognitive Flexibility in Jordanian Diabetic Patients by Wisconsin Card Sorting and Trail Making Tests: Implications with Demographic, Anthropometric and Therapeutic Variables
Amin SN, El-Akabawy G, Abuqasem MA, AL-Rawashdeh AA, Ayyad MM, Ibrahim AK, AlShawagfeih AM, Ebdah SK, AlHajri RJ, Ismail AA
Diabetes, Metabolic Syndrome and Obesity 2024 , 17:2655-2670
Published Date: 1 July 2024
Importance of Assessing Sarcopenia in Patients with Type 2 Diabetes Mellitus Based on Body Fat Percentage Measured by Dual-Energy X-Ray Absorptiometry in Different Genders
Hou B, Wei X, Yang M, Cao Y, Dai W
Diabetes, Metabolic Syndrome and Obesity 2024 , 17:2571-2581
Published Date: 25 June 2024
New Diabetic Treatment by Alleviation of Autonomic Nervous System Dysfunction Measured as Periosteal Pressure Sensitivity at Sternum Improves Empowerment, Treatment Satisfaction, and Self-Reported Health of People with Type 2 Diabetes: A Randomized Trial
Hecquet SK, Ballegaard S, Eldrup E, Hansen CS, Hansen TW, Harboe GS, Rossing P, Pichat CSH, Watt T, Gyntelberg F, Ørsted N, Faber JO
Diabetes, Metabolic Syndrome and Obesity 2024 , 17:2519-2531
Published Date: 19 June 2024
Efficacy and Safety of DPP-4 Inhibitors and Metformin Combinations in Type 2 Diabetes: A Systematic Literature Review and Network Meta-Analysis
Chen R, Li J, Chen D, Wen W, Zhang S, Li J, Ruan Y, Zhang Z, Sun J, Chen H
Diabetes, Metabolic Syndrome and Obesity 2024 , 17:2471-2493
The Regulation of Metabolic Homeostasis by Incretins and the Metabolic Hormones Produced by Pancreatic Islets
Reed J, Bain SC, Kanamarlapudi V
Diabetes, Metabolic Syndrome and Obesity 2024 , 17:2419-2456
Published Date: 13 June 2024
A Novel Diabetic Arthritic Model in Rats Induced by Streptozotocin, High-Fat Diet, and Complete Freund’s Adjuvant
Boedijono DR, Dilogo IH, Prasetyo M, Antarianto RD, Dewi FNA, Yunir E, Kekalih A, Nugroho A, Aprilya D
Orthopedic Research and Reviews 2024 , 16:163-170
Published Date: 11 June 2024
Integrated Bioinformatics Analysis Revealed Immune Checkpoint Genes Relevant to Type 2 Diabetes
Zhang Z, Sun G, Wang Y, Wang N, Lu Y, Chen Y, Xia F
Diabetes, Metabolic Syndrome and Obesity 2024 , 17:2385-2401
Effects of Different Carbohydrate Content Diet on Gut Microbiota and Aortic Calcification in Diabetic Mice

Shen X, Guo G, Feng G, Wang Z
Diabetes, Metabolic Syndrome and Obesity 2024 , 17:2327-2346
Published Date: 10 June 2024
Gestational Diabetes Mellitus Risk Factors in Pregnant Women Attending Public Health Institutions in Ethiopia’s Sidama Region: An Unmatched Case-Control Study
Diabetes, Metabolic Syndrome and Obesity 2024 , 17:2303-2316
Published Date: 7 June 2024
Evaluation of MASLD Fibrosis, FIB-4 and APRI Score in MASLD Combined with T2DM and MACCEs Receiving SGLT2 Inhibitors Treatment
Liu H, Hao YM, Jiang S, Baihetiyaer M, Li C, Sang GY, Li Z, Du GL
International Journal of General Medicine 2024 , 17:2613-2625
Published Date: 5 June 2024
Plasma Asprosin Concentrations are Associated with Progression of Diabetic Kidney Disease
Xu M, Zhang C, Zhang L, Qu H, Wang Y
Diabetes, Metabolic Syndrome and Obesity 2024 , 17:2235-2242
Assessment of Health-Related Quality of Life Among Patients with Chronic Diseases and Its Relationship with Multimorbidity: A Cross-Sectional Study from Saudi Arabia
Alzarea AI, Khan YH, Alzarea SI, Alanazi AS, Alsaidan OA, Alrowily MJ, Al-Shammari M, Almalki ZS, Algarni MA, Mallhi TH
Patient Preference and Adherence 2024 , 18:1077-1094
Published Date: 4 June 2024
Current Perspectives, Practices, and Barriers Faced by Community Pharmacists Regarding Pharmaceutical Care Services for Diabetes Mellitus in the United Arab Emirates
Jairoun AA, Al-Hemyari SS, Shahwan M, Jairoun SA, Alorfi NM, Zyoud SH, Suliman AA, Shahwan MK, Alnuaimi G, Shahwan M, Al-Qirim T, El-Dahiyat F
Journal of Multidisciplinary Healthcare 2024 , 17:2563-2576
Published Date: 23 May 2024
The Prevalence of Sleep Disorders in People with Type 2 Diabetes and Obesity in Saudi Arabia: A Cross-Sectional Study
AL-asiri IS, Almatrafi FG, Al-thagafi SD, AlQarni AM, Aljubran HJ, Aljamaan AK, Al-Zahrani N
Diabetes, Metabolic Syndrome and Obesity 2024 , 17:2075-2083
Published Date: 20 May 2024
Machine Learning-Based Predictive Modeling of Diabetic Nephropathy in Type 2 Diabetes Using Integrated Biomarkers: A Single-Center Retrospective Study
Zhu Y, Zhang Y, Yang M, Tang N, Liu L, Wu J, Yang Y
Diabetes, Metabolic Syndrome and Obesity 2024 , 17:1987-1997
Published Date: 10 May 2024
Correlation Between Blood Urea Nitrogen and Short- and Long-Term Glycemic Variability in Elderly Patients with Type 2 Diabetes Mellitus Who Were hospitalized:A Retrospective Study
Huang L, Wang Z, Pan Y, Zhou K, Zhong S
Diabetes, Metabolic Syndrome and Obesity 2024 , 17:1973-1986
Published Date: 8 May 2024
Erectile Dysfunction and Depression Prevalence Among Male Patients with Type II Diabetes
Alswat KA, Almorgi Z, Aljardahi AN, Alobaylan RM, Altowairqi MK, Almorgi MW, Almalki DM, Alshehri KM
Journal of Multidisciplinary Healthcare 2024 , 17:2041-2051
Published Date: 3 May 2024
Causal Association Between Diabetes, Body Mass Index and Lichen Sclerosus: A Bidirectional Two-Sample Mendelian Randomization Analysis
Liu L, Zhang Q, Chang J, Yang K
Clinical, Cosmetic and Investigational Dermatology 2024 , 17:931-940
Published Date: 26 April 2024
Health Technology Assessment: Evaluation of 7 Glucagon-Like Peptide-1 Receptor Agonists for the Treatment of Type 2 Diabetes Mellitus
Xie Z, Hu J, Li M, Hu X, Chen J
Risk Management and Healthcare Policy 2024 , 17:1053-1067
Published Date: 23 April 2024
Correlation Between Serum Vitamin E and HOMA-IR in Patients with T2DM
Zhang J, Hou Y, Zhang Z, Shi Y, Wang Z, Song G
Diabetes, Metabolic Syndrome and Obesity 2024 , 17:1833-1843
Prevalence and Associated Factors of Sensorineural Hearing Impairment Among Patients with T2DM in Amhara Region, Northwest Ethiopia, 2022: A Multi-Centered Cross-Sectional Study
Esubalew D, Melese M, Belete M, Ambelu A, Mulat B, Adane T, Wondie WT, Diress M
Diabetes, Metabolic Syndrome and Obesity 2024 , 17:1821-1832
Published Date: 20 April 2024
Identification of a Novel Mitochondrial tRNA Mutation in Chinese Family with Type 2 Diabetes Mellitus
Li X, Shang J, Li S, Wang Y
Pharmacogenomics and Personalized Medicine 2024 , 17:149-161
Published Date: 17 April 2024
Serum Folate and Vitamin B12 Modify the Associations of N6AMT1 Genetic Variants with Gestational Diabetes Mellitus: A Cross-Sectional Study in Chinese Pregnant Women
Guo G, Chen X, Zhang J, Meng X, Jia A, Xing X, Huang F, Zhang X, Liu J, Li S, Zhang Q
Diabetes, Metabolic Syndrome and Obesity 2024 , 17:1781-1791

Latent Profile Analysis of Medication Beliefs in Patients with Type 2 Diabetes in the Hospital-Home Transition and Comparison with Medication Adherence
Jiang S, Luo T, Zhu Z, Huang Y, Liu H, Li B, Feng S, Zeng K
Patient Preference and Adherence 2024 , 18:839-853
Published Date: 16 April 2024
Fractional Excretion of Urate is Positively Associated with Type 2 Diabetes in HUA Patients: A Cross-Sectional Study
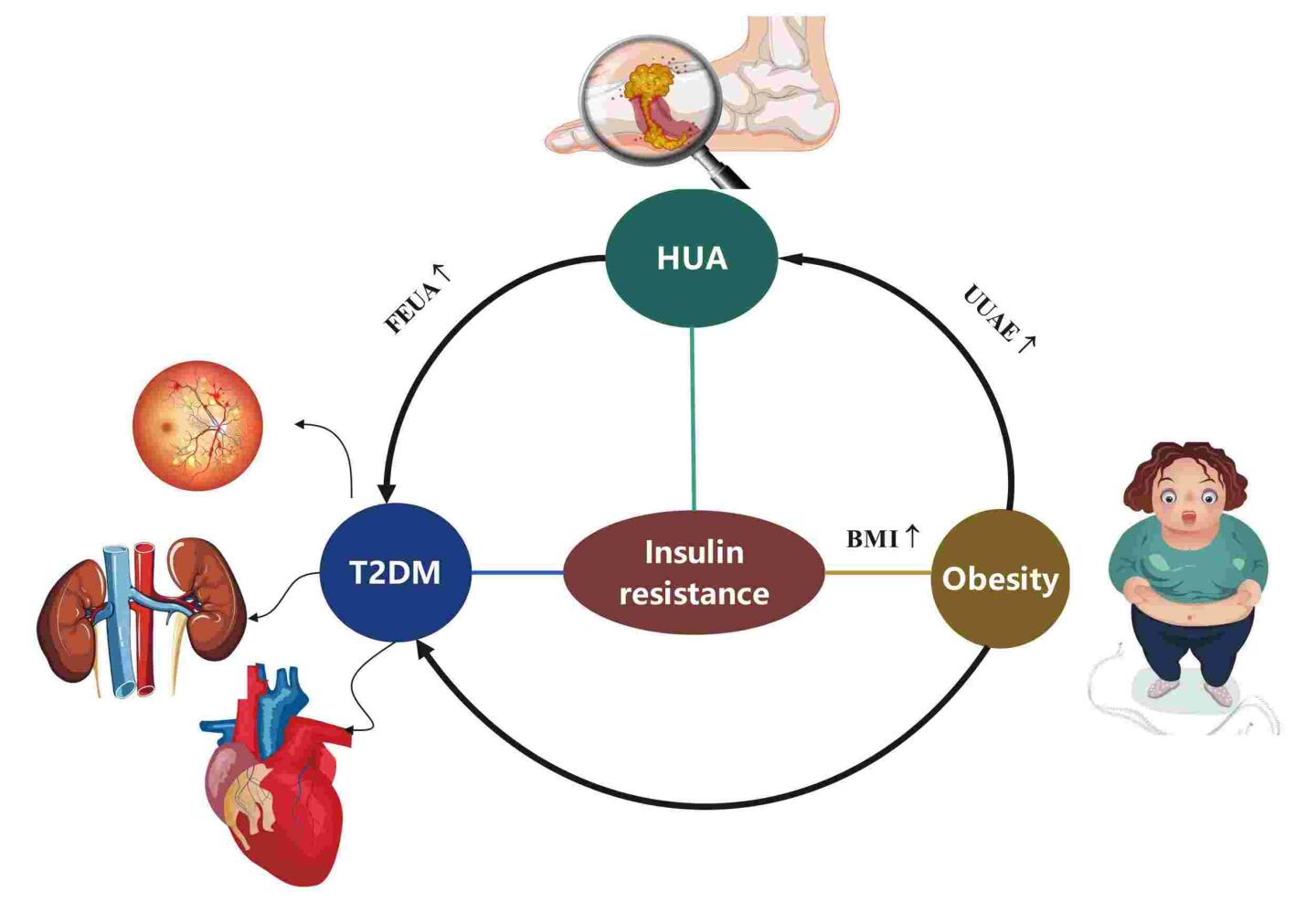
Huang B, Li X, Zhang X, Li S, Liu Y, Zhang M, Cui J
Diabetes, Metabolic Syndrome and Obesity 2024 , 17:1701-1713
Published Date: 13 April 2024
A Systematic Guideline by the ASPN Workgroup on the Evidence, Education, and Treatment Algorithm for Painful Diabetic Neuropathy: SWEET
Sayed D, Deer TR, Hagedorn JM, Sayed A, D'Souza RS, Lam CM, Khatri N, Hussaini Z, Pritzlaff SG, Abdullah NM, Tieppo Francio V, Falowski SM, Ibrahim YM, Malinowski MN, Budwany RR, Strand NH, Sochacki KM, Shah A, Dunn TM, Nasseri M, Lee DW, Kapural L, Bedder MD, Petersen EA, Amirdelfan K, Schatman ME, Grider JS
Journal of Pain Research 2024 , 17:1461-1501
Semaglutide Effects on Metabolic Outcomes in Diabetes Mellitus Patients — Real World Study
Balcázar-Valencia CM, García-Ramos AF, Osorio-Toro LM, Ordoñez-Guzmán YA, Buitrago-Gómez N, Cabarcas-López WF, Vizcaino-Guerrero CJ, Daza-Arana JE, Ramírez-Rincón A, Restrepo-Erazo K
Diabetes, Metabolic Syndrome and Obesity 2024 , 17:1667-1673
Published Date: 10 April 2024
Diversities of Mechanism in Patients with VHL Syndrome and diabetes: A Report of Two Cases and Literature Review
Wang Y, Liu Z, Zhao W, Cao C, Xiao L, Xiao J
Diabetes, Metabolic Syndrome and Obesity 2024 , 17:1611-1619
Published Date: 9 April 2024
Metabolic Syndrome and Tendon Disease: A Comprehensive Review
Lai C, Li R, Tang W, Liu J, Duan XD, Bao D, Liu H, Fu S
Diabetes, Metabolic Syndrome and Obesity 2024 , 17:1597-1609
First-Trimester Triglyceride-Glucose Index and Triglyceride/High-Density Lipoprotein Cholesterol are Predictors of Gestational Diabetes Mellitus Among the Four Surrogate Biomarkers of Insulin Resistance
Ma N, Bai L, Lu Q
Diabetes, Metabolic Syndrome and Obesity 2024 , 17:1575-1583
Published Date: 8 April 2024
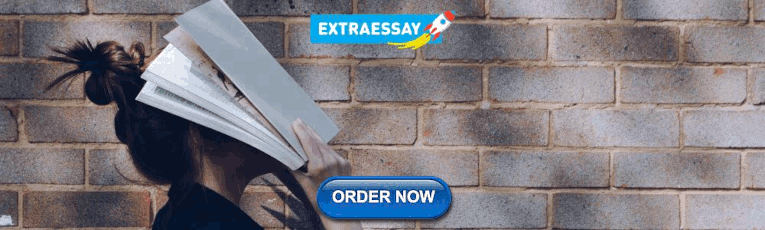
DR10627, a Novel Dual Glucagon‑like Peptide‑1 and Gastric Inhibitory Polypeptide Receptor Agonist for the Treatment of Obesity and Type 2 Diabetes Mellitus
Shao Y, Chen Y, Zhu M, Liu Y, Fang C, Wang M, Sun P, Fu W, Huang J, Sheng S, Huang Y
Diabetes, Metabolic Syndrome and Obesity 2024 , 17:1563-1573
Published Date: 6 April 2024
A Nomogram Including Total Cerebral Small Vessel Disease Burden Score for Predicting Mild Vascular Cognitive Impairment in Patients with Type 2 Diabetes Mellitus
Teng Z, Feng J, Xie X, Xu J, Jiang X, Lv P
Diabetes, Metabolic Syndrome and Obesity 2024 , 17:1553-1562
Age at Diagnosis of Diabetes in Young Men is Associated with Albuminuria
Zhang Q, Cheng L, Li B, Lu Y, Yang S, Wu Y
Diabetes, Metabolic Syndrome and Obesity 2024 , 17:1543-1549
Published Date: 4 April 2024
Cost-Savings Associated with Multi-Disciplinary Team Approach for Reducing Macrovascular and Microvascular Complications in Patients with Type 2 Diabetes: A Predictive Model
Alshowair A, Altamimi S, Alruhaimi FA, Alshahrani S, Alsuwailem F, Alkhaldi M, Abdalla H, Alkhuraiji FH, Alaqeel MS, Almureef SS, Alhawasy S, Abdel-Azeem A
ClinicoEconomics and Outcomes Research 2024 , 16:211-223
Butyrate and Propionate are Negatively Correlated with Obesity and Glucose Levels in Patients with Type 2 Diabetes and Obesity
Zhang S, Zhang Y, Li J, Wang X, Zhang M, Du M, Jiang W, Li C
Diabetes, Metabolic Syndrome and Obesity 2024 , 17:1533-1541
Published Date: 2 April 2024
Trajectories of Body Mass Index and Risk for Diabetes Complications and All-Cause Mortality in Finnish Type 2 Diabetes Patients
Wang Z, Lavikainen P, Wikström K, Laatikainen T
Clinical Epidemiology 2024 , 16:203-212
Published Date: 29 March 2024
Type 2 Diabetes, the Epidemic: Trends in Prevalence and Incidence, 2004-2020
Overbeek JA, Nijpels G, Swart KMA, Blom MT, Elders PJM, Herings RMC
Diabetes, Metabolic Syndrome and Obesity 2024 , 17:1503-1509
Published Date: 28 March 2024
Study on the Interaction Between C3 Gene Polymorphism and Environment in Patients with Type 2 Diabetes Combined with Coronary Artery Disease
Qiu H, Abudureyimu S, Liu M, Liu F, Gao Y
Diabetes, Metabolic Syndrome and Obesity 2024 , 17:1467-1479
Published Date: 27 March 2024
Correlation Between Thyroid-Related Hormones and Diabetic Retinopathy in Type 2 Diabetes Mellitus Patients with Normal Thyroid Function: A Retrospective Study
Xiao M, Luo G, Zhang Z, Liu Y, Gong R, Ke J
Diabetes, Metabolic Syndrome and Obesity 2024 , 17:1481-1490
Published Date: 26 March 2024
More articles
Contact Us • Privacy Policy • Associations & Partners • Testimonials • Terms & Conditions • Recommend this site • Cookies • Top
Contact Us • Privacy Policy
Become a Member
Diabetes Care®
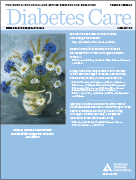
Diabetes Care is a journal for the health care practitioner that is intended to increase knowledge, stimulate research, and promote better management of people with diabetes. To achieve these goals, the journal publishes original articles on human studies in the following categories: clinical care, education, and nutrition; epidemiology, health services; and psychosocial research; emerging treatments and technologies; and pathophysiology and complications.
The journal also publishes ADA-issued recommendations and statements, clinically relevant review articles, editorials, and commentaries. Topics covered are of interest to clinically oriented physicians, researchers, epidemiologists, psychologists, diabetes educators, and other health professionals. Diabetes Care is the highest-ranked peer-reviewed journal in the field of diabetes treatment and prevention.
Read Diabetes Care .
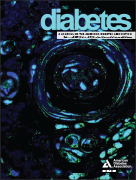
Diabetes publishes original research about the physiology and pathophysiology of diabetes. Emphasis is on investigative reports focusing on areas such as the pathogenesis of diabetes and its complications, normal and pathologic pancreatic islet function and intermediary metabolism, pharmacological mechanisms of drug and hormone action, and biochemical and molecular aspects of normal and abnormal biological processes. "Perspectives on Diabetes” provide readers with novel reviews and commentaries on diabetes-related research. Diabetes is the top-ranked journal devoted exclusively to diabetes research.
Read Diabetes .
Diabetes Spectrum®
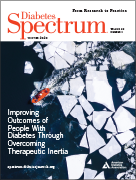
Diabetes Spectrum is committed to assisting health care professionals in developing strategies to individualize treatment, enhance diabetes self-management education, and optimize patient outcomes. It achieves these goals by presenting comprehensive peer-reviewed original research and review articles on a wide range of topics in diabetes medical management, care innovations, professional and patient education, medical nutrition therapy, behavioral science and counseling, pharmacy and therapeutics, and advocacy. The journal's "From Research to Practice” section provides in-depth explorations of selected diabetes care topics, with a primary focus on translating current research findings into practical clinical applications.
Read Diabetes Spectrum .
Clinical Diabetes®
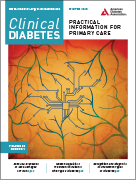
The mission of Clinical Diabetes is to provide primary care providers and all health professionals involved in the care of people with diabetes with information on advances and state-of-the-art care for people with diabetes. Clinical Diabetes is also a forum for discussing diabetes-related problems in practice, medical-legal issues, case studies, digests of recent research, and patient education materials. Each issue contains one or more feature articles that focus on the latest trends and innovations in diabetes care and treatment, as well as mini-reviews of landmark studies, practical treatment pointers, and best practices related to diabetes care.
Read Clinical Diabetes .
BMJ Open Diabetes Research & Care
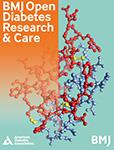
BMJ Open Diabetes Research & Care is published by BMJ in partnership with the American Diabetes Association. It is an open access journal committed to publishing high-quality basic and clinical research articles regarding type 1 and type 2 diabetes and associated complications. Submissions are subject to rigorous external peer review to ensure the publication of high-quality information. The online-only format allows for continuous updates and serves as an invaluable resource to the multidisciplinary community of endocrinology, public health/prevention, and internal medicine.
Read BMJ Open Diabetes Research & Care
Thank you for visiting nature.com. You are using a browser version with limited support for CSS. To obtain the best experience, we recommend you use a more up to date browser (or turn off compatibility mode in Internet Explorer). In the meantime, to ensure continued support, we are displaying the site without styles and JavaScript.
- View all journals
Type 2 diabetes articles from across Nature Portfolio
Type 2 diabetes mellitus, the most frequent subtype of diabetes, is a disease characterized by high levels of blood glucose (hyperglycaemia). It arises from a resistance to and relative deficiency of the pancreatic β-cell hormone insulin.
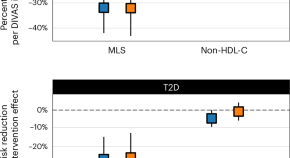
Blood lipid profiling indicates that dietary fat quality is associated with cardiometabolic risk
Dietary guidelines advise substituting saturated fats with unsaturated fats. We used detailed blood fat composition profiling in diet trials and population studies to confirm that a moderate high-fat diet from plant oils is better for metabolism and heart health than a diet with similar fat levels from animal sources.
New insights into the regulation of GIPR signalling
Two recent studies have unravelled novel modes of glucose-dependent insulinotropic polypeptide receptor (GIPR) signalling regulation. Kizilkaya et al. characterized the effect of changes in β-arrestin 2 coupling with naturally occurring GIPR coding variants, whereas Regmi et al. investigated GIPR expression profiles and functional regulation in adipocytes.
- Yusman Manchanda
- Alejandra Tomas
Latest Research and Reviews
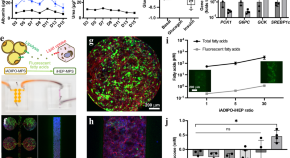
Adipocyte inflammation is the primary driver of hepatic insulin resistance in a human iPSC-based microphysiological system
In vitro modelling of the adipose tissue-liver axis can advance understanding and therapy of metabolic disease, including by distinguishing effects of obesity and inflammation. Here, authors develop such a system based on isogenic human iPSCs and interconnected microphysiological devices.
- Marko Groeger
- Andreas Stahl
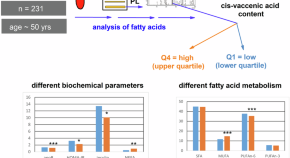
Associations of plasma phospholipid cis-vaccenic acid with insulin resistance markers in non-diabetic men with hyperlipidemia
- Jaroslav Macášek
- Barbora Staňková
- Miroslav Zeman
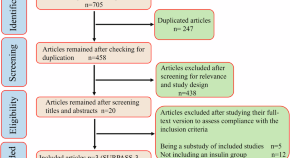
Tirzepatide outcompetes long-acting insulin in managing type 2 diabetes: a meta-analysis of three phase 3 randomized controlled trials
- Razieh Mohammad Jafari
- Mohammad Poursalehian
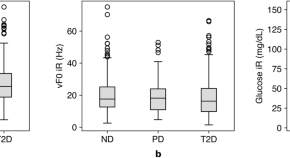
Linear effects of glucose levels on voice fundamental frequency in type 2 diabetes and individuals with normoglycemia
- Jaycee Kaufman
- Jouhyun Jeon
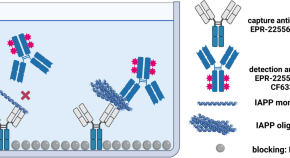
IAPP - oligomerisation levels in plasma of people with type 2 diabetes
- Fabian Rehn
- Victoria Kraemer-Schulien
- Dieter Willbold
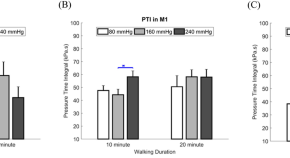
Effect of different inner pressures of air insoles and walking durations on plantar pressure time integral
- Gilang Titah Ramadhan
- Fahni Haris
- Chi-Wen Lung
News and Comment
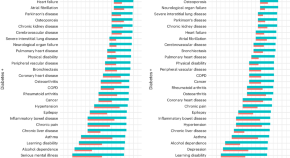
Years lived with and years lost to multiple long-term condition combinations that include diabetes
We introduced time-based metrics to analyze multiple long-term condition (MLTC) combinations of two or more conditions that include diabetes, among adults in England. We calculated the median age of MLTC onset and years of life lived with and lost to the MLTC, and examined MLTC burdens from both individual perspectives and community perspectives.
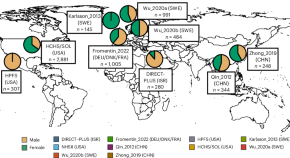
Linking gut microbial strains and functions to diabetes in an international population
Although gut microorganisms have been associated with type 2 diabetes, the mechanisms involving specific microbial strains remain unidentified. Now, a comprehensive study has analyzed 8,117 shotgun metagenomes from the USA, Europe, Israel and China, providing cross-population microbial signatures in a strain-resolved manner and new mechanistic insights into type 2 diabetes.
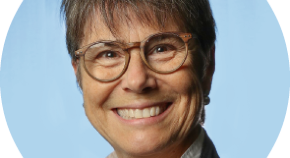
How medical schools can prepare students for new technologies
Patient educators and nurses can demonstrate the real-life use of health technologies.
- Chantal Mathieu
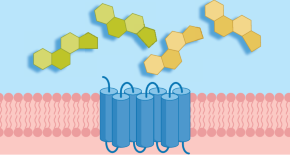
Bile acid receptor protects against diabetic cardiomyopathy
Activation of the bile acid receptor TGR5 inhibits CD36-mediated fatty acid uptake in cardiomyocytes and protects against cardiac lipotoxicity and the development of diabetic cardiomyopathy in mice, according to a new study.
- Irene Fernández-Ruiz
Quick links
- Explore articles by subject
- Guide to authors
- Editorial policies

Search Icon
Events See all →
Incantation.

6:00 p.m. - 7:30 p.m.
Penn Museum, 3260 South St.
Movable Books Opening

4:00 p.m. - 8:00 p.m.
Van Pelt-Dietrich Library, 3420 Walnut St.
September 2024 Wellness Walk

LOVE Sculpture on Locust Walk
Health Sciences
Understanding diabetes and oral health
Two undergraduates, supported by purm, worked on research projects this summer with the graves lab to contribute to the knowledge of diabetes’ impact on oral wound healing and periodontal disease..
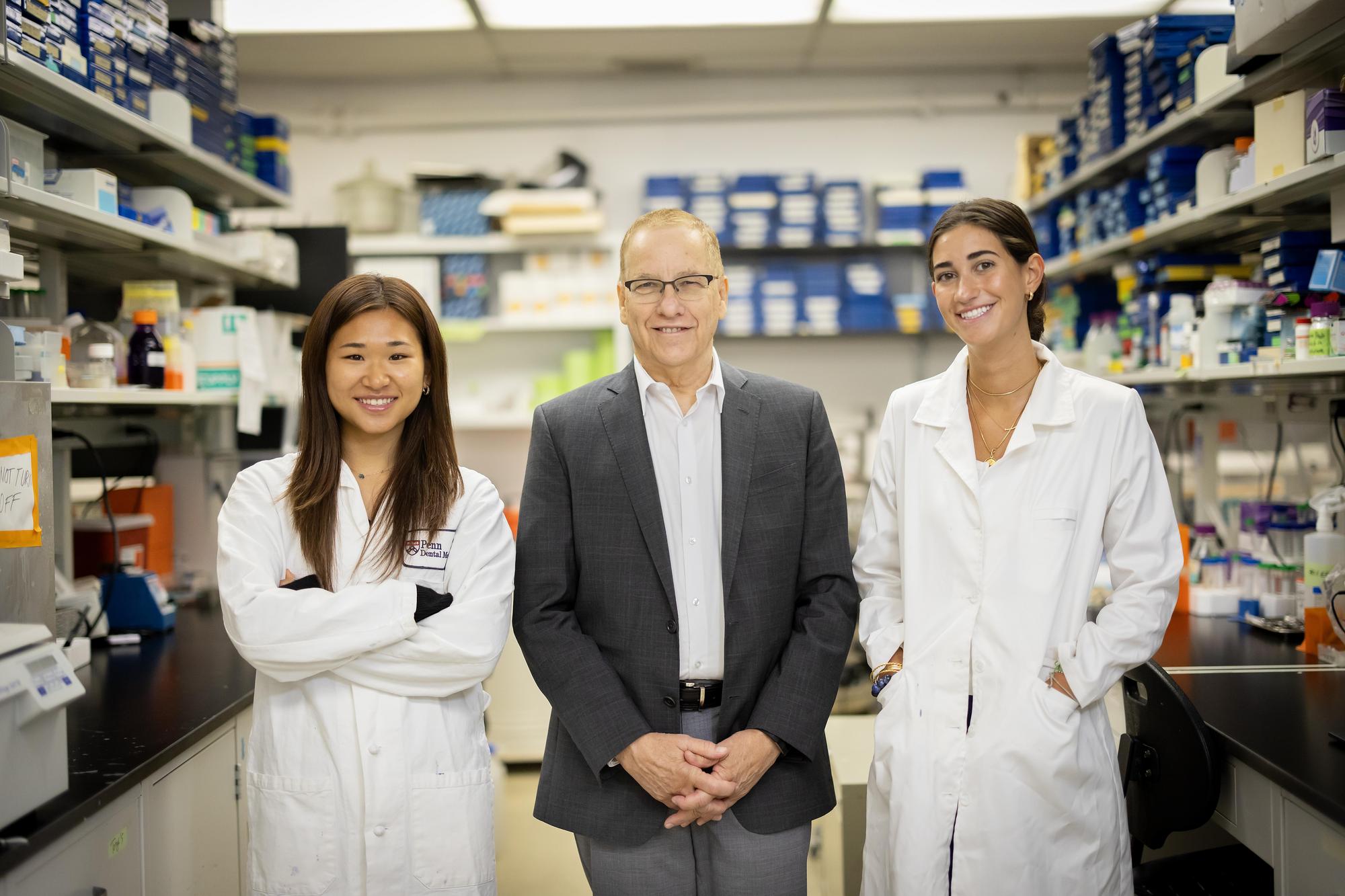
Despite a robust amount of research about diabetes, much is still unknown about its effects on oral health. Changes in inflammation are understood to contribute to the impact of diabetes, but what is driving the increase is less clear.
Through a study in the Graves Lab, led by Dana Graves , a professor of periodontics in the School of Dental Medicine , the goal has been to identify molecules that are modulated by diabetes to increase pathology.
One project, co-led by research scholar Hamideh Afzali, used single-cell RNA sequencing, which is a sophisticated assay that measures the mRNA level of thousands of genes in each individual cell. The sequencing focused on white blood cells in contrast to many wound healing studies that focus on fibroblasts. That led the research team to identify the gene S100A11 that was found at high levels in diabetic wounds in a specific white blood cell, neutrophils.
“Not as many genes were obviously affected by diabetes as we expected," explains Graves. “There’s not a huge number that are different. … And this gene is the one that Hamideh thought was pretty important.”
The process was to examine the healing of diabetic wounds in mice and conduct bioinformatic analysis during the healing process when connective tissue starts to form. The analysis led to S100A11, but the question remained whether inhibiting this gene would improve healing.
Helpful in this process was Sanan Gueyikian, a third-year neuroscience major with a minor in health care management in the College of Arts and Sciences , who measured histologic tissues to assess, quantitatively, whether healing improved. Gueyikian conducted this work through the Penn Undergraduate Research Mentoring Program (PURM), a 10-week opportunity from the Center for Undergraduate Research and Fellowships . The program provides rising second- and third-year students with a $5,000 award to work alongside Penn faculty.
“There’s a long history of diabetes in my family, and the lack of information regarding its cause or what it can trigger fascinates me,” Gueyikian says. “I am also interested in dental medicine and pursuing it in the future. So, Dr. Graves’ lab was the perfect place for me to explore these interests.”
Through the experience, she says, she learned a lot about signaling pathways and testing for genes. Gueyikian adds that, with the mentorship of Afzali, she learned to read research papers differently and understand them better. She says that this was her first experience with research and further cemented her interest in oral surgery.
“I came out with a much better understanding of the research process,” she says.
A second research project in the Graves Lab, supported with a PURM student researcher, looked at periodontal disease and bone loss among diabetics. Su Ah Kim, a third-year student majoring in finance and healthcare management at the Wharton School with a minor in chemistry, worked with senior research investigator Min Liu to genotype and quantify bone loss in diabetic mice. Liu says Kim is “very smart and works very hard and learns very fast,” and analyzed the bone levels to help determine whether the Akt1 gene played a significant role in the increased periodontal disease caused by diabetes.
Like Gueyikian, Kim says her family has a history of diabetes, which partly spurred her interest in the PURM project. She plans to pursue dentistry after graduating and has spent time shadowing at University Dental Associates. She hopes to combine her business and dentistry knowledge to one day own her own practice.
The research opportunity, Kim says, taught her how to be more self-sufficient, while also giving her a chance to interact with Penn Dental students who were happy to answer questions about dentistry as a career path.
“I learned in the 10 weeks that self-initiation and being proactive are fundamental for success in scientific research,” Kim says.
“It was my first time conducting research in a wet lab, so I experienced learning curves with performing procedures, including genotyping and histological staining,” she adds. “However, through several processes of trial and error and collaboration with other lab members, I developed a strong knowledge of each procedure, which I was able to carry out successfully on my own.” Graves is a big fan of the PURM program and has brought undergraduates into projects for many years, he says—always to great success.
“Both of them contributed a lot to their projects,” says Graves. “This was not a teaching exercise; this was an opportunity to participate as a researcher. They both will be coauthors on a paper and both provided valuable data, bringing a lot of enthusiasm, while learning quickly. They were a pleasure to work with.”
Participating PURM students, Sanan and Kim, he says, learned how important teamwork is in a research lab setting and how it is necessary to obtain successful results. He says both students embraced a spirit of cooperation, which is “a key factor” in lab work.
“They became real researchers toward the end, both understanding their projects well and capable of carrying out the experimental assays,” he says.
At Convocation, a call to ‘come together’
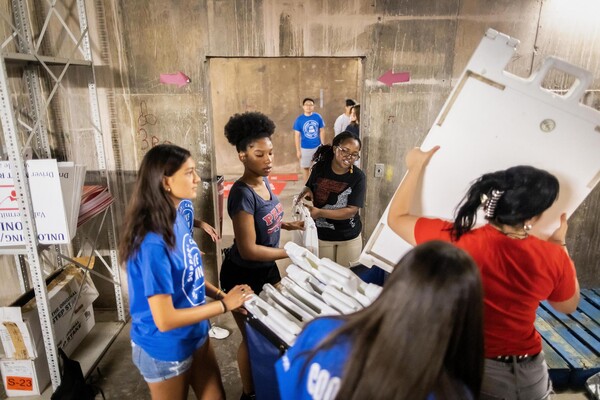
Campus & Community
Move-In coordinators help ease transition to college
Forty-eight second-year, third-year, and fourth-year students will be on the ground during Move-In to assist approximately 6,000 new and returning Quakers.
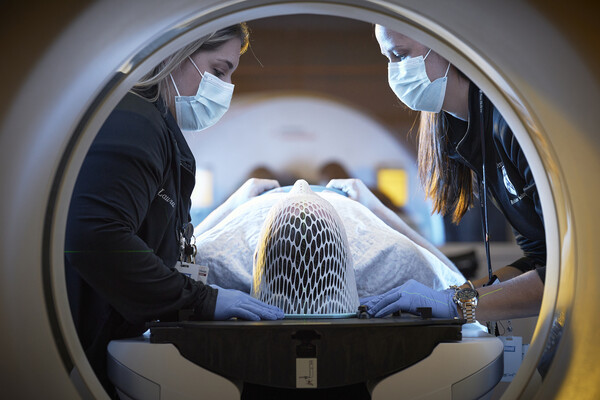
The power of protons
Penn Medicine has treated more than 10,000 cancer patients at three proton therapy centers across the region, including the largest and busiest center in the world—while also leading the way in research to expand the healing potential of these positive particles.
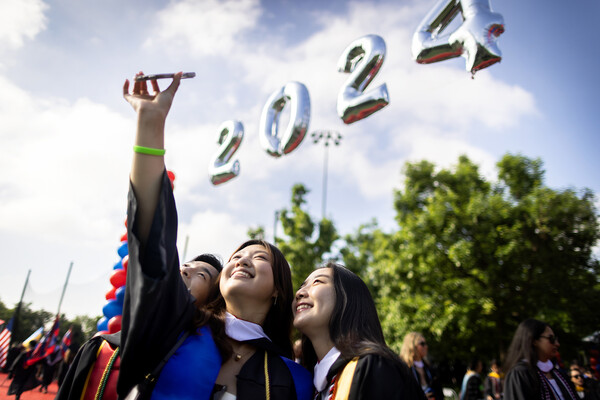
To Penn’s Class of 2024: ‘The world needs you’
The University celebrated graduating students on Monday during the 268th Commencement.
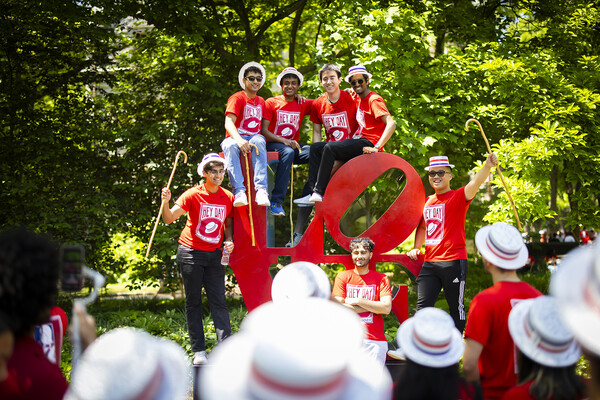
Class of 2025 relishes time together at Hey Day
An iconic tradition at Penn, third-year students were promoted to senior status.

An official website of the United States government
The .gov means it’s official. Federal government websites often end in .gov or .mil. Before sharing sensitive information, make sure you’re on a federal government site.
The site is secure. The https:// ensures that you are connecting to the official website and that any information you provide is encrypted and transmitted securely.
- Publications
- Account settings
Preview improvements coming to the PMC website in October 2024. Learn More or Try it out now .
- Advanced Search
- Journal List
- J Healthc Eng
- v.2021; 2021

Machine Learning Based Diabetes Classification and Prediction for Healthcare Applications
Umair muneer butt.
1 School of Computer Sciences, Universiti Sains Malaysia, Penang, Malaysia
Sukumar Letchmunan
Mubashir ali.
2 Department of Management,Information and Production Engineering, University of Bergamo, Bergamo, Italy
Fadratul Hafinaz Hassan
Anees baqir.
3 Department of Environmental Sciences,Informatics,and Statistics, Ca'Foscari University of Venice, Venice, Italy
Hafiz Husnain Raza Sherazi
4 School of Computing and Engineering, University of West London, London, UK
Associated Data
The data used to support the findings of this study are included within the article.
The remarkable advancements in biotechnology and public healthcare infrastructures have led to a momentous production of critical and sensitive healthcare data. By applying intelligent data analysis techniques, many interesting patterns are identified for the early and onset detection and prevention of several fatal diseases. Diabetes mellitus is an extremely life-threatening disease because it contributes to other lethal diseases, i.e., heart, kidney, and nerve damage. In this paper, a machine learning based approach has been proposed for the classification, early-stage identification, and prediction of diabetes. Furthermore, it also presents an IoT-based hypothetical diabetes monitoring system for a healthy and affected person to monitor his blood glucose (BG) level. For diabetes classification, three different classifiers have been employed, i.e., random forest (RF), multilayer perceptron (MLP), and logistic regression (LR). For predictive analysis, we have employed long short-term memory (LSTM), moving averages (MA), and linear regression (LR). For experimental evaluation, a benchmark PIMA Indian Diabetes dataset is used. During the analysis, it is observed that MLP outperforms other classifiers with 86.08% of accuracy and LSTM improves the significant prediction with 87.26% accuracy of diabetes. Moreover, a comparative analysis of the proposed approach is also performed with existing state-of-the-art techniques, demonstrating the adaptability of the proposed approach in many public healthcare applications.
1. Introduction
Public health is a fundamental concern for protecting and preventing the community from health hazard diseases [ 1 ]. Governments are spending a considerable amount of their gross domestic product (GDP) for the welfare of the public, and initiatives such as vaccination have prolonged the life expectancy of people [ 2 ]. However, for the last many years, there has been a considerable emergence of chronic and genetic diseases affecting public health. Diabetes mellitus is one of the extremely life-threatening diseases because it contributes to other lethal diseases, i.e., heart, kidney, and nerve damage [ 3 ].
Diabetes is a metabolic disorder that impairs an individual's body to process blood glucose, known as blood sugar. This disease is characterized by hyperglycemia resulting from defects in insulin secretion, insulin action, or both [ 3 ]. An absolute deficiency of insulin secretion causes type 1 diabetes (T1D). Diabetes drastically spreads due to the patient's inability to use the produced insulin. It is called type 2 diabetes (T2D) [ 4 ]. Both types are increasing rapidly, but the ratio of increase in T2D is higher than T1D. 90 to 95% of cases of diabetes are of T2D.
Inadequate supervision of diabetes causes stroke, hypertension, and cardiovascular diseases [ 5 ]. To avoid and reduce the complications due to diabetes, a monitoring method of BG level plays a prominent role [ 6 ]. A combination of biosensors and advanced information and communication technology (ICT) provides an efficient real-time monitoring management system for the health condition of diabetic patients by using SMBG (self-monitoring of blood glucose) portable device. A patient can check the changes in glucose level in his blood by himself [ 7 ]. Users can better understand BG changes by using CGM (continuous glucose monitoring) sensors [ 4 ].
By exploiting the advantages of the advancement in modern sensor technology, IoT, and machine learning techniques, we have proposed an approach for the classification, early-stage identification, and prediction of diabetes in this paper. The primary objective of this study is twofold. First, to classify diabetes into predefined categories, we have employed three widely used classifiers, i.e., random forest, multilayer perceptron, and logistic regression. Second, for the predictive analysis of diabetes, long short-term memory (LSTM), moving averages (MA), and linear regression (LR) are used. To demonstrate the effectiveness of the proposed approach, PIMA Indian Diabetes is used for experimental evaluation. We concluded that, in experimental evaluation, MLP achieved an accuracy of 86.083% in diabetes classification as compared to the other classifiers and LSTM achieved a prediction accuracy of 87.26% for the prediction of diabetes. Moreover, we have also performed a comparative analysis of the proposed approach with existing state-of-the-art approaches. The accuracy results of our proposed approach demonstrate its adaptability in many healthcare applications.
Besides, we have also presented the IoT-based hypothetical diabetes self-monitoring system that uses BLE (Bluetooth Low Energy) devices and data processing in real-time. The latter technique used two applications: Apache Kafka (for streaming messages and data) and MongoDB (to store data). By utilizing BLE-based sensors, one can collect essential sign data about weight and blood glucose. These data will be handled by data processing techniques in a real-time environment. A BLE device will receive all the data produced by sensors and other necessary information about the patient that resides in the user application, installed on the cell phone. The raw data produced by sensors will be processed using the proposed approach to produce results, suggestions, and treatment from the patient's server-side.
The rest of the paper is organized as follows. In Section 2 , the paper presents the motivations for the proposed system by reviewing state-of-the-art techniques and their shortcomings. It covers the literature review about classification, prediction, and IoT-based techniques for healthcare. Section 3 highlights the role of physical activity in diabetes prevention and control. In Section 4 , we proposed the design and architecture of the diabetes classification and prediction systems. Section 5 discusses the results and performance of the proposed approach with state-of-the-art techniques. In Section 6 , an IoT-based hypothetical system is presented for real-time monitoring of diabetes. Finally, the paper is concluded in Section 7 , outlining the future research directions.
2. Literature Review
In this section, we discussed the classification and prediction algorithms for diabetes prediction in healthcare. Particularly, the significance of BLE-based sensors and machine learning algorithms is highlighted for self-monitoring of diabetes mellitus in healthcare. Machine learning plays an essential part in the healthcare industry by providing ease to healthcare professionals to analyze and diagnose medical data [ 8 – 12 ]. Moreover, intelligent healthcare systems are providing real-time clinical care to needy patients [ 13 , 14 ]. The features covered in this study are compared with the state-of-the-art studies ( Table 1 ).
Features' comparison of the proposed study vs. state-of-the-art studies.
Study | Diabetes classification | Diabetes prediction | Real-time healthcare data analysis | Performance measures |
---|---|---|---|---|
[ ] | ✔ | ✖ | ✖ | Accuracy |
[ ] | ✔ | ✖ | ✖ | NA |
[ ] | ✔ | ✖ | ✖ | Accuracy |
[ ] | ✔ | ✖ | ✖ | NA |
[ ] | ✔ | ✔ | ✖ | Accuracy |
[ ] | ✔ | ✖ | ✖ | Accuracy |
[ ] | ✔ | ✖ | ✖ | NA |
[ ] | ✖ | ✔ | ✖ | Accuracy |
[ ] | ✔ | ✔ | ✖ | Accuracy |
[ ] | ✖ | ✔ | ✖ | Accuracy |
[ ] | ✖ | ✔ | ✖ | Accuracy |
[ ] | ✖ | ✔ | ✖ | Accuracy, correlation coefficient |
[ ] | ✖ | ✖ | ✔ | NA |
[ – ] | ✖ | ✖ | ✔ | NA |
[ ] | ✔ | ✖ | ✔ | Accuracy |
[ ] | ✔ | ✖ | ✔ | Accuracy, standard deviation |
Proposed | ✔ | ✔ | ✔ | Accuracy, Precision, Recall, RMSE, |
2.1. Diabetes Classification for Healthcare
Health condition diagnosis is an essential and critical aspect for healthcare professionals. Classification of a diabetes type is one of the most complex phenomena for healthcare professionals and comprises several tests. However, analyzing multiple factors at the time of diagnosis can sometimes lead to inaccurate results. Therefore, interpretation and classification of diabetes are a very challenging task. Recent technological advances, especially machine learning techniques, are incredibly beneficial for the healthcare industry. Numerous techniques have been presented in the literature for diabetes classification.
Qawqzeh et al. [ 15 ] proposed a logistic regression model based on photoplethysmogram analysis for diabetes classification. They used 459 patients' data for training and 128 data points to test and validate the model. Their proposed system correctly classified 552 persons as nondiabetic and achieved an accuracy of 92%. However, the proposed technique is not compared with state-of-the-art techniques. Pethunachiyar [ 16 ] presented a diabetes mellitus classification system using a machine learning algorithm. Mainly, he used a support vector machine with different kernel functions and diabetes data from the UCI Machine Repository. He found SVM with linear function more efficient than naïve Bayes, decision tree, and neural networks. Nevertheless, the state-of-the-art comparison is missing and parameter selection is not elaborated.
Gupta et al. [ 17 ] exploited naïve Bayes and support vector machine algorithms for diabetes classification. They used the PIMA Indian Diabetes dataset. Besides, they used a feature selection based approach and k-fold cross-validation to improve the accuracy of the model. The experimental results showed the supremacy of the support vector machine over the naïve Bayes model. However, state-of-the-art comparison is missing along with achieved accuracy. Choubey et al. [ 18 ] presented a comparative analysis of classification techniques for diabetes classification. They used PIMA Indian data collected from the UCI Machine Learning Repository and a local diabetes dataset. They used AdaBoost, K-nearest neighbor regression, and radial basis function to classify patients as diabetic or not from both datasets. Besides, they used PCA and LDA for feature engineering, and it is concluded that both are useful with classification algorithms for improving accuracy and removing unwanted features.
Maniruzzaman et al. [ 19 ] used a machine learning paradigm to classify and predict diabetes. They utilized four machine learning algorithms, i.e., naive Bayes, decision tree, AdaBoost, and random forest, for diabetes classification. Also, they used three different partition protocols along with the 20 trials for better results. They used US-based National Health and Nutrition Survey data of diabetic and nondiabetic individuals and achieved promising results with the proposed technique. Ahuja et al. [ 20 ] performed a comparative analysis of various machine learning approaches, i.e., NB, DT, and MLP, on the PIMA dataset for diabetic classification. They found MLP superior as compared to other classifiers. The authors suggested that the performance of MLP can be enhanced by fine-tuning and efficient feature engineering. Recently, Mohapatra et al. [ 21 ] have also used MLP to classify diabetes and achieved an accuracy of 77.5% on the PIMA dataset but failed to perform state-of-the-art comparisons. MLP has been used in the literature for various healthcare disease classifications such as cardiovascular and cancer classification [ 35 , 36 ].
2.2. Predictive Analysis of Diabetes for Healthcare
Accurate classification of diabetes is a fundamental step towards diabetes prevention and control in healthcare. However, early and onset identification of diabetes is much more beneficial in controlling diabetes. The diabetes identification process seems tedious at an early stage because a patient has to visit a physician regularly. The advancement in machine learning approaches has solved this critical and essential problem in healthcare by predicting disease. Several techniques have been proposed in the literature for diabetes prediction.
Singh and Singh [ 22 ] proposed a stacking-based ensemble method for predicting type 2 diabetes mellitus. They used a publicly available PIMA dataset from the UCI Machine Learning Repository. The stacking ensemble used four base learners, i.e., SVM, decision tree, RBF SVM, and poly SVM, and trained them with the bootstrap method through cross-validation. However, variable selection is not explicitly mentioned and state-of-the-art comparison is missing.
Kumari et al. [ 23 ] presented a soft computing-based diabetes prediction system that uses three widely used supervised machine learning algorithms in an ensemble manner. They used PIMA and breast cancer datasets for evaluation purposes. They used random forest, logistic regression, and naïve Bayes and compared their performance with state-of-the-art individual and ensemble approaches, and their system outperforms with 79% accuracy.
Islam et al. [ 24 ] utilized data mining techniques, i.e., random forest, logistic regression, and naïve Bayes algorithm, to predict diabetes at the early or onset stage. They used 10-fold cross-validation and percentage split techniques for training purposes. They collected diabetic and nondiabetic data from 529 individuals directly from a hospital in Bangladesh through questionnaires. The experimental results show that random forest outperforms as compared to other algorithms. However, the state-of-the-art comparison is missing and achieved accuracy is not reported explicitly.
Malik et al. [ 25 ] performed a comparative analysis of data mining and machine learning techniques in early and onset diabetes mellitus prediction in women. They exploited traditional machine learning algorithms for proposing a diabetes prediction framework. The proposed system is evaluated on a diabetes dataset of a hospital in Germany. The empirical results show the superiority of K-nearest neighbor, random forest, and decision tree compared to other traditional algorithms.
Hussain and Naaz [ 26 ] presented a thorough review of machine learning models presented during 2010–2019 for diabetes prediction. They compared traditional supervised machine learning models with neural network-based algorithms in terms of accuracy and efficiency. They used Matthews correlation coefficient for evaluation purposes and observed naïve Bayes and random forest's supremacy compared to other algorithms.
2.3. Real-Time IoT-Based Processing of Healthcare Data
Real-time diabetes prediction is a complicated task. The emerging use of sensors in healthcare paved the path to handle fatal diseases [ 37 ]. Several techniques have been presented in the literature to classify and predict diabetes. Acciaroli et al. [ 4 ] exposed two accurate meters to measure diabetes in blood with less error rate. Furthermore, these commercial versions of glucometers are Accu-Chek with 6.5% error and CareSens with 4.0% error. Buckingham et al. [ 38 ] described the accuracy link of CGM with the calibration sensor. Alfian et al. [ 27 ] uncovered that the FDA had accepted CGM sensors for monitoring glucose in different trends and patterns. Moreover, at one particular time, one glucose reading should not be used to analyze the amount of insulin as not accepted in a glucometer. Rodríguez et al. [ 28 ] proposed a structural design containing a local gateway as a smartphone, cloud system, and sensors for advanced management of diabetes.
Filippoupolitis et al. [ 29 ] planned action to acknowledge a system using Bluetooth Low Energy (BLE) beacons and smartwatches. Mokhtari et al. considered technologies working with BLE for activity labeling and resident localization [ 30 ]. Gentili et al. [ 31 ] have used BLE with another application called Blue Voice, which can reveal the probability of multimedia communication of sensor devices and speech streaming service. Suárez et al. [ 32 ] projected a monitoring system based on the BLE device for air quality exposure with the environmental application. It aims at defining potential policy responses and studies the variables that are interrelated between societal level factors and diabetes prevalence [ 33 , 34 ].
Wang et al. [ 39 ] have given a general idea of the up-to-date BLE technology for healthcare systems based on a wearable sensor. They suggested that low-powered communication sensor technologies such as a BLE device can make it feasible for wearable systems of healthcare because it can be used without location constraints and is light in weight. Moreover, BLE is the first wireless technology in communication for healthcare devices in the form of a wearable device that meets expected operating requirements with low power, communication with cellular directly, secure data transmission, interoperability, electronic compatibility, and Internet communications. Rachim and Chung [ 40 ] have suggested one transmission system that used deficient power to observe the heart's activity through electrocardiograph signals using a BLE device for data transmission collecting by armband sensors and smartphones.
Mora et al. projected a dispersed structure using the IoT model to check human biomedically generated signals in reports using a BLE sensor device [ 41 ]. Cappon et al. [ 42 ] explored the study of CGM wearable sensors' prototypes and features of the commercial version currently used. Årsand et al. [ 43 ] offered the easiest method for monitoring blood glucose, physical activity, insulin injections, and nutritional information using smartphones and smartwatches. Morón et al. [ 44 ] observed the performance of the smartphone used in the medical field. Lee and Yoo [ 45 ] anticipated a structure using PDA (personal digital assistant) to manage diabetic patient's conditions better. It can also be used to send information about blood pressure, BG level, food consumption, and exercise plan of a patient with diabetes and give the direction of treatment by monitoring physical activity, food consumption, and insulin prescribed amount.
Rodríguez et al. [ 28 ] suggested an application for the smartphone, which can be used to receive the data from the sensor using a glucometer automatically. Rodríguez-Rodríguez et al. [ 46 ] suggested that checking the patient's glucose level and heart rate using sensors will produce colossal data, and analysis on big data can be used to solve this problem.
3. Role of Physical Activity in Prevention and Control of Diabetes Mellitus
Generally, physical activity is the first prevention and control strategy suggested by healthcare professionals to diabetic or prediabetic patients [ 47 ]. Among diet and medicine, exercise is a fundamental component in diabetes, cardiovascular disease, obesity, and lifestyle rescue programs. Nonetheless, dealing with all the fatal diseases has a significant economic burden. However, diabetes mellitus emerged as a devastating problem for the health sector and economy of a country of this century.
Recently, the international diabetes prevention and control federation predicts that diabetes can affect more than 366 million people worldwide [ 49 ]. The disease control and prevention center in the US alarmed the government that diabetes can affect more than 29 million people [ 50 ]. While these alarming numbers are continuously increasing, they will burden the economy around the globe. Therefore, researchers and healthcare professionals worldwide are researching and proposing guidelines to prevent and control this life-threatening disease. Sato [ 51 ] presented a thorough survey on the importance of exercise prescription for diabetes patients in Japan. He suggested that prolonged sitting should be avoided and physical activity should be performed every 30 minutes. Kirwan et al. [ 47 ] emphasized regular exercise to control and prevent type 2 diabetes. Particularly, they studied the metabolic effect on tissues of diabetic patients and found very significant improvements in individuals performing regular exercise. Moser et al. [ 48 ] have also highlighted the significance of regular exercise in improving the functionality of various organs of the body, as shown in Figure 1 .

Impact of regular exercise on metabolism of diabetic patients [ 48 ].
Yang et al. [ 52 ] focused on exercise therapy which plays a significant role in treating diabetes and its associated side effects. Specifically, they discovered cytokines which gives a novel insight into diabetes control, but the sequence is still under study. Kim and Jeon [ 53 ] presented a systematic overview of the effect of different exercises on the metabolism improvement of diabetic young individuals. They pointed out that several studies reported the significance of exercise on insulin, BP, and BG level improvement. However, none of these studies mentions the beta-cell improvement. Therefore, many challenges persist in diabetes prevention and control, which need serious attention from researchers worldwide.
4. Proposed Diabetic Classification and Prediction System for Healthcare
The proposed diabetes classification and prediction system has exploited different machine learning algorithms. First, to classify diabetes, we utilized logistic regression, random forest, and MLP. Notably, we fine-tuned MLP for classification due to its promising performance in healthcare, specifically in diabetes prediction [ 20 , 21 , 35 , 36 ]. The proposed MLP architecture and algorithm are shown in Figure 2 and Algorithm 1 , respectively.

Proposed MLP architecture with eight variables as input for diabetes classification.

Diabetes classification algorithm using MLP for healthcare.
Second, we implement three widely used machine learning algorithms for diabetes prediction, i.e., moving averages, linear regression, and LSTM. Mainly, we optimized LSTM for crime prediction due to its outstanding performance in real-world applications, particularly in healthcare [ 53 ]. The implementation details of the proposed algorithms are as follows.
4.1. Diabetes Classification Techniques
For diabetic classification, we fine-tuned three widely used state-of-the-art techniques. Mainly, a comparative analysis is performed among the proposed techniques for classifying an individual in either of the diabetes categories. The details of the proposed diabetes techniques are as follows.
4.1.1. Logistic Regression
It is appropriate to use logistic regression when the dependent variable is binary [ 54 ], as we have to classify an individual in either type 1 or type 2 diabetes. Besides, it is used for predictive analysis and explains the relationship between a dependent variable and one or many independent variables, as shown in equation ( 1 ). Therefore, we used the sigmoid cost function as a hypothesis function ( h θ ( x )). The aim is to minimize cost function J ( θ ). It always results in classifying an example either in class 1 or class 2.
4.1.2. Random Forest (RF)
As its name implies, it is a collection of models that operate as an ensemble. The critical idea behind RF is the wisdom of the crowd, each model predicts a result, and in the end, the majority wins. It has been used in the literature for diabetic prediction and was found to be effective [ 55 ]. Given a set of training examples X = x 1 , x 2 ,…, x m and their respective targets Y = y 1 , y 2 ,…, y m , RF classifier iterates B times by choosing samples with replacement by fitting a tree to the training examples. The training algorithm consists of the following steps depicted in equation ( 2 ).
- For b = 1... B , sample with replacement n training examples from X and Y .
- Train a classification tree f b on X b and Y b .
4.1.3. Multilayer Perceptron
For diabetes classification, we have fine-tuned multilayer perceptron in our experimental setup. It is a network where multiple layers are joined together to make a classification method, as shown in Figure 2 . The building block of this model is perceptron, which is a linear combination of input and weights. We used a sigmoid unit as an activation function shown in Algorithm 1 . The proposed algorithm consists of three main steps. First, weights are initialized and output is computed at the output layer ( δ k ) using the sigmoid activation function. Second, the error is computed at hidden layers ( δ h ) for all hidden units. Finally, in a backward manner, all network weights ( w i , j ) are updated to reduce the network error. The detailed procedure is outlined in Algorithm 1 for diabetes classification.
Figure 2 shows the multilayer perceptron classification model architecture where eight neurons are used in the input layer because we have eight different variables. The middle layer is the hidden layer where weights and input will be computed using a sigmoid unit. In the end, results will be computed at the output layer. Backpropagation is used for updating weights so that errors can be minimized for predicting class labels. For simplicity, only one hidden layer is shown in the architecture, which in reality is much denser.
Input data from the input layer are computed on the hidden layers with the input values and weights initialized. Every unit in the middle layer called the hidden layer takes the net input, applies activation function “sigmoid” on it, and transforms the massive data into a smaller range between 0 and 1. The calculation is functional for every middle layer. The same procedure is applied on the output layer, which leads to the results towards the prediction for diabetes.
4.2. Diabetes Prediction
It is more beneficial to identify the early symptoms of diabetes than to cure it after being diagnosed. Therefore, in this study, a diabetes prediction system is proposed where three state-of-the-art machine learning algorithms are exploited, and a comparative analysis is performed. The details of the proposed approaches are as follows.
4.2.1. Moving Averages
To predict diabetes, we used moving averages with the experimental setup due to its effectiveness in diabetes prediction for children [ 56 ]. It is based on a calculation that analyzes data points by creating a series of averages of the subset of the data randomly. The moving average algorithm is based on the “forward shifting” mechanism. It excludes the first number from the series and includes the next value in the dataset, as shown in equation ( 3 ). The input values are calculated by averaging ( P SM ) the train data at certain time stamps P M + P M + … P M −( n −1) . The algorithm used past observations as input and predicted future events.
4.2.2. Linear Regression
Second, a linear regression model is applied to the PIMA Indian dataset with the same experimental setup. We used this approach to model a relationship between a dependent variable, that is, outcome in our case, and one or more independent variables. The autonomous variable response affects a lot on the target/dependent variable, as shown in equation ( 4 ). We use a simplified hypothesis and cost function for multivariate linear regression, as there are eight different variables in our dataset [ 57 ]. We choose a very simplified hypothesis function ( h θ ( x )). The aim is to minimize cost function J ( θ ) by choosing the suitable weight ( θ T x ) parameters and minimizing sum of squared error (SSE).
4.2.3. Long Short-Term Memory
For diabetic forecasting, we have calibrated the long short-term memory algorithm with our experimental setup. The proposed approach outperformed as compared to other state-of-the-art techniques implemented, as shown in Table 2 . LSTM is based on recurrent neural network (RNN) architecture, and it has feedback connections that make it suitable for diabetes forecasting [ 58 ]. LSTM mainly consists of a cell, keep gate, write gate, and an output gate, as shown in Figure 3 . The key behind using LSTM for this problem is that the cell remembers the patterns over a long period, and three portals help regulate the information flow in and out of the system. The details are presented in Algorithm 2 .

BG prediction using long short-term memory (LSTM) algorithm.

Diabetes prediction algorithm by exploiting LSTM for healthcare.
Performance comparison of classifiers in diabetes classification.
Algorithm | Accuracy (%) | Recall (%) | Precision (%) |
---|---|---|---|
Logistic regression | 73.05 | 72.7 | 73 |
Random forest | 77.4 | 75.7 | 76.9 |
Proposed fine-tuned MLP | 86.083 | 85.1 | 86.6 |
Input to the algorithm is eight attributes enlisted in Table 3 , measured from healthy and diabetic patients. The proposed LSTM-based diabetes prediction algorithm is trained with 80% of the data, and the remaining 20% is used for testing. We fine-tuned the prediction model by using a different number of LSTM units in the cell state. This fine-tuning helps to identify more prominent features in the dataset. These features will be kept in the cell state of the keep gate of the LSTM and will be given more weightage because they provide more insights to predict BG level. After that, we updated the network's weights by pointwise addition of the cell state and passed only those essential attributes for BG prediction. At this stage, we captured the dependencies between diabetes parameters and the output variable. Finally, the output gate updates the cell state and outputs/forwards only those variables that can be mapped efficiently on the outcome variable.
Description of variables in the dataset.
Attributes | Description | Mean | Std. deviation | Range |
---|---|---|---|---|
Pregnancies | No. of pregnancies | 3.85 | 3.37 | 0–17 |
Glucose | 2 hours of oral glucose tolerance test for plasma glucose concentration | 121 | 32 | 0–199 |
Blood pressure | Blood pressure in mm Hg | 69.1 | 19.3 | 0–122 |
Skin thickness | Skinfold thickness of triceps (mm) | 20.5 | 15.9 | 0–99 |
Insulin | Two hours of serum insulin (mu U/ml) | 79.8 | 115 | 0–846 |
BMI | Body mass index (weight in kg/(height in m) ) | 32 | 7.88 | 0–67 |
Diabetes Pedigree Function | Attribute used in diabetes prognosis | 0.47 | 0.33 | 0.078–2.4 |
Age | Age (years) | 33.2 | 11.8 | 21–81 |
Outcome | Class variable (0 or 1) | 0.35 | 0.48 | Y/N |
The diabetes prediction algorithm consists of three fundamental steps. First, weights are initialized and a sigmoid unit is used in the forget/keep gate to decide which information should be retained from previous and current inputs ( C t −1 , h t −1 , and x t ). The input/write gate takes the necessary information from the keep gate and uses a sigmoid unit which outputs a value between 0 and 1. Besides, a Tan h unit is used to update the cell state C t and combine both outputs to update the old cell state to the new cell state.
Finally, inputs are processed at the output gate and again a sigmoid unit is applied to decide which cell state should be output. Also, Tan h is applied to the incoming cell state to push the output between 1 and −1. If the output of the gate is 1, then the memory cell is still relevant to the required production and should be kept for future results. If the output of the gate is 0, the memory cell is not appropriate, so it should be erased. For the write gate, the suitable pattern and type of information will be determined written into the memory cell. The proposed LSTM model predicts the BG level ( h t ) as output based on the patient's existing BG level ( X t ).
5. Experimental Studies
The proposed diabetes classification and prediction algorithm is evaluated on a publicly available PIMA Indian Diabetes dataset ( https://www.niddk.nih.gov/health-information/diabetes ). Besides, a comparative analysis is performed with state-of-the-art algorithms. The experimental results show the supremacy of the proposed algorithm as compared to state-of-the-art algorithms. The details of the dataset, performance measures, and comparative analysis performed are described in the following sections.
5.1. Dataset
This study used the PIMA Indian Diabetes (PID) dataset taken from the National Institute of Diabetes and Kidney Diseases center [ 59 ]. The primary objective of using this dataset is to build an intelligent model that can predict whether a person has diabetes or not, using some measurements included in the dataset. There are eight medical predictor variables and one target variable in the dataset. Diabetes classification and prediction are a binary classification problem. The details of the variables are shown in Table 3 .
The dataset consists of 768 records of different healthy and diabetic female patients of age greater than twenty-one, as shown in Figure 4 . The feature value distribution is shown in Figure 5 . The target variable outcome contains only two values, 0 and 1. The primary objective of using this dataset was to predict diabetes diagnostically. Whether a user has a chance of diabetes in the coming four years in women belongs to PIMA Indian. The dataset has a total of eight variables: glucose tolerance, no. of pregnancies, body mass index, blood pressure, age, insulin, and Diabetes Pedigree Function. All eight attributes shown in Table 3 are used for the training dataset in the classification model in this work.

PIMA data distribution.

Dataset features' distribution visualization.
5.2. Experimental Result and Discussion
This paper compares the proposed diabetes classification and prediction system with state-of-the-art techniques using the same experimental setup on the PIMA Indian dataset. The following sections highlighted the performance measure used and results attained for classification and prediction, and a comparative analysis with baseline studies is presented.
5.2.1. Performance Metrics
Three widely used state-of-the-art performance measures (Recall, Precision, and Accuracy) are used to evaluate the performance of proposed techniques, as shown in Table 4 . TP shows a person does not have diabetes and identified as a nondiabetic patient, and TN shows a diabetic patient correctly identified as a diabetic patient. FN shows the patient has diabetes but is predicted as a healthy person. Moreover, FP shows the patient is a healthy person but predicted as a diabetic patient. The algorithm utilized 10-fold cross-validation for training and testing the classification and prediction model.
Performance metrics for diabetes classification.
Performance metric | Formula |
---|---|
Recall | TP/(TP+FN) |
Precision | TP/(TP+FP) |
Accuracy | (TP+TN)/(TP+TN+FP+FN) |
For diabetes prediction, the two most commonly used performance measures are the means correlation coefficient ( r /Pearson R ) and root mean square error (RMSE), as shown in Table 5 . R is mainly used to measure the linear dependence strength among the two variables. One variable is for actual value, and another variable is for predicted values. RMSE generates a hint of the overall correctness of the estimate. There can be three values for correlation: 0 for no relation, 1 for positive correlation, and −1 for the negative correlation. RMSE shows the difference between actual values and predicted values.
Performance measure for diabetes prediction.
Performance metric | Formula |
---|---|
Root mean square error (RMSE) | |
Accuracy | (TP+TN)/(TP+TN+FP+FN) |
5.2.2. Attained Results of Diabetic Classification Technique
For diabetic classification, three state-of-the-art classifiers are evaluated on the PIMA dataset. The results illustrate that the fine-tuned MLP algorithm obtained the highest accuracy of 86.083% as compared to state-of-the-art systems, as shown in Table 2 .
It is evident from the results that our proposed calibrated MLP model could be used for the effective classification of diabetes. The proposed classification approach can also be beneficial in the future with our proposed hypothetical system. Data of weight scales, blood pressure monitor, and blood glucometer will be collected through sensor devices such as BLE and input of user's demographic data (for example, date of birth, height, and age). The proposed MLP algorithm outperforms with 86.6% Precision, 85.1% Recall, and 86.083% Accuracy, as shown in Figure 6 . These results are outstanding for decision-making with the proposed hypothetical system to determine patient diabetes, T1D or T2D.

Performance comparison of classifiers.
We also have explored the dataset used in Andy Choens' study [ 27 ]. This dataset consists of records of only one patient. The information was recorded every five minutes. The collection of data was made by using a sensor device (a CGM device). This device allows the patient to store information about BG every five minutes. So, the recorded data by using this device are in massive amounts. Dataset was limited, and most data were noisy that can affect the accuracy of the proposed system, so we neglected it.
5.2.3. Achieved Results of Diabetic Prediction Techniques
For diabetic prediction, we implemented three state-of-the-art algorithms, i.e., linear regression, moving averages, and LSTM. Notably, we fine-tuned LSTM and compared its performance with other algorithms. It is evident from Figure 7 and Table 6 that the LSTM outperformed as compared to other algorithms implemented in this study.

Performance comparison of forecasting model.
Forecasting model comparison for BG.
Algorithm | RMSE | Accuracy | |
---|---|---|---|
Moving average | 0.71 | 42.946 | 75.4 |
Linear regression | −0.071 | 82.592 | 72.7 |
Proposed fine-tuned LSTM | 0.999 | 2.285 | 87.26 |
Table 2 shows the performance values of prediction models with RMSE and r evaluation measures. The proposed fine-tuned LSTM produced the highest accuracy, 87.26%, compared to linear regression and moving average. We can see in Table 6 that the correlation coefficient value is 0.999 using LSTM, −0.071 for linear regression, and 0.710 for moving average, as shown in Figure 7 .
5.2.4. Comparison of the Proposed Method with Baseline Studies
Different baseline studies have been implemented and compared with the proposed system to verify the performance of the proposed diabetes classification and prediction system. Mainly, we focus on those studies that used the PIMA dataset.
First, we compare the state-of-the-art diabetes classification techniques with the proposed technique. All the baseline techniques [ 17 – 19 ] used the PIMA dataset and the same evaluation measures used in this study. In particular, the authors compared naïve Bayes [ 17 ], PCA_CVR (classification via regression) [ 18 ], and SVM [ 19 ] with different machine learning techniques for diabetes classification. However, the proposed fine-tuned MLP-based diabetes classification technique outperformed as compared to baseline studies, as shown in Figure 8 .

Proposed diabetes classification method vs. state-of-the-art techniques.
Several attempts have also been made in the literature for diabetic prediction due to its importance in real life. For this comparison, we have chosen the most recent and state-of-the-art techniques. We compare the proposed system performance with the recent state-of-the-art systems [ 60 – 65 ], as shown in Figure 9 and Table 7 . The proposed method outperformed as compared to state-of-the-art systems with an accuracy of 87.26%, all the compared systems evaluated on the PID with the same experimental setup.

Proposed diabetes prediction method vs. state-of-the-art systems.
Proposed prediction method vs. state-of-the-art systems.
Algorithm | Accuracy (%) |
---|---|
J48 [ ] | 67.9 |
K-mean [ ] | 70.5 |
Feed forward-neural network [ ] | 71.8 |
RB-Bayes [ ] | 72.9 |
Naive Bayes [ ] | 76.3 |
Artificial neural network [ ] | 85.09 |
Proposed method (LSTM) | 87.26 |
6. Proposed Hypothetical IoT-Based Diabetic Monitoring System for Healthcare
This study has also proposed the architecture of a hypothetical diabetic monitoring system for diabetic patients. The proposed hypothetical system will enable a patient to control, monitor, and manage their chronic conditions in a better way at their homes. The monitoring system will store the health activities and create interaction between patients, smartphones, sensor medical devices, web servers, and medical teams by providing a platform having wireless communication devices, as shown in Figure 10 . The central theme of the proposed healthcare monitoring system is the collection of data from sensors using wireless devices and transmitting to a remote server for diagnosis and treatment of diabetes. Knowledge-based data are stored. Rule-based procedures will be applied for the suggestions and treatment of diabetes, informing the patient about his current health condition, prediction, and recommendation of future changes in BG.

The proposed hypothetical architecture of the healthcare monitoring system.
First, essential data about patient health will be collected from sensors such as BLE wireless devices. Data comprised weight, blood pressure, blood glucose, and heartbeat, along with some demographic information such as age, sex, name, and CNIC (Social Security Number). Some information is required in the application installed on the user's mobile and sensor data. All completed data in the application will be transferred to the real-time data processing system. On the other side, aggregate data will be stored in MongoDB for future processing. Analysis and prepossessing techniques are performed to extract rules from the knowledge base for the treatment and suggestions about the user. Results and treatment procedures will be sent to the monitoring system, and finally, the user can get the output by interacting with their android mobile phone. In the end, the patient will know about the health condition and risk prediction of diabetes based on the data transferred by their application and stored data from history about the user.
6.1. Tools and Technology for Implementation of Hypothetical System for Healthcare
The proposed structural design for hypothetical real-time processing and monitoring of diabetes is shown in Figure 11 . The data from the user's mobile will be transmitted in the JavaScript Object Notation (JSON) format to the Application Program Interface (API) in any language. The data produced at this stage will be in the form of messages, which are then transferred to the Kafka application [ 27 ]. Kafka will store all the data and messages and deliver the required data and processed output to the endpoints that could be a web server, monitoring system, or a database for permanent storage. In Kafka, application data are stored in different brokers, which can cause latency issues. Therefore, within the system architecture, it is vital to consider processing the readings from the sensors closer to the place where data are acquired, e.g., on the smartphone. The latency problem could be solved by placing sensors close to the place, such as a smartphone where data are sent and received.

Implementation level details of the proposed hypothetical system.
This inclusion will make the overall network architecture compliant to the emerging Edge and Fog computing paradigms, whose importance in critical infrastructures such as hospitals is gaining momentum. It is essential to consider the Edge and Fog computation paradigm while sending and receiving data from smartphones to increase the performance of the hypothetical system. Edge computing utilizes sensors and mobile devices to process, compute, and store data locally rather than cloud computing. Besides, Fog computing places resources near data sources such as gateways to improve latency problems [ 9 ].
Apache Kafka will be used in real time as a delivery agent for messages in a platform that allows fault-tolerant, tall throughput, and low-latency publication. The vital signs' data collected by the patients are placed using the JSON format and then transmitted using wireless devices with the help of an android application having HTTP along with REST API for the confined remote server for the design [ 28 ]. Moreover, Node.js for web design will be used as a REST API to collect sensor data. Kafka application will receive it in the form of streams of records.
The sensor data that comes from the Kafka application is continuously generated and stored on the server. In the proposed system, the MongoDB NoSQL database will be used for data storage due to its efficiency in handling and processing real-world data [ 29 ]. The stored diabetes patient data can be input into our proposed diabetes classification and prediction techniques to get useful insights.
7. Conclusion
In this paper, we have discussed an approach to assist the healthcare domain. The primary objective of this study is twofold. First, we proposed an MLP-based algorithm for diabetes classification and deep learning based LSTM for diabetes prediction. Second, we proposed an IOT-based hypothetical real-time diabetic monitoring system. The proposed theoretical diabetic monitoring system will use a smartphone, BLE-based sensor device, and machine learning based methods in the real-time data processing environment to predict BG levels and diabetes. The primary objective of the proposed system is to help the users monitor their vital signs using BLE-based sensor devices with the help of their smartphones.
Moreover, the proposed model will help the users to find out the risk of diabetes at a very early stage and help them gaining future predictions of their BG increase levels. For diabetic classification and prediction, MLP and LSTM are fine-tuned. The proposed approaches are evaluated on the PIMA Indian Diabetes dataset. Both approaches are compared with state-of-the-art approaches and outperformed with an accuracy of 86.083% and 87.26%, respectively.
As future work, we plan to implement the android application for the proposed hypothetical diabetic monitoring system with the proposed classification and prediction approaches. Genetic algorithms can also be explored with the proposed prediction mechanism for better monitoring [ 24 , 64 , 66 – 71 ].
Acknowledgments
This work was funded by the School of Computer Sciences, Universiti Sains Malaysia, Penang, Malaysia.
Data Availability
Conflicts of interest.
The authors declare that there are no conflicts of interest regarding the publication of this article.
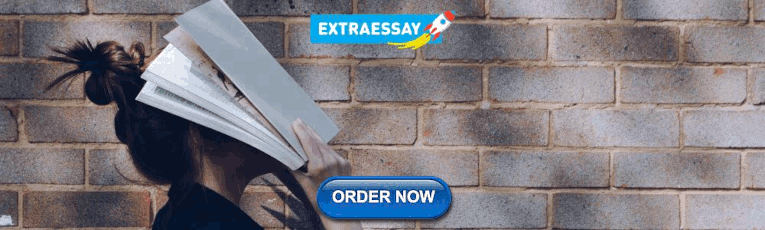
IMAGES
COMMENTS
I. Introduction. Diabetes mellitus, a metabolic disease defined by elevated fasting blood glucose levels due to insufficient insulin production, has reached epidemic proportions worldwide (World Health Organization, 2020).Type 1 and type 2 diabetes (T1D and T2D, respectively) make up the majority of diabetes cases with T1D characterized by autoimmune destruction of the insulin-producing ...
Glycemic, blood-pressure, and lipid control improved from 1999 to 2010 in U.S. adults with diabetes, 10 but recent analyses suggest that progress may have stalled or reversed in later periods. 11 ...
Analysis of data related to the Ukraine famine of 1932-1933 shows that in utero exposure to famine increases the risk of adult type 2 diabetes by more than twofold. Karen O'Leary Research ...
The molecular genetics of diabetes received extensive attention in recent years by many prominent investigators and research groups in the biomedical field. A large array of mutations and single nucleotide polymorphisms in genes that play a role in the various steps and pathways involved in glucose metabolism and the development, control and ...
The best evidence for a link between diabetes mellitus and breast cancer comes from a systematic review of six prospective cohort studies and more than 150,000 women, in which the hazard ratio (HR ...
Diabetes is one of the leading causes of death and disability worldwide, and affects people regardless of country, age group, or sex. Using the most recent evidentiary and analytical framework from the Global Burden of Diseases, Injuries, and Risk Factors Study (GBD), we produced location-specific, age-specific, and sex-specific estimates of diabetes prevalence and burden from 1990 to 2021 ...
Introduction. Insulin resistance and β-cell dysfunction are the 2 major hallmarks of type 2 diabetes mellitus (T2DM) that appear as the result of disturbed homeostasis [].Failure of β-cells (∼80% of their β-cell function) and insulin resistance in muscles and the liver is a vicious triumvirate responsible for the core physiological defects.
Journal of Diabetes Research. Journal of Diabetes Research is an open access journal that publishes articles related to type 1 and type 2 diabetes. Topics include etiology, pathogenesis, management, and prevention of diabetes, as well as associated complications such as nephropathy. As part of Wiley's Forward Series, this journal offers a ...
A paper published in 2014 provided the first demonstration of the use of a bihormonal closed-loop system under free-living conditions in adults and adolescents with type 1 diabetes. Read more.
Two papers—one global and one focused on the USA—together tell the unhappy and inequitable story of diabetes. By 2045, as many as three in four adults with diabetes will be living in low-income and middle-income countries. Currently, only 10% of people with diabetes living in these countries receive guideline-based diabetes care.
Type 2 diabetes accounts for nearly 90% of the approximately 537 million cases of diabetes worldwide. The number affected is increasing rapidly with alarming trends in children and young adults (up to age 40 years). Early detection and proactive management are crucial for prevention and mitigation of microvascular and macrovascular complications and mortality burden.
In their timely article 'Social determinants of health and diabetes: a scientific review', published in Diabetes Care 4, the authors provide an overview of key definitions and social ...
Diabetes publishes original research about the physiology and pathophysiology of diabetes mellitus. Submitted manuscripts can report any aspect of laboratory, animal, or human research. ... Each month, the Editors of Diabetes select an original research article to designate Paper of the Month. View these exclusive articles in a freely ...
In this paper, we provide a review of three methods that have been shown to successfully reverse type 2 diabetes. ... Diabetes Prevention Program Research Group Long-term effects of metformin on diabetes prevention: Identification of subgroups that benefited most in the diabetes prevention program and diabetes prevention outcomes study ...
Introduction. Type 2 Diabetes Mellitus (T2DM) is characterized by a persistently elevated blood glucose, or an elevation of blood glucose after a meal containing carbohydrate ().Unlike Type 1 Diabetes which is characterized by a deficiency of insulin, most individuals affected by T2DM have elevated insulin levels (fasting and/or post glucose ingestion), unless there has been beta cell failure ...
Recent Advances. ADA-funded researchers use the money from their awards to conduct critical diabetes research. In time, they publish their findings in order to inform fellow scientists of their results, which ensures that others will build upon their work. Ultimately, this cycle drives advances to prevent diabetes and to help people burdened by it.
Plasma proteomics in children with new-onset type 1 diabetes identifies new potential biomarkers of partial remission. Olivier G. Pollé. , Sébastien Pyr dit Ruys. & Philippe A. Lysy. Article. 28 ...
Latest Research on Diabetes. Diabetes, a chronic disease that occurs either when the pancreas does not produce enough insulin or when the body cannot effectively use the insulin it produces, is recognized by the World Health Organization as a major cause of mortality around the world. At Dove Medical Press, we have curated the most recent ...
1. Introduction. Type 2 Diabetes Mellitus (T2DM) is one of the most common metabolic disorders worldwide and its development is primarily caused by a combination of two main factors: defective insulin secretion by pancreatic β-cells and the inability of insulin-sensitive tissues to respond to insulin [].Insulin release and action have to precisely meet the metabolic demand; hence, the ...
Diabetes Care is a journal for the health care practitioner that is intended to increase knowledge, stimulate research, and promote better management of people with diabetes.To achieve these goals, the journal publishes original articles on human studies in the following categories: clinical care, education, and nutrition; epidemiology, health services; and psychosocial research; emerging ...
Type 2 diabetes mellitus, the most frequent subtype of diabetes, is a disease characterized by high levels of blood glucose (hyperglycaemia). It arises from a resistance to and relative deficiency ...
Despite a robust amount of research about diabetes, much is still unknown about its effects on oral health. Changes in inflammation are understood to contribute to the impact of diabetes, but what is driving the increase is less clear. ... They both will be coauthors on a paper and both provided valuable data, bringing a lot of enthusiasm ...
The chronic complications of type 2 diabetes are a major cause of mortality and disability worldwide [1, 2]. Clinical research is the main way to gain knowledge about long-term diabetic complications and reduce the burden of diabetes. This allows for designing effective programs for screening and follow-up and fine-targeted therapeutic ...
In Section 6, an IoT-based hypothetical system is presented for real-time monitoring of diabetes. Finally, the paper is concluded in Section 7, outlining the future research directions. 2. Literature Review. In this section, we discussed the classification and prediction algorithms for diabetes prediction in healthcare. Particularly, the ...