Navigation Menu
Search code, repositories, users, issues, pull requests..., provide feedback.
We read every piece of feedback, and take your input very seriously.
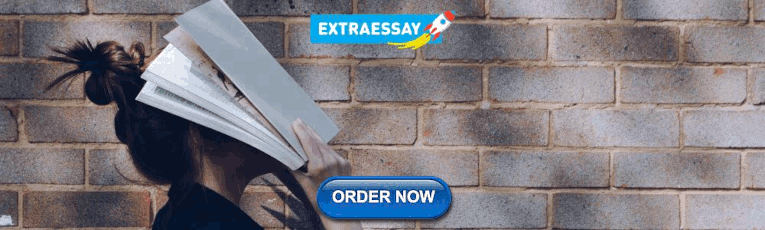
Saved searches
Use saved searches to filter your results more quickly.
To see all available qualifiers, see our documentation .
- Notifications You must be signed in to change notification settings
In the banking industry, detecting credit card fraud using machine learning is not just a trend; it is a necessity for banks, as they need to put proactive monitoring and fraud prevention mechanisms in place. Machine learning helps these institutions reduce time-consuming manual reviews, costly chargebacks and fees, and denial of legitimate tran…
krunal-nagda/Credit-Card-Fraud-Detection-Capstone-Project---Decision-Tree-and-Random-Forest
Folders and files, repository files navigation, credit-card-fraud-detection-capstone-project---decision-tree-and-random-forest.
In the banking industry, detecting credit card fraud using machine learning is not just a trend; it is a necessity for banks, as they need to put proactive monitoring and fraud prevention mechanisms in place. Machine learning helps these institutions reduce time-consuming manual reviews, costly chargebacks and fees, and denial of legitimate transactions.
Suppose you are part of the analytics team working on a fraud detection model and its cost-benefit analysis. You need to develop a machine learning model to detect fraudulent transactions based on the historical transactional data of customers with a pool of merchants.
- Jupyter Notebook 100.0%
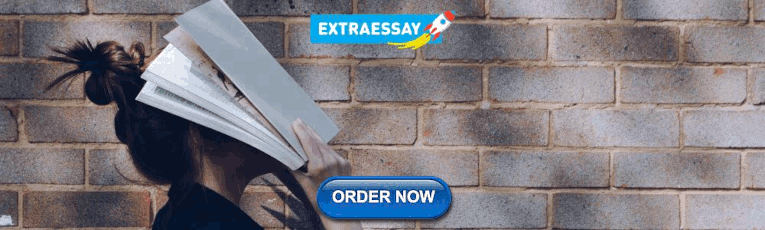
Improving Credit Card Fraud Detection
Using Machine Learning to Profile and Predict Behavior
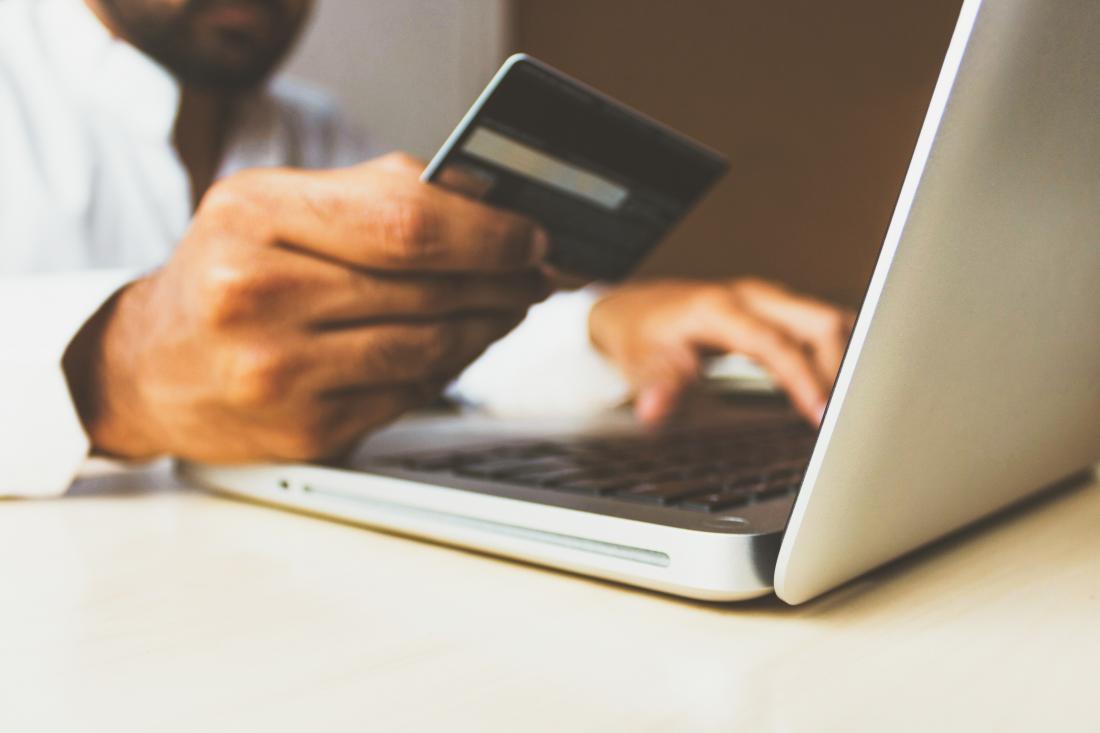
Credit card fraud costs billions of dollars annually, increasing the incentive among financial institutions to develop fast, effective and dynamic fraud detection systems.
Researchers Navin Kasa, Andrew Dahbura, and Charishma Ravoori undertook a capstone project—part of the Master of Science in Data Science program—that addresses credit card fraud detection through a semi-supervised approach, in which clusters of account profiles are created and used for modeling classifiers. Accounts are profiled based on their behavioral trends and clustered into similar groups. Groups are further identified as distinct customer segments based on purchase characteristics such as amount, frequency or distance.
The primary question of this research investigates whether clustering helps improve the predictive performance of credit card fraud.
By engineering useful and descriptive features at the account level, the researchers hypothesized that clustering would be able to separate accounts into meaningful clusters that will improve prediction capabilities. Two baseline models without clustering were generated for comparison against cluster specific models.
Results highlight the potential for optimal classifiers to vary by cluster, suggesting that these classifiers may boost overall fraud detection performance when evaluated using clustering. Additionally, account and transaction characteristics of each cluster should be investigated further to help understand what features are useful in dividing customers. Specifically, clusters that cannot be differentiated must be investigated further to better understand their customer behaviors.
If banks can understand groups of consumers where models perform better or worse, they can begin to investigate and engineer new features that may be more useful.
Further research could investigate whether reassigning accounts in underperforming clusters helps improve performance. It is possible that accounts on the fringe of two customer groups share characteristics that may be useful in predicting fraud when looked at jointly, but are missed by the current model. This also presents the task of determining when accounts should be assigned to new clusters as their behavioral patterns change over time.
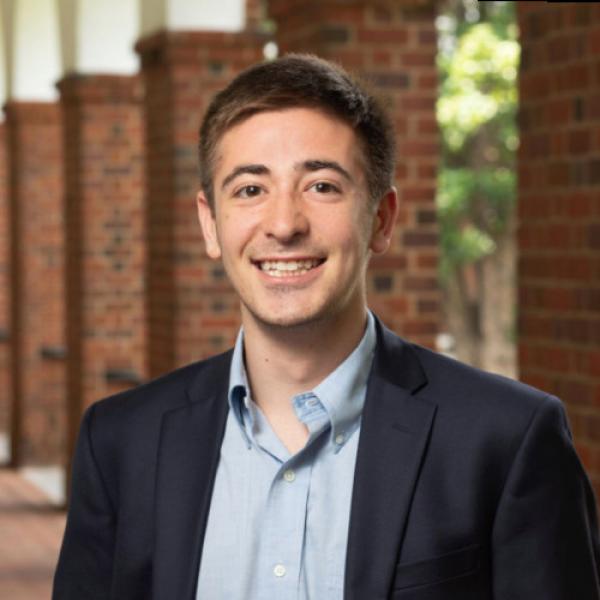
Get the latest news
Subscribe to receive updates from the School of Data Science.
- Prospective Student
- School of Data Science Alumnus
- UVA Affiliate
- Industry Member
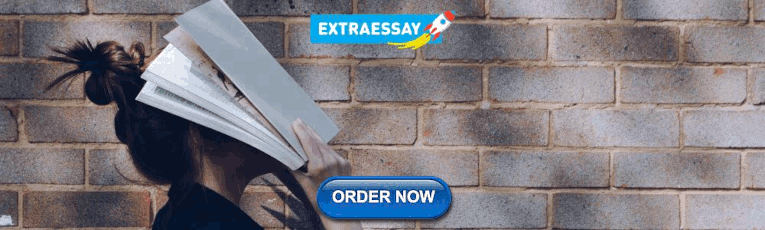
IMAGES
COMMENTS
In this Upgrad/IIIT-B Capstone project, we navigated the complex landscape of credit card fraud, employing advanced machine learning techniques to bolster banks against financial losses. With a focus on precision, we predicted fraudulent credit card transactions by analyzing customer-level data from Worldline and the Machine Learning Group.
Credit Card Fraud Detection Project. Problem Statement: The problem statement chosen for this project is to predict fraudulent credit card transactions with the help of machine learning models.
"Combatting financial threats, the Credit Card Fraud Detection Capstone Project empowers learners to build robust models using data analysis and machine learning. Tackling real-world challenges, it culminates in a deployable system to safeguard transactions, showcasing skills in fraud prevention and data-driven security.
In the banking industry, detecting credit card fraud using machine learning is not just a trend; it is a necessity for banks, as they need to put proactive monitoring and fraud prevention mechanisms in place.
Credit card fraud detection. Capstone Breakdown. Duration of specialized content (Kafka streaming): ~1 week. Estimated project duration: ~4 weeks *Note that the durations above are only suggested durations for the theory and the capstone project. It may be possible to keep studying the theory while starting the capstone project simultaneously.
Explore and run machine learning code with Kaggle Notebooks | Using data from Credit Card Fraud Detecion.
detect these fraudulent transactions in time in order to help consumers as well as banks, who are losing their credit worth each day. Every fraudulent credit card transaction that occur is a direct financial loss to the bank as the bank is
Credit-Card-Fraud-Detection-Capstone-Project---Decision-Tree-and-Random-Forest. In the banking industry, detecting credit card fraud using machine learning is not just a trend; it is a necessity for banks, as they need to put proactive monitoring and fraud prevention mechanisms in place.
Researchers Navin Kasa, Andrew Dahbura, and Charishma Ravoori undertook a capstone project that addresses credit card fraud detection through a semi-supervised approach, in which clusters of account profiles are created and used for modeling classifiers.
Exploring the intricacies of how credit card fraud operates was crucial in developing the machine learning-based fraud detection project I led. Here’s a step-by-step breakdown, informed by real-life scenarios and the deployment of a credit card fraud detection project: