- Open access
- Published: 10 June 2024
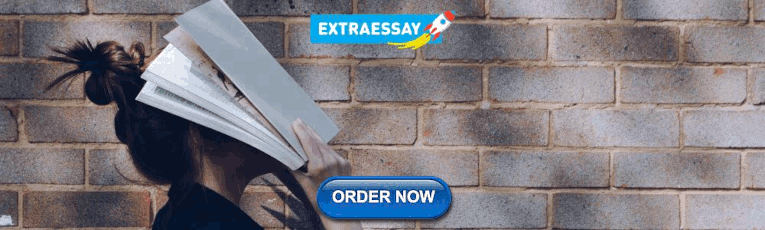
New advances in the diagnosis and treatment of autism spectrum disorders
- Lei Qin 1 ,
- Haijiao Wang 2 ,
- Wenjing Ning 1 ,
- Mengmeng Cui 1 &
- Qian Wang 3
European Journal of Medical Research volume 29 , Article number: 322 ( 2024 ) Cite this article
1978 Accesses
1 Citations
Metrics details
Autism spectrum disorders (ASD) are a group of neurodevelopmental disorders that affect individuals' social interactions, communication skills, and behavioral patterns, with significant individual differences and complex etiology. This article reviews the definition and characteristics of ASD, epidemiological profile, early research and diagnostic history, etiological studies, advances in diagnostic methods, therapeutic approaches and intervention strategies, social and educational integration, and future research directions. The highly heritable nature of ASD, the role of environmental factors, genetic–environmental interactions, and the need for individualized, integrated, and technology-driven treatment strategies are emphasized. Also discussed is the interaction of social policy with ASD research and the outlook for future research and treatment, including the promise of precision medicine and emerging biotechnology applications. The paper points out that despite the remarkable progress that has been made, there are still many challenges to the comprehensive understanding and effective treatment of ASD, and interdisciplinary and cross-cultural research and global collaboration are needed to further deepen the understanding of ASD and improve the quality of life of patients.
Autism spectrum disorders (ASD) are a broad group of neurodevelopmental disorders that affect an individual's social interactions, communication skills, and behavioral patterns [ 1 , 2 ]. The characteristics of ASD vary significantly between individuals, from mild social impairments to severe communication and behavioral problems, a diversity that reflects the use of the term “spectrum” [ 3 ]. Although the exact causes of ASD are not fully understood, research suggests that both genetic and environmental factors play a key role in its development [ 4 ].
Characteristics of ASD
Difficulties in social interaction.
Individuals with ASD often exhibit significant difficulties in social interactions. These difficulties may include difficulty understanding the feelings and intentions of others, maintaining eye contact and facial expressions, and adapting to social norms and expectations. Individuals with ASD may experience challenges in establishing and maintaining friendships, they may not understand the two-way nature of social interactions, or they may feel uncomfortable sharing interests and activities [ 5 ].
Communication disorders
Communication deficits are another core feature of ASD. This may manifest itself in delays in language development, including delays in uttering first words or simple sentences. Some individuals with ASD may not use language to communicate at all. Even among individuals with ASD who have normal language skills, they may have difficulty using language in conversations to communicate thoughts, feelings, or needs. In addition, nonverbal communication, such as the understanding and use of body language and facial expressions, may also be affected [ 6 ].
Repetitive behaviors and interests
Individuals with ASD often display restricted, repetitive patterns of behavior and interests. These may include a strong fixation on specific topics or activities, repetitive body movements (e.g., rocking, clapping), and an overreliance on daily routines. These repetitive behaviors are sometimes seen as a way of self-soothing or as an attempt to control an environment that otherwise feels unpredictable and overwhelming to them [ 7 ].
Sensory sensitivity
Many individuals with ASD have abnormalities in sensory processing and may have very strong or delayed responses to sound, light, touch, taste or odor. For example, some individuals with ASD may find background noises in their everyday environment unusually harsh, or they may not notice pain or other bodily sensations [ 8 ].
Epidemiologic profile of ASD
According to the World Health Organization (WHO), the average prevalence of ASD among children globally is approximately 1% [ 9 ]. However, this figure varies significantly between regions and countries. For example, the Centers for Disease Control and Prevention (CDC) reports that the prevalence of ASD among 8-year-olds in the U.S. is 1 to 54. ASD is significantly more prevalent in males than females, at a ratio of approximately 4:1 [ 10 ]. This gender difference may reflect differences in genetic susceptibility and/or gender bias in the diagnostic process. Early diagnosis is key to improving developmental outcomes for children with ASD. Despite this, many children are not diagnosed by age 3. The CDC reports that most children are first evaluated for ASD by age 4, but diagnosis may occur later. Research suggests that ASD is highly heritable, but multiple genetic variants are associated with disease risk and environmental factors also play a role [ 11 ]. For example, there is an increased risk of ASD in preterm and low birth weight infants. Socioeconomic factors influence ASD diagnosis and treatment access. Families of lower socioeconomic status may face greater challenges, including barriers to accessing early intervention services, etc. ASD is a global public health problem, and its incidence, time to diagnosis, and treatment access are influenced by multiple factors [ 12 ]. Ongoing epidemiologic research and the advancement of a deeper understanding of ASD are critical to the development of effective prevention, diagnosis, and interventions.
Historical background
Early history of research and diagnosis of asd.
The concept of ASD was first clearly defined in the 1940s, when a group of children exhibiting extreme self-isolation and lack of responsiveness to the environment was first described by American psychiatrist Leo Kanner [ 13 ]. Almost simultaneously, Austrian child psychologist Hans Asperger described a similar but higher level of functioning in a condition that came to be known as Asperger’s syndrome [ 14 ]. These two independent studies laid the foundation for the modern understanding of ASD. For the first few decades, ASD was considered extremely rare and was often confused with schizophrenia. Due to a lack of in-depth understanding of ASD, early diagnostic criteria were unclear and treatment was largely limited to behavioral interventions and psychotherapy. Over time, researchers began to pay more attention to the genetic and neurobiological underpinnings of ASD, thus contributing to a more comprehensive understanding of this complex condition. Since the 1990s, the diagnosis of ASD has risen significantly, as diagnostic criteria have continued to be refined and public awareness has increased. This period has also witnessed an increased awareness of the importance of early diagnosis and intervention for ASD, which has led to significant improvements in the prognosis and quality of life for many children and adults with ASD [ 15 ].
Evolution of research paradigms
The research paradigm for ASD has undergone a remarkable evolution since the mid-twentieth century, a process that reflects a deepening of the understanding of ASD as well as advances in scientific research methods [ 16 ]. In the early stages, ASD research focused on behavioral observations and psychoanalysis, when ASD was often mistaken for an emotional disorder due to an indifferent mother. During this period, understanding of ASD was relatively limited and treatments focused primarily on psychotherapy and behavior modification. Into the second half of the twentieth century, with advances in genetics and neuroscience, researchers began to explore the biological basis of ASD. This marked a shift from a psychosocial to a biomedical model, and the focus of research gradually shifted to genetic factors and abnormalities in brain structure and function. Through a large number of family and twin studies, scientists found that ASD has a high genetic predisposition, while neuroimaging studies revealed the specificity of brain development in ASD patients. In the twenty-first century, with the application of bioinformatics and high-throughput gene sequencing technology, the study of ASD has entered a new stage [ 17 ]. Researchers have not only been able to identify specific genetic variants associated with ASD, but have also begun to explore the interaction between environmental factors and genetic susceptibility. In addition, the adoption of interdisciplinary research approaches, such as combining neuroscience, genetics, psychology, and computational modeling, has provided new perspectives for understanding the complexity of ASD.
Recently, the concepts of precision medicine and personalized treatment strategies have been introduced to the study of ASD, aiming to develop customized intervention programs based on each patient’s genetic background and symptom profile. With advances in technology and improved methods of data analysis, future research on ASD is expected to reveal more knowledge about its pathomechanisms and provide more effective support and treatment for patients with ASD.
Etiologic studies
Genetic factors, monogenic genetic cases.
The etiology of ASD is multifactorial, involving a complex interaction of genetic and environmental factors. Although most cases of ASD are thought to be the result of polygenic interactions, there are some cases that are directly associated with variations in a single gene, and these are referred to as monogenic genetic cases. Monogenic genetic cases provide an important window into understanding the genetic basis of ASD, although they represent a relatively small proportion of all ASD cases [ 18 ]. A number of specific genetic syndromes, such as fragile X syndrome, tuberous sclerosis, 15q11-q13 duplication syndrome, and Rett syndrome, have been found to be associated with a higher risk of ASD. These conditions, often caused by mutations or abnormalities in a single gene, can lead to significant differences in brain development and function, thereby increasing the probability of an ASD phenotype. Fragile X syndrome is one of the most common forms of inherited intellectual disability and the single-gene disorder known to be most strongly associated with ASD. It is caused by a repeat expansion on the FMR1 gene [ 19 ]. Tuberous sclerosis (TSC) is an inherited disorder that affects multiple systems and is caused by mutations in the TSC1 or TSC2 genes, and the prevalence of ASD is higher in patients with TSC. 15q11-q13 duplication syndrome (Dupuy 15q syndrome) involves a region of chromosome 15, the duplication of which is associated with an increased risk of ASD [ 20 ]. Rett syndrome, which predominantly affects females, is caused by mutations in the MECP2 gene, and patients often exhibit some of the features of ASD, such as impaired social interactions [ 21 ]. The association of these classical candidate genes with ASD is summarized in Table 1 .
The discovery of these monogenic genetic cases is not only crucial for understanding the genetic mechanisms of ASD, but also potentially valuable for the development of interventional and therapeutic strategies targeting specific genetic variants. However, even in these cases, the expression of the genetic variants showed a degree of heterogeneity, suggesting that the diversity of phenotypic features and clinical manifestations, even in monogenic genetic cases, may be influenced by other genetic and environmental factors. Therefore, an in-depth study of these conditions will not only improve our understanding of the genetic basis of ASD, but also provide clues for the development of more personalized therapeutic strategies.
Multigene interactions
The development of ASD is widely recognized as a result of the interaction of genetic and environmental factors, with polygenic interactions occupying a central position in the genetic background of the disease. Unlike monogenic cases, polygenic interactions involve variants or polymorphisms in multiple genes that together increase the risk of ASD. These genetic variants may contribute a smaller effect in each individual, but when acting together they can significantly increase the probability of ASD development [ 30 ]. Current research suggests that no single gene can explain all cases of ASD. Instead, hundreds of genetic loci have been identified that are associated with an increased risk of ASD. These genes are often involved in key processes such as brain development, neuronal signaling, and intercellular communication, suggesting that ASD involves extensive regulation of brain function and structure. The complexity of multigene interactions means that genetic studies of ASD require large-scale genomic data and sophisticated statistical methods to reveal those genomic variants that increase risk.
Meta-analyses of large-sample genome-wide association studies (GWAS) have identified several consistently replicated ASD risk gene loci, such as those in the chromosomal regions 3p21, 5p14, 7q35, and 20p12. These loci contain genes like CNTN4, CNTNAP2, and NRXN1, which play crucial roles in neurodevelopment and synaptic function, particularly in processes such as synaptic adhesion and neurotransmission. These findings provide a more robust understanding of the genetic architecture of ASD and highlight the importance of integrating genetic findings with functional studies to advance our understanding of the disorder. They also have implications for future research, such as the development of personalized diagnostic and therapeutic strategies based on an individual's genetic profile. Through genome-wide association studies (GWAS) and other genomic approaches, scientists are gradually unraveling the genetic landscape of this complex disease. Understanding the impact of multiple gene interactions on ASD not only helps us understand its genetic basis, but also opens up the possibility of developing personalized treatment and intervention strategies [ 31 ].
Environmental factors
Maternal exposure.
Exposure during pregnancy refers to a mother’s exposure to specific environmental factors or substances during fetal development, which may increase the child's risk of developing ASD in the future. These exposures include certain prescription medications (e.g., anti-seizure medications and opioids), environmental pollutants (e.g., heavy metals and air pollutants), infections (e.g., rubella and influenza viruses), and poor nutrition or deficiencies in specific nutrients (e.g., folic acid). These factors may increase the risk of ASD by affecting fetal brain development and the maturation process of the nervous system. Understanding the effects of exposure during pregnancy can help to take preventive measures to reduce the incidence of ASDs [ 32 ].
Effects of early developmental stages
The early developmental stages of ASD are influenced by a variety of factors that include genetic predisposition, environmental exposures, and early life experiences. During a child's early development, the brain experiences rapid growth and the formation of neural networks. Any disruption during this critical period may interfere with the proper development of brain structure and function, thereby increasing the risk of ASD. For example, very early lack of social interaction, delayed language development or abnormal sensory processing may be early signs of ASD. These developmental abnormalities reflect difficulties in the brain’s nervous system in processing information, making connections and adapting to environmental changes. Early identification and intervention are essential to promote optimal development in children with ASD [ 33 ].
Genetic–environmental interactions
The genetic–environmental interactions are summarized in Fig. 1 . ASD develops as a result of the interaction between genetic and environmental factors, and this interaction reflects the complexity of the combination of genetic background and external environmental factors that influence ASD risk. Specifically, certain genetic susceptibilities may be activated in response to environmental triggers, leading to the development of ASD. For example, genetic variants may make individuals more sensitive to certain environmental exposures (e.g., substance use during pregnancy, environmental pollutants, or maternal nutritional status), which together may increase the risk of ASD by acting on key brain developmental stages [ 34 ]. This complex genetic–environmental interaction underscores the need to understand multifactorial etiological models of ASD and the importance of developing personalized intervention strategies.
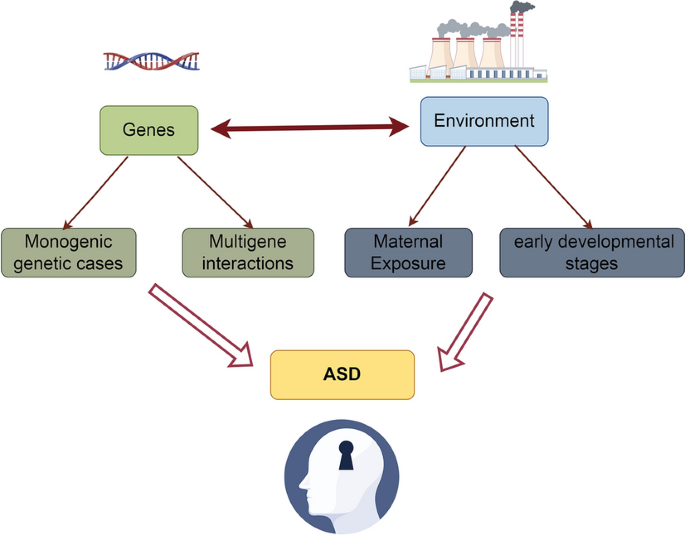
Advances in diagnostic methods
Traditional diagnostic methods.
Traditional diagnostic methods for ASD rely heavily on detailed assessments of behavior and developmental history. These assessments are usually conducted by specialized health care providers such as pediatricians, neuropsychologists, or psychiatrists. The diagnostic process encompasses direct observation of the child as well as in-depth interviews with parents or caregivers to gather information about the child's social interactions, communication skills, and behavioral patterns [ 35 ]. Diagnostic tools include, but are not limited to, the Childhood Autism Rating Scale (CARS), the Autism Diagnostic Observation Scale (ADOS), and the Autism Diagnostic Interview-Revised (ADI-R). These tools are designed to identify core symptoms of ASD, such as social communication deficits and repetitive behaviors or interests. In addition, the doctor may perform a series of developmental or cognitive assessments to rule out other conditions that may explain the child’s behavior, such as language disorders or other neurodevelopmental disorders [ 36 ]. While these traditional diagnostic methods are highly effective in recognizing ASD, they rely on subjective assessments and the experience of the professional, and therefore may have some degree of variability. In recent years, with a deeper understanding of ASDs, new diagnostic techniques and methods are being developed and adopted to improve diagnostic accuracy and efficiency.
Latest diagnostic techniques and tools
Genetic testing.
Genetic testing for ASD is a method of identifying risks associated with ASD by analyzing genetic variants in an individual's DNA. This testing looks for specific genetic variants that have been linked by scientific research to the development of ASD. Although the genetic background of ASD is extremely complex, involving multiple genes and the interaction of genes with environmental factors, variants in specific genes have been identified as having a significant impact on ASD risk [ 37 ]. For example, variants in the SHANK3 gene are associated with Phelan–McDermid syndrome, and patients with this syndrome often exhibit ASD features. Variants in the FMR1 gene are responsible for fragile X syndrome, which is the most common single-gene cause of ASD known to be associated with ASD. Mutations in the MECP2 gene have been associated with Rett syndrome, and patients with Rett syndrome often exhibit ASD condition. In addition, variants in the NRXN1 and NLGN3/4 genes have been found to increase the risk of ASD [ 38 ]. Genetic testing can help provide more precise diagnostic information, and in those cases of ASD where the cause is unknown, it may even reveal the underlying genetic cause. This will not only help to understand the genetic mechanisms of ASD, but also provide more targeted intervention and support strategies for patients and families.
Neuroimaging
Neuroimaging techniques in the study of ASD provide a non-invasive way to explore changes in brain structure and function, helping scientists better understand the biological basis of ASD. These techniques include functional magnetic resonance imaging (fMRI), structural magnetic resonance imaging (sMRI), diffusion tensor imaging (DTI), and positron emission tomography (PET). Through these neuroimaging techniques, researchers are able to observe structural and functional differences in specific regions and networks of the brain in individuals with ASD [ 39 ]. For example, fMRI can reveal patterns of brain activity when performing specific tasks, helping to understand the impairments in social, language, and cognitive functioning in individuals with ASD. dTI focuses on the microstructure of the brain’s white matter, revealing the connections of bundles of nerve fibers, which can help to study neural connectivity issues in ASD. PET scans, on the other hand, are able to assess the activity of specific chemicals in the brain, providing clues to study the neurochemical basis of ASD [ 40 ]. With these advanced neuroimaging techniques, researchers will not only be able to delve deeper into the neurodevelopmental abnormalities of ASD, but also identify possible novel therapeutic targets that can provide a scientific basis for developing more effective interventions. However, while these techniques provide valuable perspectives in understanding ASD, a complete understanding of the complexity of the brain remains a challenge for future research.
Early screening methods
Recently, the field of early screening for ASD has witnessed the application of a number of innovative techniques designed to improve the accuracy and convenience of screening. One notable new approach is the use of artificial intelligence (AI) and machine learning techniques to analyze children's behavioral videos and biomarkers. By training algorithms to recognize specific behavioral patterns and physiological signals associated with ASD, these technologies can help physicians and researchers identify potential ASD symptoms earlier [ 41 ]. Another area of innovation is eye-tracking technology, which assesses children’s social and cognitive development by analyzing their eye movement patterns when viewing pictures or videos. Studies have shown that the eye movement patterns of children with ASD while viewing social scenes differ from those of typically developing children, providing a non-invasive window for early screening [ 42 ]. The application of these state-of-the-art technologies not only improves the efficiency and accessibility of early screening, but also provides new perspectives for understanding the complexity and individual differences in ASD [ 43 ]. Although these approaches are still in the research and development stage, they demonstrate the great potential of utilizing technological advances to improve the process of ASD screening and diagnosis. With further validation and refinement of these techniques, it is expected that they will make a significant contribution to the early identification and intervention of ASD in the future.
Treatment approaches and intervention strategies
Behavioral and educational interventions, applied behavior analysis (aba).
Applied behavior analysis (ABA) is an intervention approach based on the principles of behavioral psychology that is widely used in the treatment of children with autism spectrum disorders (ASD). ABA works to understand and improve specific behaviors, particularly to enhance social, communication, academic skills, and daily living skills, while reducing maladaptive behaviors. It helps individuals learn new skills and behaviors by systematically applying reinforcement strategies that encourage and reward desired behaviors [ 44 ]. ABA therapy is highly individualized and customized to each child’s specific needs and abilities. Treatment planning begins with a detailed behavioral assessment to identify target behaviors and intervention strategies. Learned behaviors are then reinforced and cemented through one-on-one teaching sessions using positive reinforcement. ABA also emphasizes the importance of data, which is collected and analyzed on an ongoing basis by the therapist to monitor progress and adjust the treatment plan as necessary [ 45 ]. Research has shown that ABA is an effective way to improve social interactions, communication skills, and learning in children with ASD. Through early and consistent intervention, ABA can significantly improve the independence and overall quality of life of children with ASD. Although ABA treatment requires a commitment of time and resources, the long-term benefits it brings to children with ASD and their families are immeasurable.
Social skills training
Social skills training (SST) for children with autism spectrum disorders (ASD) is an intervention designed to improve their ability to interact socially in everyday life. This training focuses on teaching children with ASD the ability to understand social cues, establish effective communication skills, and develop friendships. Through SST, children learn how to recognize and interpret other people's facial expressions, body language, and social etiquette, which are essential for building positive relationships [ 46 ]. Social skills training typically includes a series of structured instructional activities such as role-playing, social stories, interactive group exercises, and peer modeling. These activities are designed to provide practice in real-world social situations in a supportive and interactive manner, helping children with ASD learn and practice new skills in a safe environment [ 47 ]. In addition, SST can include teaching emotion management and conflict resolution skills to help children with ASD better understand and express their emotions and cope with challenges in social interactions. Through regular and consistent practice, children with ASD can improve their self-confidence, increase their social engagement, and ultimately improve their social competence and quality of life. SST has been shown to be significantly effective in enhancing social adjustment and interpersonal interactions in children with ASD [ 48 ].
Medical treatment
While there is no cure for ASD, certain medications can be used to manage specific symptoms associated with ASD, such as behavioral problems, attention deficits, anxiety, and mood swings that are common in individuals with autism. Medication is often used as part of a comprehensive intervention program designed to improve the quality of life and daily functioning of the patient [ 49 ]. Medications commonly used for ASD symptom management include antipsychotics, antidepressants, stimulants, and anxiolytics. For example, two antipsychotics, risperidone and aripiprazole, have been approved by the FDA for the treatment of stereotypic and aggressive behavior in children and adolescents with ASD. In addition, selective serotonin reuptake inhibitors (SSRIs) may be helpful in managing anxiety and depressive symptoms in individuals with ASD.
Importantly, medication needs to be closely monitored by a physician to ensure the effectiveness and safety of the medications, as they may have side effects. We have summarized the research evidence on the efficacy and safety of commonly used medications in ASD, including antipsychotics for treating irritability and aggression, antidepressants for co-occurring anxiety and depression, and other medications such as stimulants and melatonin. While these medications can be helpful in managing specific symptoms, they also carry potential side effects and risks, such as weight gain, metabolic disturbances, and behavioral activation. Therefore, a thorough diagnostic evaluation, individualized treatment planning, close monitoring, and regular follow-up are essential when considering pharmacotherapy for individuals with ASD. The decision to medicate should be based on an individualized assessment that takes into account the patient’s specific needs, the severity of symptoms, and possible side effects. At the same time, pharmacological treatments are often used in combination with non-pharmacological treatments such as behavioral interventions and educational support to achieve optimal therapeutic outcomes [ 50 ].
Biofeedback and neuromodulation
Biofeedback and neuromodulation are innovative approaches that have been explored in recent years in the treatment of ASD, aiming to reduce ASD symptoms by improving brain function. Biofeedback techniques enable individuals to learn how to control physiological processes that are not normally under conscious control, such as heart rate, muscle tension, and brainwave activity. Through real-time feedback, patients can learn how to regulate their physiology, resulting in improved concentration, reduced anxiety, and improved emotional regulation. Neuromodulation, specifically transcranial magnetic stimulation (TMS) and transcranial direct current stimulation (tDCS), affects neural activity in the brain through external stimulation. tMS utilizes a magnetic field to affect neuronal activity in specific areas of the brain, while tDCS modulates neuronal excitability by applying a weak electrical current. These methods have been studied for improving social communication skills and reducing stereotypical behaviors in people with ASD [ 51 ].
Biofeedback helps individuals develop self-regulation skills by providing real-time feedback on physiological states, while neuromodulation techniques like TMS and tDCS modulate cortical excitability and neural plasticity in aberrant circuits implicated in ASD. Current research suggests potential benefits of these techniques in improving emotional regulation, social functioning, and cognitive performance, but mixed results highlight the need for larger, well-controlled trials to validate efficacy, safety, and optimal protocols. Despite challenges, these techniques show promise as adjunctive therapies in the comprehensive management of ASD, warranting further research to guide their translation into clinical practice. Although biofeedback and neuromodulation show potential in the treatment of ASD, research on these techniques is currently in its infancy. More clinical trials and studies are needed to evaluate their effectiveness, safety, and long-term effects and to determine which patients may benefit from these interventions. Nevertheless, as non-pharmacologic treatments, they offer promising complementary options to the comprehensive treatment of ASD.
Emerging intervention approaches
Technology-assisted interventions.
Technology-assisted interventions have become an important development in the field of ASD treatment in recent years, providing new ways for children with ASD to learn and communicate. These interventions utilize computers, tablets, smartphone apps, and virtual reality technology to design a range of interactive learning tools and games designed to improve social skills, communication, and cognitive functioning in children with ASD [ 52 ]. A key advantage of technology-assisted interventions is their ability to provide highly personalized learning experiences. Software and applications can be adapted to a child's specific needs and interests, ensuring that learning content is both engaging and appropriate to the individual's developmental level. In addition, the feedback provided by technology is often immediate and consistent, helping children with ASD to better understand and process information. The use of virtual reality technology, by simulating social situations, provides a safe and controlled environment for children with ASD to practice social interaction and problem-solving skills, which is often difficult to achieve in traditional educational and therapeutic settings [ 53 ]. Although technology-assisted interventions have demonstrated great potential, research on their long-term effects and optimal implementation is still ongoing. To maximize the benefits of these tools, it is often recommended that technology-assisted interventions be used in conjunction with other therapeutic approaches to provide a comprehensive intervention program.
Diet and nutrition interventions
Dietary and nutritional interventions have received increasing attention in the treatment of ASD, based on the observed potential link between nutritional imbalances and ASD symptoms. This intervention approach aims to improve the behavioral performance and overall health of children with ASD by optimizing their diet. Specific strategies include restricting certain foods that may exacerbate symptoms, such as gluten and lactose, as well as increasing intake of foods rich in essential nutrients to support brain development and function [ 54 ]. Several studies support the potential benefits of specific dietary interventions, such as implementing a gluten-free lactose-free (GFCF) diet, which may help improve behavioral and digestive symptoms in some children with ASD. In addition, supplementation with omega-3 fatty acids, vitamins, and minerals (e.g., magnesium and zinc) have been proposed as potentially beneficial strategies to support neurologic health and alleviate ASD-related symptoms [ 55 ]. However, the effectiveness of dietary and nutritional interventions may vary by individual and more scientific research is needed to gain a deeper understanding of their long-term effects on children with ASD. Before implementing any dietary intervention, it is recommended to consult with a physician or nutritional expert to ensure that the individual needs of the child are met and to avoid malnutrition. In combination, dietary and nutritional interventions can be used as part of a comprehensive treatment plan for ASD, complementing traditional behavioral and educational interventions.
Social and educational integration
Educational integration of children with asd.
Educational integration of children with ASD is an inclusive educational practice that seeks to integrate children with ASD into the mainstream educational system to learn and grow with their typically developing peers. This integration model emphasizes individualized learning plans and adaptive teaching strategies to meet the unique needs of children with ASD while promoting their social inclusion and emotional development. Through educational integration, children with ASD are provided with opportunities to interact with other children, which is essential for them to learn social skills, enhance their communication abilities, and improve their ability to adapt to society. To support the successful integration of children with ASD, schools often provide special education services such as speech and language therapy, occupational therapy, and behavioral interventions, which take place in classroom settings to ensure their academic and social progress. Educational inclusion is not only beneficial for children with ASD, but it also helps to foster a sense of inclusion and diversity among their peers. By learning and playing together, all children learn to respect and understand differences, laying the foundation for a more inclusive society. However, effective integrated education requires close collaboration among teachers, parents and professionals, as well as the availability of appropriate resources and support systems [ 56 ].
Social integration and employment of adults with ASD
The social integration and employment of adults with ASD is a current focus of attention in ASD research and social services. For many adults with ASD, social integration challenges include establishing stable relationships, participating in community activities, and finding and keeping a job. Although adults with ASD may have unique skills and interests in specific areas, social communication deficits and fixed patterns of behavior may make it difficult for them in traditional work settings. In recent years, more and more organizations and businesses have begun to recognize the value of diversity and inclusion and are working to create work environments that are better suited for adults with ASD. This includes providing flexible work arrangements, clear communication guidelines, and individualized support measures such as workplace co-worker support and professional career counseling. In addition, social service programs and non-profit organizations offer training and job readiness programs specifically designed for adults with ASD to help them develop necessary vocational skills and social competencies. Through these efforts, adults with ASD will not only be able to find jobs that meet their interests and abilities, but also find a place for themselves in society, enhancing their independence and life satisfaction. However, the realization of this goal requires sustained social awareness-raising and the construction of an ASD-friendly environment [ 57 ].
Future research directions
Application of precision medicine in asd treatment.
The application of precision medicine in the treatment of ASD represents a paradigm of a personalized treatment strategy that aims to tailor the treatment plan to each patient's genetic information, biomarkers, history of environmental exposure, and lifestyle factors. The philosophy behind this approach is that, although ASD is classified as a spectrum, each patient's etiology, symptoms, and their severity are different, and therefore treatment should be highly individualized [ 58 , 59 ]. By fully sequencing a patient's genome, scientists and physicians can identify specific genetic variants that may affect ASD symptoms, allowing them to develop targeted treatments. For example, if a particular ASD patient's symptoms are linked to an abnormality in a specific metabolic pathway, that pathway could be modulated through dietary adjustments, nutritional supplements, or specific medications with a view to improving symptoms. In addition, precision medicine involves the consideration of environmental factors and personal behavior to ensure that treatment options are not only scientifically effective, but also appropriate to the patient's lifestyle. Although precision medicine is still in its early stages in the field of ASD, it offers great potential for delivering more personalized and effective treatment regimens, which are expected to significantly improve the quality of life of people with ASD [ 60 ].
Prospects for emerging biotechnologies
Emerging biotechnologies in the field of ASD, such as gene editing, stem cell therapies, and biomarker development, are opening up new possibilities for treating and understanding ASD. Gene editing technologies, particularly the CRISPR-Cas9 system, provide researchers with the means to precisely modify genetic variants associated with ASD, promising to reveal how specific genetic variants affect brain development and function, thereby providing clues for the development of targeted therapies [ 61 ]. Stem cell therapies utilize a patient's own induced pluripotent stem cells (iPSCs) to study the pathomechanisms of ASD by mimicking the neurodevelopmental process in vitro, as well as exploring potential cellular alternative treatments. In addition, the discovery of biomarkers facilitates early diagnosis and monitoring of disease progression, making personalized treatment possible [ 62 ]. In addition, induced pluripotent stem cell (iPSC)-derived brain organoids from ASD patients have emerged as a powerful tool for studying the neurodevelopmental abnormalities associated with ASD. These 3D, self-organizing models recapitulate key features of human brain development in vitro, allowing researchers to investigate the cellular and molecular mechanisms underlying ASD pathogenesis. By comparing brain organoids derived from ASD patients with those from healthy controls, researchers can identify alterations in neuronal differentiation, migration, and connectivity that may contribute to the development of ASD. Moreover, patient-derived brain organoids provide a personalized platform for drug screening and testing, enabling the identification of targeted therapies that can be tailored to an individual's genetic background. This approach has the potential to revolutionize the development of precision medicine strategies for ASD, by providing a more accurate and relevant model system for investigating disease mechanisms and testing novel therapeutic interventions. As the field continues to advance, iPSC-derived brain organoids are expected to play an increasingly important role in unraveling the complex etiology of ASD and guiding the development of personalized treatment strategies [ 63 ]. The development of these technologies has not only improved our understanding of the complex etiology of ASD, but also provided more precise and effective treatment options for ASD patients. Although most of these emerging biotechnologies are still in the research phase, they bring hope and anticipation for the future of ASD treatment and management. As research progresses and technology matures, it is expected that these innovative approaches will bring substantial benefits to individuals with ASD and their families.
Interaction between social policy and ASD research
The interaction between social policy and ASD research is key to achieving better social inclusion and quality of life for individuals with ASD and their families. Effective social policies can provide the necessary financial support and legal framework for ASD research, promoting a deeper understanding of ASD and the development of new treatments. For example, policies can promote collaboration in interdisciplinary research, encourage the use of innovative technologies and methods, and support long-term follow-up studies. In addition, social policies play a crucial role in ensuring that ASD research results are translated into practical applications and that education, employment, and social services are provided to individuals with ASD. Through the development of inclusive education policies, employment assistance programs, and the provision of integrated social services, policies can help individuals with ASD realize their potential and better integrate into society. At the same time, advances in ASD research also provide a scientific basis for the development of more targeted and effective social policies, helping policymakers understand the needs of individuals with ASD and develop more precise support measures. Thus, there is a close interplay between social policy and ASD research, which together have contributed to the advancement of the understanding of ASD and coping strategies.
Limitations of the current research
Although significant progress has been made in ASD research, a number of key limitations remain. First, the etiology of ASD is extremely complex, involving genetic and environmental factors and their interactions, making it extremely challenging to identify specific etiologies and develop targeted treatment strategies. Second, the heterogeneity of ASD is reflected in the extreme variability of symptoms among patients, which makes it difficult to develop uniform diagnostic criteria and treatment approaches. In addition, most studies have focused on children, and adult patients with ASD have been relatively understudied, which limits the understanding of the full lifespan of ASD. In terms of research methodology, most current ASD research relies on small, short-term studies, which may affect the broad applicability of results and the assessment of long-term effectiveness. In addition, although advances in technology have provided new tools for ASD diagnosis and intervention, the popularization and application of these technologies still face economic and resource constraints. Finally, ASD research is unequal across the globe, with far more research conducted in resource-rich countries and regions than in resource-limited areas. This imbalance limits a comprehensive understanding of ASD in different cultural and social contexts. Therefore, to overcome these limitations, more interdisciplinary, cross-cultural, and long-term research, as well as global collaborations, are needed to deepen the understanding of ASD and improve the quality of life of individuals with ASD.
Perspectives on future research
The outlook for future prevention and treatment of ASD points in a more individualized, integrated, and technology-driven direction. With a deeper understanding of the genetic and environmental factors of ASD, it is expected that more targeted interventions and therapeutic strategies will be developed that will be based on an individual's specific genetic background and pathologic characteristics. The application of precision medicine is expected to improve treatment outcomes, reduce unwanted side effects, and optimize resource allocation. Meanwhile, technological advances, particularly artificial intelligence, machine learning, and virtual reality, are expected to revolutionize the way ASDs are diagnosed, monitored, and treated. These technologies are capable of delivering customized learning and treatment programs that enhance the acceptability and effectiveness of interventions. In addition, interdisciplinary research will be strengthened, and social policies and public health strategies will focus more on early screening and intervention, as well as increasing public awareness and understanding of ASD. Most importantly, the future of ASD prevention and treatment will place greater emphasis on the needs of patients and families, promote social integration and employment of patients, and improve their quality of life. As society's awareness of diversity and inclusion increases, individuals with ASD will receive more support and respect and enjoy fuller opportunities for social participation.
Author information
Authors and affiliations.
Department of Rehabilitation, The Second Affiliated Hospital of Shandong First Medical University, Taian, Shandong, China
Lei Qin, Wenjing Ning & Mengmeng Cui
Department of Intensive Care Medicine, Feicheng People’s Hospital, Taian, Shandong, China
Haijiao Wang
Department of Central Laboratory, The Affiliated Taian City Central Hospital of Qingdao University, Taian, China
You can also search for this author in PubMed Google Scholar
Contributions
LQ, HW and WN wrote the draft of the manuscript. MC and QW revised this manuscript. All the listed authors have made a substantial, direct, and intellectual contribution to the work, and approved its publication.
Corresponding authors
Correspondence to Mengmeng Cui or Qian Wang .
Ethics declarations
Ethical approval and consent to participate.
Not applicable.
Competing interests
The authors declare there are no competing interests.
Additional information
Publisher's note.
Springer Nature remains neutral with regard to jurisdictional claims in published maps and institutional affiliations.
Rights and permissions
Open Access This article is licensed under a Creative Commons Attribution 4.0 International License, which permits use, sharing, adaptation, distribution and reproduction in any medium or format, as long as you give appropriate credit to the original author(s) and the source, provide a link to the Creative Commons licence, and indicate if changes were made. The images or other third party material in this article are included in the article's Creative Commons licence, unless indicated otherwise in a credit line to the material. If material is not included in the article's Creative Commons licence and your intended use is not permitted by statutory regulation or exceeds the permitted use, you will need to obtain permission directly from the copyright holder. To view a copy of this licence, visit http://creativecommons.org/licenses/by/4.0/ . The Creative Commons Public Domain Dedication waiver ( http://creativecommons.org/publicdomain/zero/1.0/ ) applies to the data made available in this article, unless otherwise stated in a credit line to the data.
Reprints and permissions
About this article
Cite this article.
Qin, L., Wang, H., Ning, W. et al. New advances in the diagnosis and treatment of autism spectrum disorders. Eur J Med Res 29 , 322 (2024). https://doi.org/10.1186/s40001-024-01916-2
Download citation
Received : 17 April 2024
Accepted : 01 June 2024
Published : 10 June 2024
DOI : https://doi.org/10.1186/s40001-024-01916-2
Share this article
Anyone you share the following link with will be able to read this content:
Sorry, a shareable link is not currently available for this article.
Provided by the Springer Nature SharedIt content-sharing initiative
- Autism spectrum disorder (ASD)
- Diagnostic methods
- Treatment strategies
- Precision medicine
- Emerging biotechnology
European Journal of Medical Research
ISSN: 2047-783X
- General enquiries: [email protected]
- Neurodevelopmental Disorders
- Developmental Pediatrics
- Developmental Disorders
- Autism Spectrum Disorders
Autism Spectrum Disorder: Review Article
- Medico-Legal Update 20(2):324-329
- 20(2):324-329

- Al Muthanna University

Discover the world's research
- 25+ million members
- 160+ million publication pages
- 2.3+ billion citations
- Norwahidah Mohamad Yazid

- Zainura Binti Idrus

- Doaa Elsayed
- Sergio E. Starkstein

- AM J MED GENET B

- Richard M. Kubina

- J NEUROINFLAMM

- Abraham Reichenberg

- Mark F O'Reilly

- Myriam Suárez

- Judy S. Reilly
- Patricia A Stewart
- Susan L. Hyman
- Brianne Schmidt

- John Bankart
- Howard Meltzer
- Recruit researchers
- Join for free
- Login Email Tip: Most researchers use their institutional email address as their ResearchGate login Password Forgot password? Keep me logged in Log in or Continue with Google Welcome back! Please log in. Email · Hint Tip: Most researchers use their institutional email address as their ResearchGate login Password Forgot password? Keep me logged in Log in or Continue with Google No account? Sign up
Advertisement
Detection of Autism Spectrum Disorder in Children Using Machine Learning Techniques
- Original Research
- Published: 22 July 2021
- Volume 2 , article number 386 , ( 2021 )
Cite this article
- Kaushik Vakadkar ORCID: orcid.org/0000-0002-6844-4519 1 ,
- Diya Purkayastha 1 &
- Deepa Krishnan 2
19k Accesses
68 Citations
1 Altmetric
Explore all metrics
Autism Spectrum Disorder (ASD) is a neurological disorder which might have a lifelong impact on the language learning, speech, cognitive, and social skills of an individual. Its symptoms usually show up in the developmental stages, i.e., within the first two years after birth, and it impacts around 1% of the population globally [ https://www.autism-society.org/whatis/facts-and-statistics/ . Accessed 25 Dec 2019]. ASD is mainly caused by genetics or by environmental factors; however, its conditions can be improved by detecting and treating it at earlier stages. In the current times, clinical standardized tests are the only methods which are being used, to diagnose ASD. This not only requires prolonged diagnostic time but also faces a steep increase in medical costs. To improve the precision and time required for diagnosis, machine learning techniques are being used to complement the conventional methods. We have applied models such as Support Vector Machines (SVM), Random Forest Classifier (RFC), Naïve Bayes (NB), Logistic Regression (LR), and KNN to our dataset and constructed predictive models based on the outcome. The main objective of our paper is to thus determine if the child is susceptible to ASD in its nascent stages, which would help streamline the diagnosis process. Based on our results, Logistic Regression gives the highest accuracy for our selected dataset.
Similar content being viewed by others
Early Detection of Autism Spectrum Disorder in Children Using Supervised Machine Learning
Predicting Autism Spectrum Disorder Using Various Machine Learning Techniques
Opinion on Prediction Algorithms for Identifying Autism Spectrum Disorder
Explore related subjects.
- Artificial Intelligence
Avoid common mistakes on your manuscript.
Introduction
Autism Spectrum Disorder occurs in the developmental stages of an individual and is a serious disorder which can impair the ability to interact or communicate with others. Generally caused by genetics or environmental factors, it impacts the nervous system, as a result of which the overall cognitive, social, emotional, and physical health of the individual is affected [ 8 ]. There is a wide variance in the range as well as the severity of its symptoms. A few of the common symptoms the individual faces are difficulties in communication, especially in social settings, obsessive interests, and mannerisms, which take a repetitive form. To identify ASD, an extensive examination is required. This also includes an extensive evaluation and a variety of assessments by psychologists for children and various certified professionals. Conventional methods of diagnosing include Autism Diagnostic Interview Revised (ADI-R) and Autism Diagnostic Observation Schedule Revised (ADOS-R). However, these are lengthy and cumbersome, taking up a large amount of time as well as effort.
A significant portion of the pediatric population suffers from ASD. In most cases, it can usually be identified in its preliminary stages, but the major bottleneck lies in the subjective and tedious nature of existing diagnosis procedures. As a result, there is a waiting time of at least 13 months from the initial suspicion to the actual diagnosis. The diagnosis takes many hours [ 10 ], and the continuously growing demand for appointments is much greater than the peak capacity of the country’s pediatric clinics [ 20 ].
Detecting and treating Autism Spectrum Disorder in its early stages are extremely crucial as this helps to decrease or alleviate the symptoms to a certain extent, thus improving the overall quality of life for the individual. However, owing to the gaps between initial concern and diagnosis, a lot of valuable time is lost as this disorder remains undetected. Machine Learning methods would not only help to assess the risk for ASD in a quick and accurate manner, but are also essential to streamline the whole diagnosis process and help families access the much-needed therapies faster.
Some of the screening methods used to detect ASD in children are Autism Spectrum Quotient (AQ), Childhood Autism Rating Scale (CARS-2), and Screening Tool for Autism in Toddlers and Young Children (STAT). In our paper, we have used the Q-CHAT-10 [ 2 ] screening method for toddlers.
We have structured our paper as follows: “ Introduction ” section includes the introduction to our project. “ Review of Literature ” section summarizes the literature survey performed. “ Working Model ” and “ Methodology ” section explain the working and methodology of the system we have proposed and its implementation. “ Analysis and Results ” section portrays the inferences and results obtained. Finally, “ Conclusion ” section highlights our conclusions.
Review of Literature
Several studies have made use of machine learning in various ways to improve and speed up the diagnosis of ASD. Duda et al. [ 5 ] applied forward feature selection coupled with under sampling to differentiate between autism and ADHD with the help of a Social Responsiveness Scale containing 65 items. Deshpande et al. [ 4 ] used metrics based on brain activity to predict ASD. Soft computing techniques such as probabilistic reasoning, artificial neural networks (ANN), and classifier combination have also been used [ 15 ]. Many of the studies performed have talked of automated ML models which only depend on characteristics as input features. A few studies relied on data from brain neuroimaging as well. In the ABIDE database, Li et al. [ 14 ], extracted 6 personal characteristics from 851 subjects and performed the implementation of a cross-validation strategy for the training and testing of the ML models. This was used to classify between patients with and without ASD, respectively. Thabtah et al. [ 21 ] proposed a new ML technique called Rules-Machine Learning (RML) that offers users a knowledge base of rules for understanding the underlying reasons behind the classification, in addition to detecting ASD traits. Al Banna et al. [ 1 ] made use of a personalized AI-based system which assists with the monitoring and support of ASD patients, helping them cope with the COVID-19 pandemic.
In this study, we have used five ML models to classify individual subjects as having ASD or No-ASD, by making use of various features, such as age, sex, ethnicity, etc., and evaluated each classifier to determine the best performing model.
To provide a concise view of our literature survey, we have summarized the most relevant papers that we studied, by identifying the key findings and limitations of each paper and listing them down in the form of a table (Table 1 ).
Working Model
Figure 1 demonstrates the general working and flow of our system. We begin by preprocessing the dataset to eliminate missing values and outliers, remove noise, and encode categorical attributes. We also employ feature engineering to choose the most beneficial features out of all the features present in the data set. This reduces data dimensionality to improve speed and efficiency during training. Once the data set has been preprocessed, classification algorithms like Logistic Regression, Naïve Bayes, Support Vector Machine, K-Nearest Neighbors, and Random Forest Classifiers are used to predict the output label (ASD or no ASD). The accuracy of each classifier is observed and compared. Furthermore, metrics like the F1 score and precision-recall values have also been computed for better evaluation of each classifier. If the classifier performs well, then the training accuracy will be higher than its test accuracy. This model can then be deemed to be the best model and hence be used for further training and classification. A brief description of this approach has been discussed in “ Methodology ” section.
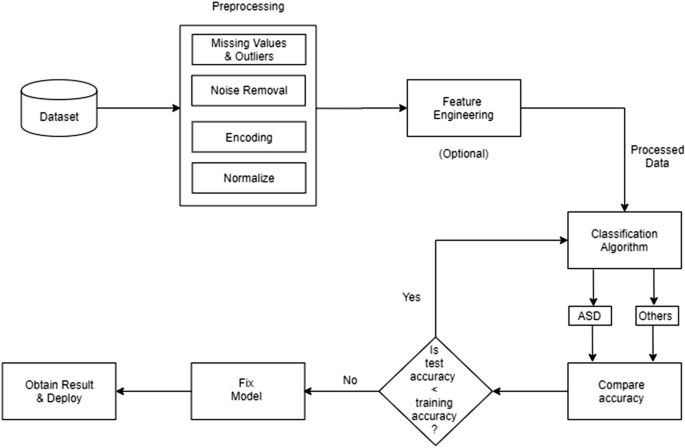
Architecture of proposed system
Methodology
Data preprocessing.
The dataset [ 3 ] that we have used has been compiled by Dr. Fadi Thabtah [ 6 ] and it contains categorical, continuous and binary attributes. Originally, the dataset had 1054 instances along with 18 attributes (including class variable). Since the dataset contained a few non-contributing and categorical attributes, we had to preprocess the data. Preprocessing refers to the transformations applied to a data set before feeding it to the model. It is done to clean raw or noisy data and make it more suited for training and analysis. We removed the non-contributing attributes, namely ‘Case_No’, ‘Who completed the test’, and ‘Qchat-10-Score’.
To deal with the categorical values, we are making use of label encoding. Label Encoding converts the labels into numeric form to make it machine-readable. Repeated labels are assigned the same value as assigned earlier. Four features having 2 classes (Sex, Jaundice, Family_mem_with_ASD, and Class/ASD_Traits) have been selected to be binary label encoded. Label Encoding proves to be ineffective when there are more than 2 classes. For multiclass features, One-Hot Encoding is used to avoid hierarchical ordering by the model. The ‘Ethnicity’ feature which has 11 classes has been one-hot encoded.
Classification Algorithms
We split the dataset into two parts—training set and test set. The training set consisting of 80% of the data (843 samples) will be used to train the classification model. The remaining 20% of the data (211 samples) will be reserved for testing the accuracy and effectiveness of the model on unseen data and will be referred to as the testing data set. This random partitioning of data into training and testing sets helps us determine if our model is overfitting or underfitting. If the model has low training error, but high testing error, then the model is overfitting the data. On the other hand, if the model has high training and testing error, the model is underfitting the data. A good model will neither overfit nor underfit the data.
After having performed data preprocessing (4.1), we applied five classification models, namely Logistic Regression, Naive Bayes, Support Vector Machine, K-Nearest Neighbors, and Random Forest Classifier, and compared the performance of each based on accuracy achieved and F1 score (Table 4 ). A brief description of the classification models used has been given below.
Logistic Regression Logistic Regression (LR)
Logistic Regression’s primary aim is in finding the model with the best fit that describes the relationship between the binomial character of interest and a set of independent variables [ 12 ]. It makes use of a logistic function to find an optimal curve to fit the data points.
Naive Bayes (NB)
Based around conditional probability (Bayes theorem) and counting, the name “naïve” comes from its assumption of conditional independence of all input features [ 13 ]. If this assumption is considered true, the rate at which an NB classifier will converge will be much higher than a discriminative model like logistic regression. Therefore, the amount of training data required would be lesser. The main disadvantage of NB is that it only works well with limited number of features. Moreover, there is a high bias when there is a small amount of data.
Support Vector Machine (SVM)
Commonly used in classification problems, Support Vector Machine is based on the idea of finding the hyperplane that divides a given data set into two classes in the best possible way [ 18 ]. The distance from the hyperplane to the closest training data point is known as the margin. SVM aims to maximize the margin of the training data by finding the most optimal separating hyperplane [ 19 ]. We began our training with a linear RBF kernel and observed it to give good results as compared to a non-linear kernel.
K-Nearest Neighbors (KNN)
The KNN algorithm is based on mainly two ideas: the notion of a distance metric and that points that are close to one another are similar. Let x be the new data point that we wish to predict a label for. The KNN algorithm works by finding the k training data points closest to x using a Euclidean distance metric. KNN algorithm then performs majority voting to determine the label for the new data point x [ 9 ]. In our analysis, lower values of k ( k = 1 to k = 10) gave us the highest accuracy.
Random Forest Classifier (RFC)
Random forest classifier is a flexible algorithm that can be used for classification, regression, and other tasks, as well [ 16 ]. It works by creating multiple decision trees on arbitrary data points. After getting the prediction from each tree, the best solution is selected by voting.
Analysis and Results
Dataset analysis.
The dataset used here is based on the Quantitative Checklist for Autism in Toddlers (Q-CHAT) screening method devised by Baron-Cohen et al. [ 2 ]. A shortened version, Q-CHAT-10, containing a set of 10 questions has been used (Table 2 ). The answers to these questions are mapped to binary values as class type. These values are assigned during the data collection process by means of answering the Q-CHAT-10 questionnaire. The class value “Yes” is assigned if the Q-CHAT-10 score happens to be greater than 3, that is, there are potential ASD traits. Otherwise, class value “No” is assigned, implying no ASD traits.
We plotted several graphs to get different visual perspectives of the dataset. In the first plot (Fig. 2 ), we can see that the number of toddlers who are ASD positive is those who do not have jaundice while birth. The count is over 2 times that of jaundice born toddlers. Thus, we can infer that jaundice born children have a weak link with ASD.
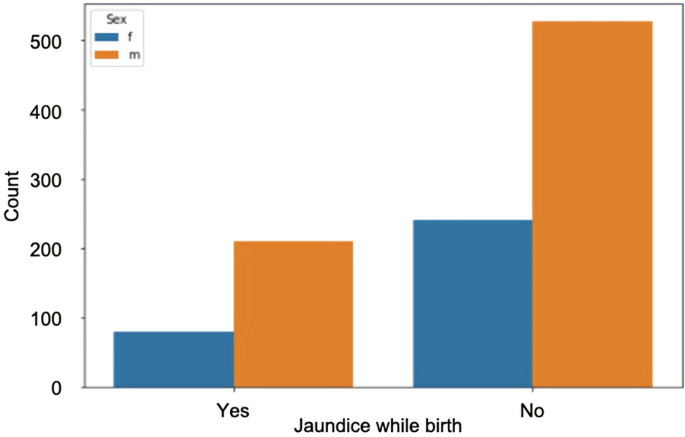
ASD positive toddlers born with jaundice based on gender
For toddlers, most of the ASD positive cases happen to be at are around 36 months of age. The least number of cases were observed between 15 and 20 months of age. From the graph, it is evident that significant signs of autism occur at the age of 3 years (Fig. 3 ). According to Ref. [ 22 ], one out of every 68 children aged between 2 and 3 years has autism.
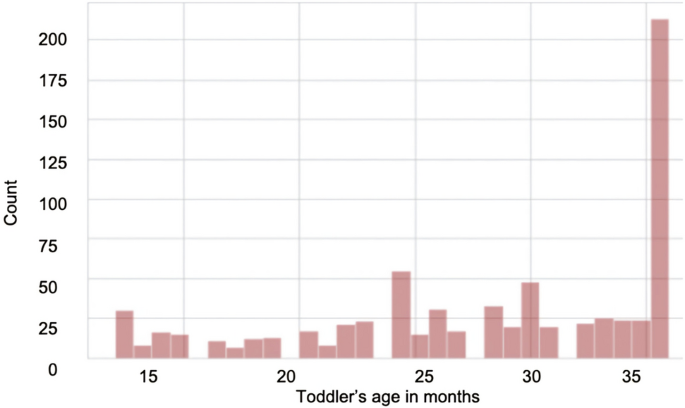
Age distribution of ASD positive
We plotted a gender distribution graph of the ASD traits observed in males and females. It can be concluded that ASD is more prevalent in males than in females as depicted in Fig. 4 .
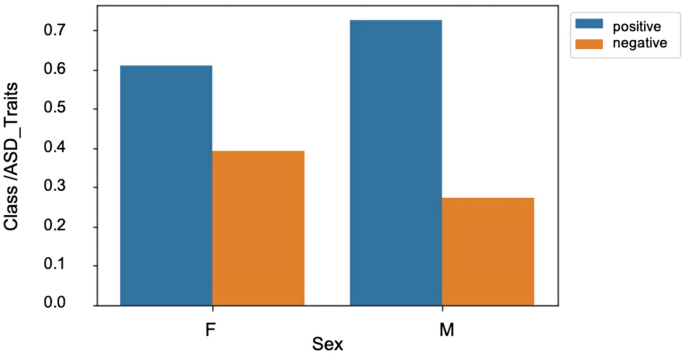
Gender distribution of ASD traits
The ethnicity distribution graph reveals that Native Indian individuals have the highest observed ASD traits (Fig. 5 ).
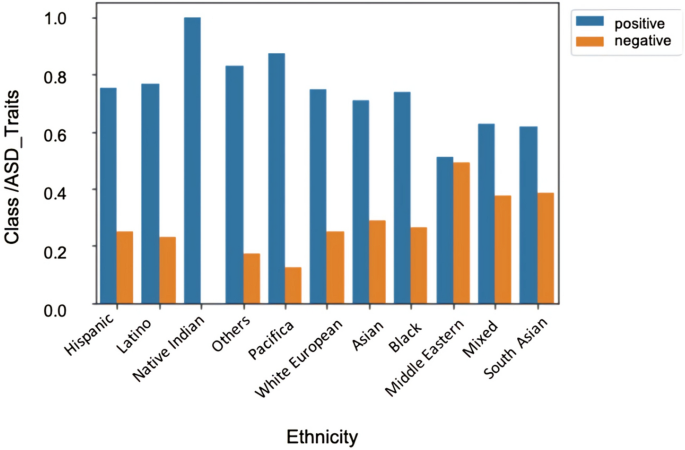
Ethnicity distribution of ASD traits
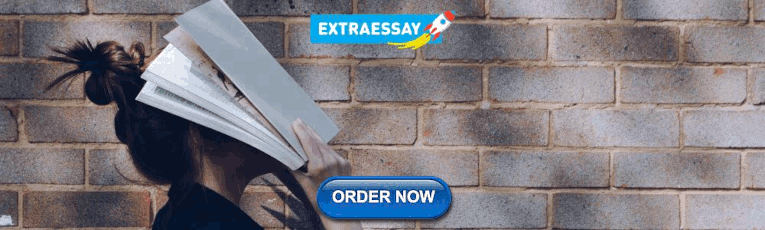
Evaluation Matrix
Usually, in most predictive models, the data points lie in the following four categories:
True positive (TP): The individual has ASD and we predicted correctly that the individual has ASD.
True negative (TN): The individual does not have ASD and we predicted correctly that the individual does not have ASD.
False positive (FP): The individual does not have ASD, but we predicted incorrectly that the individual has ASD. This is known as Type 1 error.
False negative (FN): The individual has ASD, but we predicted incorrectly that the individual does not have ASD. This is known as Type 2 error.
The above four categories when put together in the form of a matrix produce the confusion matrix. The confusion matrix is particularly useful in gauging the performance of a machine learning classification model. The confusion matrix along with its parameters is shown below (Table 3 ).
Comparison of Classification Models
We applied five machine learning models—Logistic Regression (LR), Naïve Bayes (NB), Support Vector Machine (SVM), K-Nearest Neighbors (KNN), and Random Forest Classifier (RFC). For the purpose of evaluating the performance of all these models, we have used the confusion matrix and F1 score. Table 4 shows a comparison of all the classification models we used.
From the values obtained, we can thereby infer that Logistic Regression, giving the highest accuracy, is the best model for our current dataset. Logistic regression performs well when the training data size is small and it is binary in nature. The feature space is split linearly, and it works well even when only a few variables are correlated. However, Naïve Bayes assumes that all features are conditionally independent. Hence, if some of the features are interdependent, the prediction might be inaccurate.
In addition to accuracy, we have also found out the precision and recall values to provide a better insight. Using these values, the F1 score has then been calculated by taking the weighted average (harmonic mean) of the precision and recall values. This score can vary between 0 and 1. The higher the F1 score, the better the model (a score of 1 is considered to be the best)
Precision and Recall Curves
Precision measures how accurate our positive predictions were, i.e., out of all the points predicted to be positive how many of them were actually positive
Recall measures what fraction of the positives our model identified, i.e., out of the points that are labeled positive, how many of them were correctly predicted as positive. Recall is the same as sensitivity
Accuracy can be defined as the probability of the number of correct predictions made by the classifier. In other words, it is the fraction of correct predictions made out of the total number of predictions
A precision-recall curve is generated by creating crisp class labels for probability predictions across a set of thresholds. For each threshold value, the precision and recall values are calculated. A line plot is created for the thresholds in ascending order with recall/precision on the y-axis and threshold on the x-axis. Shown below are the precision and recall curves plotted against threshold for the top three performing models—Logistic Regression (Fig. 6 ), Naïve Bayes (Fig. 7 ), and SVM (Fig. 8 ).
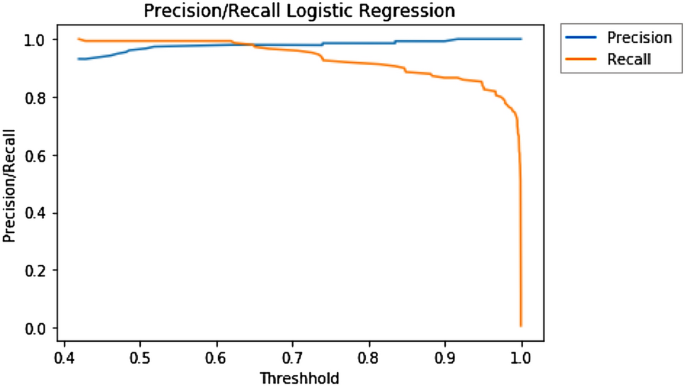
Precision/recall curve for LR
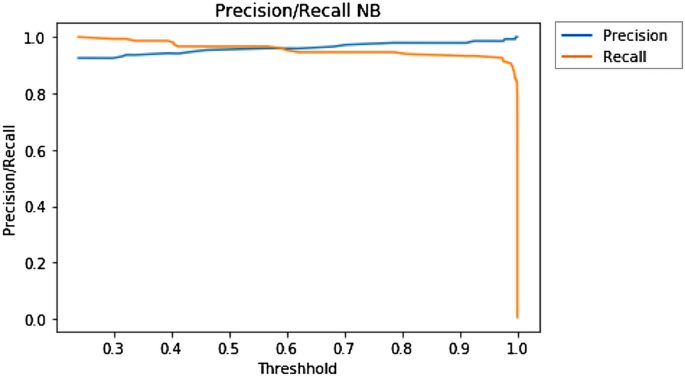
Precision/recall curve for NB
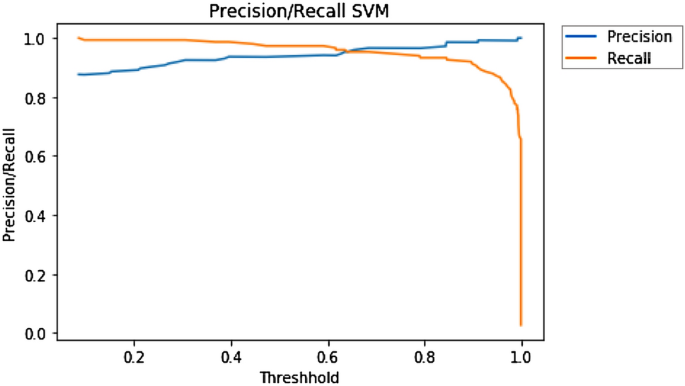
Precision/recall curves for SVM
The assessment of ASD behavioral traits is a time taking process that is only aggravated by overlapping symptomatology. There is currently no diagnostic test that can quickly and accurately detect ASD, or an optimized and thorough screening tool that is explicitly developed to identify the onset of ASD. We have designed an automated ASD prediction model with minimum behavior sets selected from the diagnosis datasets of each. Out of the five models that we applied to our dataset; Logistic Regression was observed to give the highest accuracy.
The primary limitation of this research is the scarce availability of large and open source ASD datasets. To build an accurate model, a large dataset is necessary. The dataset we used here did not have sufficient number of instances. However, our research has provided useful insights in the development of an automated model that can assist medical practitioners in detecting autism in children. In the future, we will be considering using a larger dataset to improve generalization. We also plan to employ deep learning techniques that integrate CNNs and classification to improve robustness and overall performance of the system. All in all, our research has resulted in analyzing various classification models that can accurately detect ASD in children with given attributes based on the child’s behavioral and medical information. The analysis of these classification models can be used by other researchers as a basis for further exploring this dataset or other Autism Spectrum Disorder data sets.
Al Banna MH, Ghosh T, Taher KA, Kaiser MS, Mahmud M. A monitoring system for patients of autism spectrum disorder using artificial intelligence. In: International conference on brain informatics. Cham: Springer; 2020. pp. 251–62.
Baron-Cohen S, Allen J, Gillberg C. Can autism be detected at 18 months? The needle, the haystack, and the CHAT. Br J Psychiatry. 1992;161:839–43.
Article Google Scholar
Dataset: https://www.kaggle.com/fabdelja/autism-screening-for-toddlers . Accessed 1 Oct 2019.
Deshpande G, Libero LE, Sreenivasan KR, Deshpande HD, Kana RK. Identification of neural connectivity signatures of autism using machine learning. Front Hum Neurosci. 2013;7:670.
Duda M, Ma R, Haber N, Wall DP. Use of machine learning for behavioral distinction of autism and ADHD. Transl Psychiatry. 2016;6: e732.
Thabtah, F. An accessible and efficient autism screening method for behavioural data and predictive analyses. Health Informatics Journal 2019;25(4):1739–55. https://doi.org/10.1177/1460458218796636 .
https://www.autism-society.org/what-is/facts-and-statistics/ . Accessed 25 Dec 2019.
https://www.helpguide.org/articles/autism-learning-disabilities/autismspectrumdisorders.htm . Accessed 20 Dec 2019.
“KNN Classification using Scikit-learn”, https://www.datacamp.com/community/tutorials/k-nearest-neighbor-classification-scikit-learn . Accessed 8 Oct 2019.
Kosmicki JA, Sochat V, Duda M, Wall DP. Searching for a minimal set of behaviors for autism detection through feature selection-based machine learning. Transl Psychiatry. 2015. https://doi.org/10.1038/tp.2015.7 .
Li H, Parikh NA, He L. A novel transfer learning approach to enhance deep neural network classification of brain functional connectome. Front Neurosci. 2018. https://doi.org/10.3389/fnins.2018.00491 .
Logistic Regression. https://medium.com/datadriveninvestor/logistic-regression-18afd48779ce . Accessed 7 Oct 2019.
Naive Bayes for Machine Learning. https://machinelearningmastery.com/naive-bayes-for-machine-learning/ . Accessed 8 Oct 2019.
Parikh MN, Li H, He L. Enhancing diagnosis of autism with optimized machine learning models and personal characteristic data. Front Comput Neurosci. 2019. https://doi.org/10.3389/fncom.2019.00009 .
Pratap A, Kanimozhiselvi C. Soft computing models for the predictive grading of childhood Autism—a comparative study. IJSCE. 2014;4:64–7.
Google Scholar
Random Forests(r), Explained. https://www.kdnuggets.com/2017/10/random-forests-explained.html . Accessed 8 Oct 2019.
Sen B, Borle NC, Greiner R, Brown MR. A general prediction model for the detection of ADHD and Autism using structural and functional MRI. PLoS ONE. 2018;13: e0194856.
Support Vector Machine—Introduction to Machine Learning Algorithms. https://towardsdatascience.com/support-vector-machine-introduction-to-machine-learning-algorithms-934a444fca47 . Accessed 7 Oct 2019.
Support vector machines: The linearly separable case, https://nlp.stanford.edu/IR-book/html/htmledition/support-vector-machines-the-linearly-separable-case-1.html . Accessed 8 Oct 2019.
Thabtah F. Machine learning in autistic spectrum disorder behavioral research: A review and ways forward. Inf Health Soc Care. 2017;44:278–97.
Thabtah F, Peebles D. A new machine learning model based on induction of rules for autism detection. Health Inform J. 2020;26(1):264–86.
Towle P, Patrick P. Autism spectrum disorder screening instruments for very young children: A systematic review. New York: Hindawi Publishing Corporation; 2016.
Vaishali R, Sasikala R. A machine learning based approach to classify Autism with optimum behaviour sets. Int J Eng Technol. 2017;7:18.
van den Bekerom B. Using machine learning for detection of autism spectrum disorder. In: 26th Twente Student Conference on IT, Feb 2017.
Download references
Author information
Authors and affiliations.
Computer Engineering, Mukesh Patel School of Technology Management and Engineering, NMIMS University, Mumbai, India
Kaushik Vakadkar & Diya Purkayastha
Computer Engineering Department, Mukesh Patel School of Technology Management and Engineering, NMIMS University, Mumbai, India
Deepa Krishnan
You can also search for this author in PubMed Google Scholar
Corresponding author
Correspondence to Kaushik Vakadkar .
Ethics declarations
Conflict of interest.
On behalf of all authors, the corresponding author states that there is no conflict of interest.
Additional information
Publisher's note.
Springer Nature remains neutral with regard to jurisdictional claims in published maps and institutional affiliations.
This article is part of the topical collection “Advanced Computing and Data Sciences” guest edited by Mayank Singh, Vipin Tyagi, and P.K. Gupta.
Rights and permissions
Reprints and permissions
About this article
Vakadkar, K., Purkayastha, D. & Krishnan, D. Detection of Autism Spectrum Disorder in Children Using Machine Learning Techniques. SN COMPUT. SCI. 2 , 386 (2021). https://doi.org/10.1007/s42979-021-00776-5
Download citation
Received : 24 July 2020
Accepted : 12 July 2021
Published : 22 July 2021
DOI : https://doi.org/10.1007/s42979-021-00776-5
Share this article
Anyone you share the following link with will be able to read this content:
Sorry, a shareable link is not currently available for this article.
Provided by the Springer Nature SharedIt content-sharing initiative
- Autism spectrum disorder
- Machine learning
- Preprocessing
- Random forest
- Logistic regression
- Confusion matrix
- Find a journal
- Publish with us
- Track your research
Thank you for visiting nature.com. You are using a browser version with limited support for CSS. To obtain the best experience, we recommend you use a more up to date browser (or turn off compatibility mode in Internet Explorer). In the meantime, to ensure continued support, we are displaying the site without styles and JavaScript.
- View all journals
- Explore content
- About the journal
- Publish with us
- Sign up for alerts
- Published: 16 January 2020
Autism spectrum disorder
- Catherine Lord 1 ,
- Traolach S. Brugha 2 ,
- Tony Charman 3 ,
- James Cusack 4 ,
- Guillaume Dumas 5 ,
- Thomas Frazier 6 ,
- Emily J. H. Jones 7 ,
- Rebecca M. Jones 8 , 9 ,
- Andrew Pickles 3 ,
- Matthew W. State 10 ,
- Julie Lounds Taylor 11 &
- Jeremy Veenstra-VanderWeele 12
Nature Reviews Disease Primers volume 6 , Article number: 5 ( 2020 ) Cite this article
48k Accesses
719 Citations
423 Altmetric
Metrics details
- Autism spectrum disorders
- Cognitive neuroscience
- Paediatrics
Autism spectrum disorder is a construct used to describe individuals with a specific combination of impairments in social communication and repetitive behaviours, highly restricted interests and/or sensory behaviours beginning early in life. The worldwide prevalence of autism is just under 1%, but estimates are higher in high-income countries. Although gross brain pathology is not characteristic of autism, subtle anatomical and functional differences have been observed in post-mortem, neuroimaging and electrophysiological studies. Initially, it was hoped that accurate measurement of behavioural phenotypes would lead to specific genetic subtypes, but genetic findings have mainly applied to heterogeneous groups that are not specific to autism. Psychosocial interventions in children can improve specific behaviours, such as joint attention, language and social engagement, that may affect further development and could reduce symptom severity. However, further research is necessary to identify the long-term needs of people with autism, and treatments and the mechanisms behind them that could result in improved independence and quality of life over time. Families are often the major source of support for people with autism throughout much of life and need to be considered, along with the perspectives of autistic individuals, in both research and practice.
This is a preview of subscription content, access via your institution
Access options
Access Nature and 54 other Nature Portfolio journals
Get Nature+, our best-value online-access subscription
24,99 € / 30 days
cancel any time
Subscribe to this journal
Receive 1 digital issues and online access to articles
111,21 € per year
only 111,21 € per issue
Buy this article
- Purchase on SpringerLink
- Instant access to full article PDF
Prices may be subject to local taxes which are calculated during checkout
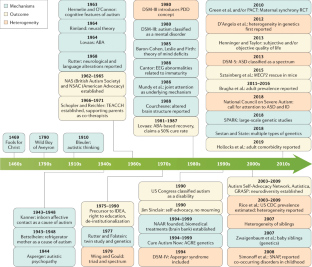
Similar content being viewed by others
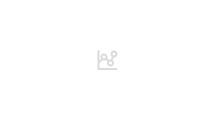
Neurogenetic disorders across the lifespan: from aberrant development to degeneration
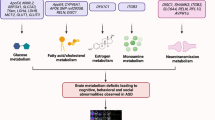
Neuroimaging genetics approaches to identify new biomarkers for the early diagnosis of autism spectrum disorder
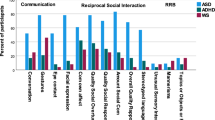
Symptoms of autism in Williams syndrome: a transdiagnostic approach
Lord, C. et al. Autism from 2 to 9 years of age. Arch. Gen. Psychiatry 63 , 694–701 (2006). This paper establishes that autism is a stable diagnosis (as a spectrum) beginning at least by 2 years of age. The paper also establishes parent interview and clinician observation as predictive of autism at 9 years of age. Finally, it is the first paper that shows that the specific DSM-IV-TR diagnoses is unstable across childhood but that the instability is almost all shifting across categories not outside the spectrum.
Article PubMed Google Scholar
Risi, S. et al. Combining information from multiple sources in the diagnosis of autism spectrum disorders. J. Am. Acad. Child Adolesc. Psychiatry 45 , 1094–1103 (2006).
Loomes, R., Hull, L. & Mandy, W. P. L. What is the male-to-female ratio in autism spectrum disorder? A systematic review and meta-analysis. J. Am. Acad. Child Adolesc. Psychiatry 56 , 466–474 (2017).
Brugha, T. S. et al. Epidemiology of autism in adults across age groups and ability levels. Br. J. Psychiatry 209 , 498–503 (2016). This paper uses active case-finding to provide representative estimates of the prevalence of autism and demonstrated that rates of autism in men and women are equivalent in adults with moderate-to-profound intellectual disability.
Brugha, T., Bankart, J., McManus, S. & Gullon-Scott, F. CDC autism rate: misplaced reliance on passive sampling? Lancet 392 , 732–733 (2018).
Baxter, A. J. et al. The epidemiology and global burden of autism spectrum disorders. Psychol. Med. 45 , 601–613 (2015).
Article CAS PubMed Google Scholar
Elsabbagh, M. et al. Global prevalence of autism and other pervasive developmental disorders. Autism Res. 5 , 160–179 (2012).
Article PubMed PubMed Central Google Scholar
Magnusson, C. et al. Migration and autism spectrum disorder: population-based study. Br. J. Psychiatry 201 , 109–115 (2012).
Goodman, R. & Richards, H. Child and adolescent psychiatric presentations of second-generation Afro-Caribbeans in Britain. Br. J. Psychiatry 167 , 362–369 (1995).
Dyches, T. T., Wilder, L. K., Sudweeks, R. R., Obiakor, F. E. & Algozzine, B. Multicultural issues in autism. J. Autism Dev. Disord. 34 , 211–222 (2004).
Keen, D. V., Reid, F. D. & Arnone, D. Autism, ethnicity and maternal immigration. Br. J. Psychiatry 196 , 274–281 (2010).
McManus, S., Bebbington, P., Jenkins, R. & Brugha, T. Adult Psychiatric Morbidity Survey: mental health and wellbeing in England, 2014. NHS https://digital.nhs.uk/data-and-information/publications/statistical/adult-psychiatric-morbidity-survey/adult-psychiatric-morbidity-survey-survey-of-mental-health-and-wellbeing-england-2014 (2016).
GBD 2017 Disease and Injury Incidence and Prevalence Collaborators. Global, regional, and national incidence, prevalence, and years lived with disability for 354 diseases and injuries for 195 countries and territories, 1990–2017: a systematic analysis for the Global Burden of Disease Study 2017. Lancet 392 , 1789–1858 (2018).
Article Google Scholar
Marcheselli, F. et al. Mental health of children and young people in England, 2017. NHS https://digital.nhs.uk/data-and-information/publications/statistical/mental-health-of-children-and-young-people-in-england/2017/2017 (2018).
Brugha, T. C. et al. Autism Spectrum Disorder, Adult Psychiatric Morbidity Survey 2014. (2014).
Lundstrom, S., Reichenberg, A., Anckarsater, H., Lichtenstein, P. & Gillberg, C. Autism phenotype versus registered diagnosis in Swedish children: prevalence trends over 10 years in general population samples. BMJ 350 , h1961 (2015).
Tromans, S., Chester, V., Kiani, R., Alexander, R. & Brugha, T. The prevalence of autism spectrum disorders in adult psychiatric inpatients: a systematic review. Clin. Pract. Epidemiol. Ment. Health 14 , 177–187 (2018).
Modabbernia, A., Velthorst, E. & Reichenberg, A. Environmental risk factors for autism: an evidence-based review of systematic reviews and meta-analyses. Mol. Autism 8 , 13 (2017).
Article PubMed PubMed Central CAS Google Scholar
Wu, S. et al. Advanced parental age and autism risk in children: a systematic review and meta-analysis. Acta Psychiatr. Scand. 135 , 29–41 (2017).
Taylor, L. E., Swerdfeger, A. L. & Eslick, G. D. Vaccines are not associated with autism: an evidence-based meta-analysis of case-control and cohort studies. Vaccine 32 , 3623–3629 (2014).
Lai, M.-C., Lombardo, M. V. & Baron-Cohen, S. Autism. Lancet 383 , 896–910 (2014).
Velikonja, T., Fett, A.-K. & Velthorst, E. Patterns of nonsocial and social cognitive functioning in adults with autism spectrum disorder: a systematic review and meta-analysis. JAMA Psychiatry 76 , 135–151 (2019).
McNally Keehn, R. H., Lincoln, A. J., Brown, M. Z. & Chavira, D. A. The coping cat program for children with anxiety and autism spectrum disorder: a pilot randomized controlled trial. J. Autism Dev. Disord. 43 , 57–67 (2013).
Jones, E. J. H., Gliga, T., Bedford, R., Charman, T. & Johnson, M. H. Developmental pathways to autism: a review of prospective studies of infants at risk. Neurosci. Biobehav. Rev. 39 , 1–33 (2014).
Ozonoff, S. et al. Recurrence risk for autism spectrum disorders: a baby siblings research consortium study. Pediatrics 128 , e488–e495 (2011).
PubMed PubMed Central Google Scholar
Jones, R. M. & Lord, C. Diagnosing autism in neurobiological research studies. Behav. Brain Res. 251 , 113–124 (2013).
Johnson, M. H. Autism: demise of the innate social orienting hypothesis. Curr. Biol. 24 , R30–R31 (2014).
Johnson, M. H., Jones, E. J. H. & Gliga, T. Brain adaptation and alternative developmental trajectories. Dev. Psychopathol. 27 , 425–442 (2015).
The Lancet Psychiatry. Of mice and mental health. Lancet Psychiatry 6 , 877 (2019).
Nelson, C. A. et al. An integrative, multidisciplinary approach to the study of brain-behavior relations in the context of typical and atypical development. Dev. Psychopathol. 14 , 499–520 (2002).
Cross-Disorder Group of the Psychiatric Genomics Consortium et al. Genetic relationship between five psychiatric disorders estimated from genome-wide SNPs. Nat. Genet. 45 , 984–994 (2013).
Article CAS Google Scholar
Gaugler, T. et al. Most genetic risk for autism resides with common variation. Nat. Genet. 46 , 881–885 (2014).
Article CAS PubMed PubMed Central Google Scholar
Wang, K., Gaitsch, H., Poon, H., Cox, N. J. & Rzhetsky, A. Classification of common human diseases derived from shared genetic and environmental determinants. Nat. Genet. 49 , 1319–1325 (2017).
Sanders, S. J. et al. Insights into autism spectrum disorder genomic architecture and biology from 71 risk loci. Neuron 87 , 1215–1233 (2015).
Satterstrom, F. K. et al. Large-scale exome sequencing study implicates both developmental and functional changes in the neurobiology of autism. Preprint at https://doi.org/10.1101/484113 (2019).
Sanders, S. J. et al. De novo mutations revealed by whole-exome sequencing are strongly associated with autism. Nature 485 , 237–241 (2012).
Neale, B. M. et al. Patterns and rates of exonic de novo mutations in autism spectrum disorders. Nature 485 , 242–245 (2012).
O’Roak, B. J. et al. Sporadic autism exomes reveal a highly interconnected protein network of de novo mutations. Nature 485 , 246–250 (2012).
Sanders, S. J. et al. Multiple recurrent de novo CNVs, including duplications of the 7q11.23 Williams syndrome region, are strongly associated with autism. Neuron 70 , 863–885 (2011).
Levy, D. et al. Rare de novo and transmitted copy-number variation in autistic spectrum disorders. Neuron 70 , 886–897 (2011).
Sebat, J. et al. Strong association of de novo copy number mutations with autism. Science 316 , 445–449 (2007). This paper is the first to focus explicitly on simplex autism and show the importance of de novo CNVs in simplex cases, versus familial cases, versus controls.
Grove, J. et al. Identification of common genetic risk variants for autism spectrum disorder. Nat. Genet. 51 , 431–444 (2019).
Willsey, J. et al. De novo coding variants are strongly associated with Tourette syndrome. Eur. Neuropsychopharmacol. 29 , S737 (2019).
Epi4K Consortium. Epi4K: gene discovery in 4,000 genomes. Epilepsia 53 , 1457–1467 (2012).
Jamain, S. et al. Mutations of the X-linked genes encoding neuroligins NLGN3 and NLGN4 are associated with autism. Nat. Genet. 34 , 27–29 (2003). This is the first paper to show a de novo loss-of-function mutation in a synaptic gene associated with non-syndromic autism and was a harbinger for many of the findings that came after.
Iossifov, I. et al. The contribution of de novo coding mutations to autism spectrum disorder. Nature 515 , 216–221 (2014).
De Rubeis, S. et al. Synaptic, transcriptional and chromatin genes disrupted in autism. Nature 515 , 209–215 (2014).
Sestan, N. & State, M. W. Lost in translation: traversing the complex path from genomics to therapeutics in autism spectrum disorder. Neuron 100 , 406–423 (2018).
State, M. W. & Sestan, N. The emerging biology of autism spectrum disorders. Science 337 , 1301–1303 (2012).
Schizophrenia Working Group of the Psychiatric Genomics Consortium. Biological insights from 108 schizophrenia-associated genetic loci. Nature 511 , 421–427 (2014).
Article PubMed Central CAS Google Scholar
Devlin, B. & Scherer, S. W. Genetic architecture in autism spectrum disorder. Curr. Opin. Genet. Dev. 22 , 229–237 (2012).
de la Torre-Ubieta, L., Won, H., Stein, J. L. & Geschwind, D. H. Advancing the understanding of autism disease mechanisms through genetics. Nat. Med. 22 , 345–361 (2016).
SFARI Gene Website. https://gene.sfari.org/ (2019).
Parikshak, N. N. et al. Integrative functional genomic analyses implicate specific molecular pathways and circuits in autism. Cell 155 , 1008–1021 (2013).
Ben-David, E. & Shifman, S. Combined analysis of exome sequencing points toward a major role for transcription regulation during brain development in autism. Mol. Psychiatry 18 , 1054–1056 (2013).
Willsey, A. J. et al. Coexpression networks implicate human midfetal deep cortical projection neurons in the pathogenesis of autism. Cell 155 , 997–1007 (2013).
Pinto, D. et al. Functional impact of global rare copy number variation in autism spectrum disorders. Nature 466 , 368–372 (2010).
Gilman, S. R. et al. Rare de novo variants associated with autism implicate a large functional network of genes involved in formation and function of synapses. Neuron 70 , 898–907 (2011).
Fuccillo, M. V. Striatal circuits as a common node for autism pathophysiology. Front. Neurosci. 10 , 27 (2016).
Velmeshev, D. et al. Single-cell genomics identifies cell type-specific molecular changes in autism. Science 364 , 685–689 (2019).
Mendell, J. R. et al. Single-dose gene-replacement therapy for spinal muscular atrophy. N. Engl. J. Med. 377 , 1713–1722 (2017).
Mercuri, E. et al. Nusinersen versus sham control in later-onset spinal muscular atrophy. N. Engl. J. Med. 378 , 625–635 (2018).
Matharu, N. et al. CRISPR-mediated activation of a promoter or enhancer rescues obesity caused by haploinsufficiency. Science 363 , eaau0629 (2019).
Abudayyeh, O. O. et al. RNA targeting with CRISPR–cas13. Nature 550 , 280–284 (2017).
Power, J. D. et al. Customized head molds reduce motion during resting state fMRI scans. NeuroImage 189 , 141–149 (2019).
Solso, S. et al. Diffusion tensor imaging provides evidence of possible axonal overconnectivity in frontal lobes in autism spectrum disorder toddlers. Biol. Psychiatry 79 , 676–684 (2016).
Clements, C. C. et al. Evaluation of the social motivation hypothesis of autism: a systematic review and meta-analysis. JAMA Psychiatry 75 , 797–808 (2018).
Ecker, C. Brain anatomy and its relationship to behavior in adults with autism spectrum disorder: a multicenter magnetic resonance imaging study. Arch. Gen. Psychiatry 69 , 195–209 (2012).
Langen, M. et al. Changes in the development of striatum are involved in repetitive behavior in autism. Biol. Psychiatry 76 , 405–411 (2014).
Elsabbagh, M. & Johnson, M. H. Autism and the social brain: the first-year puzzle. Biol. Psychiatry 80 , 94–99 (2016).
Courchesne, E. et al. Unusual brain growth patterns in early life in patients with autistic disorder: an MRI study. Neurology 57 , 245–254 (2001).
Hazlett, H. C. et al. Magnetic resonance imaging and head circumference study of brain size in autism: birth through age 2 years. Arch. Gen. Psychiatry 62 , 1366–1376 (2005).
Wolff, J. J. et al. Differences in white matter fiber tract development present from 6 to 24 months in infants with autism. Am. J. Psychiatry 169 , 589–600 (2012).
Hazlett, H. C. et al. Early brain development in infants at high risk for autism spectrum disorder. Nature 542 , 348–351 (2017). This seminal paper, through careful recruitment and methodology, was the first to show significant early differences that may contribute to our understanding of developmental features in neural structure and circuits .
Wolff, J. J. et al. Neural circuitry at age 6 months associated with later repetitive behavior and sensory responsiveness in autism. Mol. Autism 8 , 8 (2017).
Emerson, R. W. et al. Functional neuroimaging of high-risk 6-month-old infants predicts a diagnosis of autism at 24 months of age. Sci. Transl. Med. 9 , eaag2882 (2017).
Smith, E. et al. Cortical thickness change in autism during early childhood: CT in early childhood ASD. Hum. Brain Mapp. 37 , 2616–2629 (2016).
Uddin, L. Q., Dajani, D. R., Voorhies, W., Bednarz, H. & Kana, R. K. Progress and roadblocks in the search for brain-based biomarkers of autism and attention-deficit/hyperactivity disorder. Transl. Psychiatry 7 , e1218 (2017).
Herringshaw, A. J., Ammons, C. J., DeRamus, T. P. & Kana, R. K. Hemispheric differences in language processing in autism spectrum disorders: a meta-analysis of neuroimaging studies. Autism Res. 9 , 1046–1057 (2016).
He, Y., Byrge, L. & Kennedy, D. P. Non-replication of functional connectivity differences in autism spectrum disorder across multiple sites and denoising strategies. Preprint at https://doi.org/10.1101/640797 (2019).
Lawrence, K. E., Hernandez, L. M., Bookheimer, S. Y. & Dapretto, M. Atypical longitudinal development of functional connectivity in adolescents with autism spectrum disorder. Autism Res. 12 , 53–65 (2019).
Plitt, M., Barnes, K. A., Wallace, G. L., Kenworthy, L. & Martin, A. Resting-state functional connectivity predicts longitudinal change in autistic traits and adaptive functioning in autism. Proc. Natl Acad. Sci. USA 112 , E6699–E6706 (2015).
Di Martino, A. et al. The autism brain imaging data exchange: towards a large-scale evaluation of the intrinsic brain architecture in autism. Mol. Psychiatry 19 , 659–667 (2014).
Doyle-Thomas, K. A. R. et al. Atypical functional brain connectivity during rest in autism spectrum disorders. Ann. Neurol. 77 , 866–876 (2015).
Supekar, K. et al. Brain hyperconnectivity in children with autism and its links to social deficits. Cell Rep. 5 , 738–747 (2013).
Dajani, D. R. & Uddin, L. Q. Local brain connectivity across development in autism spectrum disorder: a cross-sectional investigation. Autism Res. 9 , 43–54 (2016).
Hull, J. V. et al. Resting-state functional connectivity in autism spectrum disorders: a review. Front. Psychiatry 7 , 205 (2017).
Lombardo, M. V. et al. Different functional neural substrates for good and poor language outcome in autism. Neuron 86 , 567–577 (2015).
Carlisi, C. O. et al. Disorder-specific and shared brain abnormalities during vigilance in autism and obsessive-compulsive disorder. Biol. Psychiatry Cogn. Neurosci. Neuroimaging 2 , 644–654 (2017).
Alaerts, K., Swinnen, S. P. & Wenderoth, N. Sex differences in autism: a resting-state fMRI investigation of functional brain connectivity in males and females. Soc. Cogn. Affect. Neurosci. 11 , 1002–1016 (2016).
Kirkovski, M., Enticott, P. G., Hughes, M. E., Rossell, S. L. & Fitzgerald, P. B. Atypical neural activity in males but not females with autism spectrum disorder. J. Autism Dev. Disord. 46 , 954–963 (2016).
Venkataraman, A. et al. Pivotal response treatment prompts a functional rewiring of the brain among individuals with autism spectrum disorder. NeuroReport 27 , 1081–1085 (2016).
Levisohn, P. M. The autism-epilepsy connection. Epilepsia 48 , 33–35 (2007).
Cantor, D. S., Thatcher, R. W., Hrybyk, M. & Kaye, H. Computerized EEG analyses of autistic children. J. Autism Dev. Disord. 16 , 169–187 (1986).
Lefebvre, A. et al. Alpha waves as a neuromarker of autism spectrum disorder: the challenge of reproducibility and heterogeneity. Front. Neurosci. 12 , 662 (2018).
Tierney, A. L., Gabard-Durnam, L., Vogel-Farley, V., Tager-Flusberg, H. & Nelson, C. A. Developmental trajectories of resting EEG power: an endophenotype of autism spectrum disorder. PLOS ONE 7 , e39127 (2012).
Oberman, L. M. et al. EEG evidence for mirror neuron dysfunction in autism spectrum disorders. Cogn. Brain Res. 24 , 190–198 (2005).
Fan, Y.-T., Decety, J., Yang, C.-Y., Liu, J.-L. & Cheng, Y. Unbroken mirror neurons in autism spectrum disorders. J. Child Psychol. Psychiatry 51 , 981–988 (2010).
Southgate, V. & Hamilton, A. F. Unbroken mirrors: challenging a theory of autism. Trends Cogn. Sci. 12 , 225–229 (2008).
Bernier, R., Aaronson, B. & McPartland, J. The role of imitation in the observed heterogeneity in EEG mu rhythm in autism and typical development. Brain Cogn. 82 , 69–75 (2013).
Raymaekers, R., Wiersema, J. R. & Roeyers, H. EEG study of the mirror neuron system in children with high functioning autism. Brain Res. 1304 , 113–121 (2009).
Dumas, G., Soussignan, R., Hugueville, L., Martinerie, J. & Nadel, J. Revisiting mu suppression in autism spectrum disorder. Brain Res. 1585 , 108–119 (2014). This paper replicates the mu suppression deficits in autism during action observation but questions, through high-density spectral analyses and source reconstruction, its previously drawn relation to the mirror neuron system.
Marco, E. J., Hinkley, L. B. N., Hill, S. S. & Nagarajan, S. S. Sensory processing in autism: a review of neurophysiologic findings. Pediatr. Res. 69 , 48R–54R (2011).
Schwartz, S., Shinn-Cunningham, B. & Tager-Flusberg, H. Meta-analysis and systematic review of the literature characterizing auditory mismatch negativity in individuals with autism. Neurosci. Biobehav. Rev. 87 , 106–117 (2018).
Kang, E. et al. Atypicality of the N170 event-related potential in autism spectrum disorder: a meta-analysis. Biol. Psychiatry Cogn. Neurosci. Neuroimaging 3 , 657–666 (2018).
Bonnet-Brilhault, F. et al. GABA/glutamate synaptic pathways targeted by integrative genomic and electrophysiological explorations distinguish autism from intellectual disability. Mol. Psychiatry 21 , 411–418 (2016).
Schilbach, L. Towards a second-person neuropsychiatry. Phil. Trans. R. Soc. B 371 , 20150081 (2016). This review supports that psychiatric disorders are more commonly characterized by impairments of social interaction rather than social observation, and advocates for an interactive turn in neuropsychiatry .
Barraza, P. et al. Implementing EEG hyperscanning setups. MethodsX 6 , 428–436 (2019).
Dumas, G., de Guzman, G. C., Tognoli, E. & Kelso, J. A. The human dynamic clamp as a paradigm for social interaction. Proc. Natl Acad. Sci. USA 111 , E3726–E3734 (2014).
Jones, E. J. H. et al. Reduced engagement with social stimuli in 6-month-old infants with later autism spectrum disorder: a longitudinal prospective study of infants at high familial risk. J. Neurodev. Disord. 8 , 7 (2016).
Ciarrusta, J. et al. Social brain functional maturation in newborn infants with and without a family history of autism spectrum disorder. JAMA Netw. Open 2 , e191868 (2019).
Levin, A. R., Varcin, K. J., O’Leary, H. M., Tager-Flusberg, H. & Nelson, C. A. EEG power at 3 months in infants at high familial risk for autism. J. Neurodev. Disord. 9 , 34 (2017).
Kolesnik, A. et al. Increased cortical reactivity to repeated tones at 8 months in infants with later ASD. Transl. Psychiatry 9 , 46 (2019).
Rippon, G., Brock, J., Brown, C. & Boucher, J. Disordered connectivity in the autistic brain: challenges for the ‘new psychophysiology’. Int. J. Psychophysiol. 63 , 164–172 (2007).
Rosenberg, A., Patterson, J. S. & Angelaki, D. E. A computational perspective on autism. Proc. Natl Acad. Sci. USA 112 , 9158–9165 (2015).
Masuda, F. et al. Motor cortex excitability and inhibitory imbalance in autism spectrum disorder assessed with transcranial magnetic stimulation: a systematic review. Transl. Psychiatry 9 , 110 (2019).
O’Reilly, C., Lewis, J. D. & Elsabbagh, M. Is functional brain connectivity atypical in autism? A systematic review of EEG and MEG studies. PLOS ONE 12 , e0175870 (2017).
Khan, S. et al. Somatosensory cortex functional connectivity abnormalities in autism show opposite trends, depending on direction and spatial scale. Brain 138 , 1394–1409 (2015).
Chen, H., Nomi, J. S., Uddin, L. Q., Duan, X. & Chen, H. Intrinsic functional connectivity variance and state-specific under-connectivity in autism. Hum. Brain Mapp. 38 , 5740–5755 (2017).
Catarino, A., Churches, O., Baron-Cohen, S., Andrade, A. & Ring, H. Atypical EEG complexity in autism spectrum conditions: a multiscale entropy analysis. Clin. Neurophysiol. 122 , 2375–2383 (2011).
Engemann, D. A. et al. Robust EEG-based cross-site and cross-protocol classification of states of consciousness. Brain 141 , 3179–3192 (2018).
Open Science Collaboration. Psychology. Estimating the reproducibility of psychological science. Science 349 , aac4716 (2015).
Lord, C. et al. Autism diagnostic observation schedule: ADOS-2 (Western Psychological Services, 2012).
Regier, D. A. et al. DSM-5 field trials in the United States and Canada, part II: test-retest reliability of selected categorical diagnoses. Am. J. Psychiatry 170 , 59–70 (2013).
American Psychiatric Association. Diagnostic and Statistical Manual of Mental Disorders , 5th Edn (American Psychiatric Association, 2013).
World Health Organization. International classification of diseases for mortality and morbidity statistics (11th Revision). https://icd.who.int/browse11/l-m/en (WHO, 2018).
Constantino, J. N. & Charman, T. Diagnosis of autism spectrum disorder: reconciling the syndrome, its diverse origins, and variation in expression. Lancet Neurol. 15 , 279–291 (2016).
Lord, C. A multisite study of the clinical diagnosis of different autism spectrum disorders. Arch. Gen. Psychiatry 69 , 306–313 (2012).
Miller, J. N. & Ozonoff, S. The external validity of Asperger disorder: lack of evidence from the domain of neuropsychology. J. Abnorm. Psychol. 109 , 227–238 (2000).
American Psychiatric Association. Diagnostic and Statistical Manual of Mental Disorders , Fourth Edn (American Psychiatric Association, 1994).
Green, D., Chandler, S., Charman, T., Simonoff, E. & Baird, G. Brief report: DSM-5 sensory behaviours in children with and without an autism spectrum disorder. J. Autism Dev. Disord. 46 , 3597–3606 (2016).
Ozonoff, S. et al. Diagnosis of autism spectrum disorder after age 5 in children evaluated longitudinally since infancy. J. Am. Acad. Child Adolesc. Psychiatry 57 , 849–857.e2 (2018).
Russell, G., Steer, C. & Golding, J. Social and demographic factors that influence the diagnosis of autistic spectrum disorders. Soc. Psychiatry Psychiatr. Epidemiol. 46 , 1283–1293 (2011).
Charman, T. & Gotham, K. Measurement issues: screening and diagnostic instruments for autism spectrum disorders—lessons from research and practice. Child Adolesc. Ment. Health 18 , 52–63 (2013).
Ashwood, K. L., Buitelaar, J., Murphy, D., Spooren, W. & Charman, T. European clinical network: autism spectrum disorder assessments and patient characterisation. Eur. Child Adolesc. Psychiatry 24 , 985–995 (2015).
Rutter, M., LeCouteur, A. & Lord, C. Autism Diagnostic Interview-Revised (ADI-R). (Western Psychological Services, 2003).
Durkin, M. S. et al. Autism screening and diagnosis in low resource settings: challenges and opportunities to enhance research and services worldwide. Autism Res. 8 , 473–476 (2015). This position paper highlights the challenges to translating knowledge on better awareness, understanding, identification and diagnosis (and then treatments) from the past two decades of clinical research in high-income countries into low-income and middle-income countries.
Baird, G. et al. Prevalence of disorders of the autism spectrum in a population cohort of children in South Thames: the Special Needs and Autism Project (SNAP). Lancet 368 , 210–215 (2006).
Luyster, R. et al. The autism diagnostic observation schedule — toddler module: a new module of a standardized diagnostic measure for autism spectrum disorders. J. Autism Dev. Disord. 39 , 1305–1320 (2009).
de Vries, P. J. Thinking globally to meet local needs: autism spectrum disorders in Africa and other low-resource environments. Curr. Opin. Neurol. 29 , 130–136 (2016).
Article PubMed CAS Google Scholar
Georgiades, S., Bishop, S. L. & Frazier, T. Editorial perspective: longitudinal research in autism—introducing the concept of ‘chronogeneity’. J. Child Psychol. Psychiatry 58 , 634–636 (2017).
Fountain, C., Winter, A. S. & Bearman, P. S. Six developmental trajectories characterize children with autism. Pediatrics 129 , e1112–e1120 (2012).
Kim, S. H. et al. Variability in autism symptom trajectories using repeated observations from 14 to 36 months of age. J. Am. Acad. Child Adolesc. Psychiatry 57 , 837–848.e2 (2018).
Bussu, G. et al. Latent trajectories of adaptive behaviour in infants at high and low familial risk for autism spectrum disorder. Mol. Autism 10 , 13 (2019).
Zerbi, V. et al. Dysfunctional autism risk genes cause circuit-specific connectivity deficits with distinct developmental trajectories. Cereb. Cortex 28 , 2495–2506 (2018).
Fein, D. et al. Optimal outcome in individuals with a history of autism. J. Child Psychol. Psychiatry 54 , 195–205 (2013).
Anderson, D. K., Liang, J. W. & Lord, C. Predicting young adult outcome among more and less cognitively able individuals with autism spectrum disorders. J. Child Psychol. Psychiatry 55 , 485–494 (2014).
Chlebowski, C., Robins, D. L., Barton, M. L. & Fein, D. Large-scale use of the modified checklist for autism in low-risk toddlers. Pediatrics 131 , e1121–e1127 (2013).
Stenberg, N. et al. Identifying children with autism spectrum disorder at 18 months in a general population sample. Paediatr. Perinat. Epidemiol. 28 , 255–262 (2014).
Pierce, K., Courchesne, E. & Bacon, E. To screen or not to screen universally for autism is not the question: why the task force got it wrong. J. Pediatr. 176 , 182–194 (2016).
Siu, A. L. et al. Screening for autism spectrum disorder in young children: US Preventive Services Task Force recommendation statement. JAMA 315 , 691–696 (2016).
Øien, R. A. et al. Clinical features of children with autism who passed 18-month screening. Pediatrics 141 , e20173596 (2018).
Sánchez-García, A. B., Galindo-Villardón, P., Nieto-Librero, A. B., Martín-Rodero, H. & Robins, D. L. Toddler screening for autism spectrum disorder: a meta-analysis of diagnostic accuracy. J. Autism Dev. Disord. 49 , 1837–1852 (2019).
Marlow, M., Servili, C. & Tomlinson, M. A review of screening tools for the identification of autism spectrum disorders and developmental delay in infants and young children: recommendations for use in low- and middle-income countries. Autism Res. 12 , 176–199 (2019).
Raza, S. et al. Brief report: evaluation of the short quantitative checklist for autism in toddlers (Q-CHAT-10) as a brief screen for autism spectrum disorder in a high-risk sibling cohort. J. Autism Dev. Disord. 49 , 2210–2218 (2019).
Charman, T. et al. Testing two screening instruments for autism spectrum disorder in UK community child health services. Dev. Med. Child Neurol. 58 , 369–375 (2016).
Brett, D., Warnell, F., McConachie, H. & Parr, J. R. Factors affecting age at ASD diagnosis in UK: no evidence that diagnosis age has decreased between 2004 and 2014. J. Autism Dev. Disord. 46 , 1974–1984 (2016).
Zuckerman, K. E., Lindly, O. J. & Sinche, B. K. Parental concerns, provider response, and timeliness of autism spectrum disorder diagnosis. J. Pediatr. 166 , 1431–1439.e1 (2015).
Boterberg, S., Charman, T., Marschik, P. B., Bölte, S. & Roeyers, H. Regression in autism spectrum disorder: a critical overview of retrospective findings and recommendations for future research. Neurosci. Biobehav. Rev. 102 , 24–55 (2019).
Pearson, N., Charman, T., Happé, F., Bolton, P. F. & McEwen, F. S. Regression in autism spectrum disorder: reconciling findings from retrospective and prospective research. Autism Res. 11 , 1602–1620 (2018).
Ozonoff, S. & Iosif, A.-M. Changing conceptualizations of regression: what prospective studies reveal about the onset of autism spectrum disorder. Neurosci. Biobehav. Rev. 100 , 296–304 (2019). Despite its potential importance as a biological marker and/or subgroup of ASD, developmental regression has remained very poorly understood. This paper outlines recent data and reconceptualization about patterns of onset (and loss) that chime with a more contemporaneous understanding of ASD as a heterogeneous condition in terms of its manifestation both within and across individuals .
Brugha, T. S. et al. Validating two survey methods for identifying cases of autism spectrum disorder among adults in the community. Psychol. Med. 42 , 647–656 (2012).
Brugha, T. S. The Psychiatry of Adult Autism and Asperger Syndrome: a Practical Guide (Oxford Univ. Press, 2018).
Epstein, J., Johnson, D. E. & Conners, C. K. Conners Adult ADHD Diagnostic Interview for DSM-IV (CAADID) (MHS, 2001).
Lai, M.-C. et al. Prevalence of co-occurring mental health diagnoses in the autism population: a systematic review and meta-analysis. Lancet Psychiatry 6 , 819–829 (2019).
Havdahl, A. & Bishop, S. Heterogeneity in prevalence of co-occurring psychiatric conditions in autism. Lancet Psychiatry 6 , 794–795 (2019).
Croen, L. A. et al. The health status of adults on the autism spectrum. Autism 19 , 814–823 (2015).
Mannion, A., Leader, G. & Healy, O. An investigation of comorbid psychological disorders, sleep problems, gastrointestinal symptoms and epilepsy in children and adolescents with autism spectrum disorder. Res. Autism Spectr. Disord. 7 , 35–42 (2013).
Soke, G. N., Maenner, M. J., Christensen, D., Kurzius-Spencer, M. & Schieve, L. A. Prevalence of co-occurring medical and behavioral conditions/symptoms among 4- and 8-year-old children with autism spectrum disorder in selected areas of the United States in 2010. J. Autism Dev. Disord. 48 , 2663–2676 (2018).
Chandler, S. et al. Emotional and behavioural problems in young children with autism spectrum disorder. Dev. Med. Child Neurol. 58 , 202–208 (2016).
Pezzimenti, F., Han, G. T., Vasa, R. A. & Gotham, K. Depression in youth with autism spectrum disorder. Child Adolesc. Psychiatr. Clin. N. Am. 28 , 397–409 (2019).
Hwang, Y. I. J., Srasuebkul, P., Foley, K. R., Arnold, S. & Trollor, J. N. Mortality and cause of death of Australians on the autism spectrum. Autism Res. 12 , 806–815 (2019).
Hirvikoski, T. et al. Premature mortality in autism spectrum disorder. Br. J. Psychiatry 208 , 232–238 (2016).
Havdahl, K. A. et al. Multidimensional influences on autism symptom measures: implications for use in etiological research. J. Am. Acad. Child Adolesc. Psychiatry 55 , 1054–1063.e3 (2016).
Nicolaidis, C. et al. Comparison of healthcare experiences in autistic and non-autistic adults: a cross-sectional online survey facilitated by an academic-community partnership. J. Gen. Intern. Med. 28 , 761–769 (2013).
Schreibman, L. et al. Naturalistic developmental behavioral interventions: empirically validated treatments for autism spectrum disorder. J. Autism Dev. Disord. 45 , 2411–2428 (2015).
Tomlinson, M. et al. Setting global research priorities for developmental disabilities, including intellectual disabilities and autism: setting research priorities for developmental disabilities. J. Intellect. Disabil. Res. 58 , 1121–1130 (2014).
Rahman, A. et al. Effectiveness of the parent-mediated intervention for children with autism spectrum disorder in South Asia in India and Pakistan (PASS): a randomised controlled trial. Lancet Psychiatry 3 , 128–136 (2016).
Lovaas, O. I. Behavioral treatment and normal educational and intellectual functioning in young autistic children. J. Consult. Clin. Psychol. 55 , 3–9 (1987).
Nevill, R. E., Lecavalier, L. & Stratis, E. A. Meta-analysis of parent-mediated interventions for young children with autism spectrum disorder. Autism 22 , 84–98 (2018).
Kasari, C. et al. Randomized controlled trial of parental responsiveness intervention for toddlers at high risk for autism. Infant Behav. Dev. 37 , 711–721 (2014).
Shire, S. Y. et al. Hybrid implementation model of community-partnered early intervention for toddlers with autism: a randomized trial. J. Child Psychol. Psychiatry 58 , 612–622 (2017).
Siller, M., Hutman, T. & Sigman, M. A parent-mediated intervention to increase responsive parental behaviors and child communication in children with ASD: a randomized clinical trial. J. Autism Dev. Disord. 43 , 540–555 (2013).
Rogers, S. J. et al. Effects of a brief early start denver model (ESDM)-based parent intervention on toddlers at risk for autism spectrum disorders: a randomized controlled trial. J. Am. Acad. Child Adolesc. Psychiatry 51 , 1052–1065 (2012).
Green, J. et al. Parent-mediated communication-focused treatment in children with autism (PACT): a randomised controlled trial. Lancet 375 , 2152–2160 (2010).
Pickles, A. et al. Parent-mediated social communication therapy for young children with autism (PACT): long-term follow-up of a randomised controlled trial. Lancet 388 , 2501–2509 (2016).
Dawson, G. et al. Randomized, controlled trial of an intervention for toddlers with autism: the Early Start Denver Model. Pediatrics 125 , e17–e23 (2010).
Charman, T. Editorial: trials and tribulations in early autism intervention research. J. Am. Acad. Child Adolesc. Psychiatry 58 , 846–848 (2019).
Rogers, S. J. et al. A multisite randomized controlled two-phase trial of the early start denver model compared to treatment as usual. J. Am. Acad. Child Adolesc. Psychiatry 58 , 853–865 (2019).
Dawson, G. et al. Early behavioral intervention is associated with normalized brain activity in young children with autism. J. Am. Acad. Child Adolesc. Psychiatry 51 , 1150–1159 (2012).
Myers, S. M., Johnson, C. P. & The Council on Children With Disabilities. Management of children with autism spectrum disorders. Pediatrics 120 , 1162–1182 (2007).
Laugeson, E. A., Frankel, F., Gantman, A., Dillon, A. R. & Mogil, C. Evidence-based social skills training for adolescents with autism spectrum disorders: the UCLA PEERS program. J. Autism Dev. Disord. 42 , 1025–1036 (2012).
Reichow, B., Servili, C., Yasamy, M. T., Barbui, C. & Saxena, S. Non-specialist psychosocial interventions for children and adolescents with intellectual disability or lower-functioning autism spectrum disorders: a systematic review. PLOS Med. 10 , e1001572 (2013).
Brignell, A. et al. Communication interventions for autism spectrum disorder in minimally verbal children. Cochrane Database Syst. Rev. 11 , CD012324 (2018).
PubMed Google Scholar
Tarver, J. et al. Child and parent outcomes following parent interventions for child emotional and behavioral problems in autism spectrum disorders: a systematic review and meta-analysis. Autism 23 , 1630–1644 (2019).
Keefer, A. et al. Exploring relationships between negative cognitions and anxiety symptoms in youth with autism spectrum disorder. Behav. Ther. 49 , 730–740 (2018).
Bearss, K. et al. Effect of parent training vs parent education on behavioral problems in children with autism spectrum disorder: a randomized clinical trial. JAMA 313 , 1524–1533 (2015).
Da Paz, N. S. & Wallander, J. L. Interventions that target improvements in mental health for parents of children with autism spectrum disorders: a narrative review. Clin. Psychol. Rev. 51 , 1–14 (2017).
Kasari, C. et al. Children with autism spectrum disorder and social skills groups at school: a randomized trial comparing intervention approach and peer composition. J. Child Psychol. Psychiatry 57 , 171–179 (2016).
Marshall, D. et al. Social stories in mainstream schools for children with autism spectrum disorder: a feasibility randomised controlled trial. BMJ Open 6 , e011748 (2016).
Taylor, J. L. et al. A systematic review of vocational interventions for young adults with autism spectrum disorders. Pediatrics 130 , 531–538 (2012).
Pallathra, A. A., Cordero, L., Wong, K. & Brodkin, E. S. Psychosocial interventions targeting social functioning in adults on the autism spectrum: a literature review. Curr. Psychiatry Rep. 21 , 5 (2019).
White, S. W. et al. Psychosocial treatments targeting anxiety and depression in adolescents and adults on the autism spectrum: review of the latest research and recommended future directions. Curr. Psychiatry Rep. 20 , 82 (2018).
Shattuck, P. T., Wagner, M., Narendorf, S., Sterzing, P. & Hensley, M. Post-high school service use among young adults with an autism spectrum disorder. Arch. Pediatr. Adolesc. Med. 165 , 141–146 (2011).
Wehman, P. et al. Effects of an employer-based intervention on employment outcomes for youth with significant support needs due to autism. Autism 21 , 276–290 (2017).
McCracken, J. T. et al. Risperidone in children with autism and serious behavioral problems. N. Engl. J. Med. 347 , 314–321 (2002).
Owen, R. et al. Aripiprazole in the treatment of irritability in children and adolescents with autistic disorder. Pediatrics 124 , 1533–1540 (2009).
McPheeters, M. L. et al. A systematic review of medical treatments for children with autism spectrum disorders. Pediatrics 127 , e1312–e1321 (2011).
Anagnostou, E. et al. Metformin for treatment of overweight induced by atypical antipsychotic medication in young people with autism spectrum disorder: a randomized clinical trial. JAMA Psychiatry 73 , 928–937 (2016).
Research Units on Pediatric Psychopharmacology Autism Network. Randomized, controlled, crossover trial of methylphenidate in pervasive developmental disorders with hyperactivity. Arch. Gen. Psychiatry 62 , 1266–1274 (2005).
Handen, B. L. et al. Atomoxetine, parent training, and their combination in children with autism spectrum disorder and attention-deficit/hyperactivity disorder. J. Am. Acad. Child Adolesc. Psychiatry 54 , 905–915 (2015).
Scahill, L. et al. Extended-release guanfacine for hyperactivity in children with autism spectrum disorder. Am. J. Psychiatry 172 , 1197–1206 (2015).
Hollander, E. et al. A double-blind placebo-controlled trial of fluoxetine for repetitive behaviors and global severity in adult autism spectrum disorders. Am. J. Psychiatry 169 , 292–299 (2012).
King, B. H. et al. Lack of efficacy of citalopram in children with autism spectrum disorders and high levels of repetitive behavior: citalopram ineffective in children with autism. Arch. Gen. Psychiatry 66 , 583–590 (2009).
Anagnostou, E. et al. Intranasal oxytocin in the treatment of autism spectrum disorders: a review of literature and early safety and efficacy data in youth. Brain Res. 1580 , 188–198 (2014).
Guastella, A. J. et al. The effects of a course of intranasal oxytocin on social behaviors in youth diagnosed with autism spectrum disorders: a randomized controlled trial. J. Child Psychol. Psychiatry 56 , 444–452 (2015).
Parker, K. J. et al. A randomized placebo-controlled pilot trial shows that intranasal vasopressin improves social deficits in children with autism. Sci. Transl. Med. 11 , eaau7356 (2019).
Bolognani, F. et al. A phase 2 clinical trial of a vasopressin V1a receptor antagonist shows improved adaptive behaviors in men with autism spectrum disorder. Sci. Transl. Med. 11 , eaat7838 (2019).
Rubenstein, J. L. R. & Merzenich, M. M. Model of autism: increased ratio of excitation/inhibition in key neural systems. Genes Brain Behav. 2 , 255–267 (2003).
Veenstra-VanderWeele, J. et al. Arbaclofen in children and adolescents with autism spectrum disorder: a randomized, controlled, phase 2 trial. Neuropsychopharmacology 42 , 1390–1398 (2017).
Berry-Kravis, E. et al. Mavoglurant in fragile X syndrome: results of two randomized, double-blind, placebo-controlled trials. Sci. Transl. Med. 8 , 321ra5 (2016).
Krueger, D. A. et al. Everolimus for treatment of tuberous sclerosis complex-associated neuropsychiatric disorders. Ann. Clin. Transl. Neurol. 4 , 877–887 (2017).
Georgiades, S. & Kasari, C. Reframing optimal outcomes in autism. JAMA Pediatr. 172 , 716–717 (2018).
Bishop-Fitzpatrick, L. et al. Characterizing objective quality of life and normative outcomes in adults with autism spectrum disorder: an exploratory latent class analysis. J. Autism Dev. Disord. 46 , 2707–2719 (2016).
The WHOQOL Group. Development of the World Health Organization WHOQOL-BREF quality of life assessment. Psychol. Med. 28 , 551–558 (1998).
Gotham, K. et al. Characterizing the daily life, needs, and priorities of adults with autism spectrum disorder from interactive autism network data. Autism 19 , 794–804 (2015).
Taylor, J. L. & Seltzer, M. M. Employment and post-secondary educational activities for young adults with autism spectrum disorders during the transition to adulthood. J. Autism Dev. Disord. 41 , 566–574 (2011).
Orsmond, G. I., Shattuck, P. T., Cooper, B. P., Sterzing, P. R. & Anderson, K. A. Social participation among young adults with an autism spectrum disorder. J. Autism Dev. Disord. 43 , 2710–2719 (2013).
Henninger, N. A. & Taylor, J. L. Outcomes in adults with autism spectrum disorders: a historical perspective. Autism 17 , 103–116 (2013).
Howlin, P. & Moss, P. Adults with autism spectrum disorders. Can. J. Psychiatry 57 , 275–283 (2012).
Farley, M. A. et al. Twenty-year outcome for individuals with autism and average or near-average cognitive abilities. Autism Res. 2 , 109–118 (2009).
Taylor, J. L., Henninger, N. A. & Mailick, M. R. Longitudinal patterns of employment and postsecondary education for adults with autism and average-range IQ. Autism 19 , 785–793 (2015).
Lai, M.-C. et al. Quantifying and exploring camouflaging in men and women with autism. Autism 21 , 690–702 (2016).
van Heijst, B. F. & Geurts, H. M. Quality of life in autism across the lifespan: a meta-analysis. Autism 19 , 158–167 (2015).
Moss, P., Mandy, W. & Howlin, P. Child and adult factors related to quality of life in adults with autism. J. Autism Dev. Disord. 47 , 1830–1837 (2017).
Bishop-Fitzpatrick, L., Mazefsky, C. A. & Eack, S. M. The combined impact of social support and perceived stress on quality of life in adults with autism spectrum disorder and without intellectual disability. Autism 22 , 703–711 (2017).
Kamio, Y., Inada, N. & Koyama, T. A nationwide survey on quality of life and associated factors of adults with high-functioning autism spectrum disorders. Autism 17 , 15–26 (2013).
Mason, D. et al. Predictors of quality of life for autistic adults. Autism Res. 11 , 1138–1147 (2018).
Autistica. Your questions shaping future autism research. https://www.autistica.org.uk/downloads/files/Autism-Top-10-Your-Priorities-for-Autism-Research.pdf (2016).
Ontario Brain Institute. Community priorities for research on neurodevelopmental disorders. http://braininstitute.ca/img/JLA-NDD-Final-Report.pdf (2018).
den Houting, J. Neurodiversity: an insider’s perspective. Autism 23 , 271–273 (2018).
Szatmari, P. Risk and resilience in autism spectrum disorder: a missed translational opportunity? Dev. Med. Child Neurol. 60 , 225–229 (2018).
Markowitz, L. A. et al. Development and psychometric evaluation of a psychosocial quality-of-life questionnaire for individuals with autism and related developmental disorders. Autism 20 , 832–844 (2016).
Ryan, S. & Cole, K. R. From advocate to activist? Mapping the experiences of mothers of children on the autism spectrum. J. Appl. Res. Intellect. Disabil. 22 , 43–53 (2009).
McCann, D., Bull, R. & Winzenberg, T. The daily patterns of time use for parents of children with complex needs: a systematic review. J. Child Health Care 16 , 26–52 (2012).
Karst, J. S. & Van Hecke, A. V. Parent and family impact of autism spectrum disorders: a review and proposed model for intervention evaluation. Clin. Child Fam. Psychol. Rev. 15 , 247–277 (2012).
Lounds, J., Seltzer, M. M., Greenberg, J. S. & Shattuck, P. T. Transition and change in adolescents and young adults with autism: longitudinal effects on maternal well-being. Am. J. Ment. Retard. 112 , 401–417 (2007).
Burke, M. & Heller, T. Individual, parent and social-environmental correlates of caregiving experiences among parents of adults with autism spectrum disorder. J. Intellect. Disabil. Res. 60 , 401–411 (2016).
Kim, S. H., Bal, V. H. & Lord, C. Longitudinal follow-up of academic achievement in children with autism from age 2 to 18. J. Child Psychol. Psychiatry 59 , 258–267 (2017).
Lord, C., Bishop, S. & Anderson, D. Developmental trajectories as autism phenotypes. Am. J. Med. Genet. C Semin. Med. Genet. 169 , 198–208 (2015).
Global Research on Developmental Disabilities Collaborators. Developmental disabilities among children younger than 5 years in 195 countries and territories, 1990–2016: a systematic analysis for the Global Burden of Disease Study 2016. Lancel Glob. Health 6 , e1100–e1121 (2018).
Kahn, R. S. et al. Schizophrenia. Nat. Rev. Dis. Primers 1 , 15067 (2015).
Patel, V. et al. Addressing the burden of mental, neurological, and substance use disorders: key messages from Disease Control Priorities, 3rd edition. Lancet 387 , 1672–1685 (2016).
Franz, L., Chambers, N., von Isenburg, M. & de Vries, P. J. Autism spectrum disorder in sub-Saharan Africa: a comprehensive scoping review. Autism Res. 10 , 723–749 (2017).
World Health Organization. Training parents to transform children’s lives. https://www.who.int/mental_health/maternal-child/PST/en/ (WHO, 2019).
Naslund, J. A. et al. Digital innovations for global mental health: opportunities for data science, task sharing, and early intervention. Curr. Treat. Options Psychiatry https://doi.org/10.1007/s40501-019-00186-8 (2019).
Sadowsky, J., Donvan, J. & Zucker, C. In a different key: the story of autism. J. Hist. Behav. Sci. 54 , 66–67 (2018). This paper presents a different, broad overview of the changes in perspective about autism and ASD over the years .
Rutter, M., Greenfeld, D. & Lockyer, L. A five to fifteen year follow-up study of infantile psychosis. II. Social and behavioural outcome. Br. J. Psychiatry 113 , 1183–1199 (1967).
Hermelin, B. & O’Connor, N. Psychological Experiments with Autistic Children (Pergamon Press, 1970).
Rimland, B. Infantile Autism: the Syndrome and its Implications for a Neural Theory of Behaviour (Meredith Publishing Company, 1964).
Frith, U. Studies in pattern detection in normal and autistic children: I. Immediate recall of auditory sequences. J. Abnorm. Psychol. 76 , 413–420 (1970).
Folstein, S. & Rutter, M. in Autism (eds. Rutter M. & Schopler E.) 219–241 (Springer, 1978).
Mundy, P., Sigman, M. & Kasari, C. A longitudinal study of joint attention and language development in autistic children. J. Autism Dev. Disord. 20 , 115–128 (1990).
Schopler, E. & Reichler, R. J. Parents as cotherapists in the treatment of psychotic children. J. Autism Child. Schizophr. 1 , 87–102 (1971).
Sinclair, J. Don’t mourn for us. Autism Network International http://www.autreat.com/dont_mourn.html (1993).
Wing, L. & Gould, J. Severe impairments of social interaction and associated abnormalities in children: epidemiology and classification. J. Autism Dev. Disord. 9 , 11–29 (1979).
Chawner, S. et al. A genetic first approach to dissecting the heterogeneity of autism: phenotypic comparison of autism risk copy number variants. Eur. Neuropsychopharmacol. 29 (Suppl. 3), S783–S784 (2019).
Modabbernia, A., Mollon, J., Boffetta, P. & Reichenberg, A. Impaired gas exchange at birth and risk of intellectual disability and autism: a meta-analysis. J. Autism Dev. Disord. 46 , 1847–1859 (2016).
Christensen, J. et al. Prenatal valproate exposure and risk of autism spectrum disorders and childhood autism. JAMA 309 , 1696–1703 (2013).
Xie, F., Peltier, M. & Getahun, D. Is the risk of autism in younger siblings of affected children moderated by sex, race/ethnicity, or gestational age? J. Dev. Behav. Pediatr. 37 , 603–609 (2016).
Guy, A. et al. Infants born late/moderately preterm are at increased risk for a positive autism screen at 2 years of age. J. Pediatr. 166 , 269–275.e3 (2015).
Schendel, D. & Bhasin, T. K. Birth weight and gestational age characteristics of children with autism, including a comparison with other developmental disabilities. Pediatrics 121 , 1155–1164 (2008).
Windham, G. C. et al. Maternal pre-pregnancy body mass index and gestational weight gain in relation to autism spectrum disorder and other developmental disorders in offspring. Autism Res. 12 , 316–327 (2019).
Schmidt, R. J. et al. Maternal periconceptional folic acid intake and risk of autism spectrum disorders and developmental delay in the CHARGE (CHildhood Autism Risks from Genetics and Environment) case-control study. Am. J. Clin. Nutr. 96 , 80–89 (2012).
Conde-Agudelo, A., Rosas-Bermudez, A. & Norton, M. H. Birth spacing and risk of autism and other neurodevelopmental disabilities: a systematic review. Pediatrics 137 , e20153482 (2016).
Lyall, K. et al. The changing epidemiology of autism spectrum disorders. Annu. Rev. Public Health 38 , 81–102 (2017).
Cheslack-Postava, K., Liu, K. & Bearman, P. S. Closely spaced pregnancies are associated with increased odds of autism in California sibling births. Pediatrics 127 , 246–253 (2011).
Conti, E., Mazzotti, S., Calderoni, S., Saviozzi, I. & Guzzetta, A. Are children born after assisted reproductive technology at increased risk of autism spectrum disorders? A systematic review. Hum. Reprod. 28 , 3316–3327 (2013).
Lehti, V. et al. Autism spectrum disorders in IVF children: a national case-control study in Finland. Hum. Reprod. 28 , 812–818 (2013).
Rossignol, D. A., Genuis, S. J. & Frye, R. E. Environmental toxicants and autism spectrum disorders: a systematic review. Transl. Psychiatry 4 , e360 (2014).
Curran, E. A. et al. Research review: birth by caesarean section and development of autism spectrum disorder and attention-deficit/hyperactivity disorder: a systematic review and meta-analysis. J. Child Psychol. Psychiatry 56 , 500–508 (2015).
Chandler, S., Howlin, P., Simonoff, E., Kennedy, J. & Baird, G. Comparison of parental estimate of developmental age with measured IQ in children with neurodevelopmental disorders. Child Care Health Dev. 42 , 486–493 (2016).
Charman, T. et al. IQ in children with autism spectrum disorders: data from the Special Needs and Autism Project (SNAP). Psychol. Med. 41 , 619–627 (2011).
Sparrow, S. S., Cicchetti, D. & Balla, D. A. Vineland Adaptive Behavior Scales, 2nd Edn. https://doi.org/10.1037/t15164-000 (AGS, 2005).
Jones, R. M., Pickles, A. & Lord, C. Evaluating the quality of peer interactions in children and adolescents with autism with the Penn Interactive Peer Play Scale (PIPPS). Mol. Autism 8 , 28 (2017).
Lord, C., Elsabbagh, M., Baird, G. & Veenstra-Vanderweele, J. Autism spectrum disorder. Lancet 392 , 508–520 (2018).
Duncan, A. W. & Bishop, S. L. Understanding the gap between cognitive abilities and daily living skills in adolescents with autism spectrum disorders with average intelligence. Autism 19 , 64–72 (2015).
Download references
Acknowledgements
The authors thank J. McCauley, S. Gaspar, K. Byrne and A. Holbrook from UCLA for help with manuscript preparation. S. Tromans is thanked for his updated review of the epidemiology literature. We recognize the many investigators who contributed research that we cannot cite due to space limitations. C.L. is supported by the Eunice Kennedy Shriver National Institute of Child Health and Human Development (NICHHD; R01 HD081199), the National Institute of Mental Health (NIMH; R01MH081873-01A1) and the Simons Foundation. T.S.B. is supported by grants from the Health and Social Care Information Centre, Leeds, and the National Institute for Health Research (NIHR HTA; grant ref. NIHR127337). T.C. is supported by grants from Innovative Medicines Initiative 2 (no. 777394), the Medical Research Council (MRC; grants MR/K021389/1) and the NIHR (grant 13/119/18). J.C. is funded by Autistica. G.D. is supported by the Institut Pasteur. T.F. is supported by the Autism Speaks Foundation. E.J.H.J. is supported by grants from the Economic and Social Research Council (ESRC; ES/R009368/1), the Innovative Medicines Initiative 2 (no. 777394), the MRC (MR/K021389/1) and the Simons Foundation (609081). R.M.J. acknowledges the Mortimer D. Sackler Family and the NIMH (R01MH114999). J.L.T. is supported by grants from the FAR fund and the NIMH (R34 MH104428, R03 MH 112783 and R01 MH116058). A.P. is partially supported by the Biomedical Research Centre at South London and Maudsley NHS Foundation Trust and King’s College London and the NIHR (NF-SI-0617-10120). M.W.S. is supported by the National Institutes of Health (NIH; MH106934, MH109901, MH110928, MH116487 MH102342, MH111662, MH105575 and MH115747), the Overlook International Foundation and the Simons Foundation. J.V.-V. is supported by the NIH (MH016434 and MH094604), the Simons Foundation and the New York State Psychiatric Institute. The views expressed are those of the authors and not necessarily those of the NHS, the NIHR, or the Department of Health and Social Care.
Author information
Authors and affiliations.
Departments of Psychiatry and School of Education, University of California, Los Angeles, Los Angeles, CA, USA
Catherine Lord
Department of Health Sciences, University of Leicester, Leicester, UK
Traolach S. Brugha
Institute of Psychiatry, Psychology & Neuroscience, King’s College London, London, UK
Tony Charman & Andrew Pickles
Autistica, London, UK
James Cusack
Institut Pasteur, UMR3571 CNRS, Université de Paris, Paris, France
Guillaume Dumas
Autism Speaks, New York, NY, USA
Thomas Frazier
Centre for Brain & Cognitive Development, University of London, London, UK
Emily J. H. Jones
The Sackler Institute for Developmental Psychobiology, New York, NY, USA
Rebecca M. Jones
The Center for Autism and the Developing Brain, White Plains, NY, USA
Department of Psychiatry, Langley Porter Psychiatric Institute and Weill Institute for Neurosciences, University of California, San Francisco, CA, USA
Matthew W. State
Department of Pediatrics and Vanderbilt Kennedy Center, Vanderbilt University Medical Center, Nashville, TN, USA
Julie Lounds Taylor
Department of Psychiatry, Columbia University, New York, NY, USA
Jeremy Veenstra-VanderWeele
You can also search for this author in PubMed Google Scholar
Contributions
All authors read and edited the full document. Introduction (C.L.), Epidemiology (T.S.B.), Mechanisms/pathophysiology (M.W.S., G.D., R.M.J., T.C. and E.J.H.J.), Diagnosis, screening and prevention (T.C., E.J.H.J. and T.S.B.), Management (T.S.B., T.C., E.J.H.J., J.L.T. and J.V.-V.), Quality of life (J.L.T., J.C. and T.F.), Outlook (C.L. and A.P.), Overview of Primer (C.L.).
Corresponding author
Correspondence to Catherine Lord .
Ethics declarations
Competing interests.
C.L. acknowledges the receipt of royalties from Western Psychological Services for the sale of the Autism Diagnostic Interview-Revised (ADIR), the Autism Diagnostic Observation Schedule (ADOS) and the Social Communication Questionnaire (SCQ). T.S.B. has received royalties from Cambridge University Press and Oxford University Press. T.C. has served as a consultant to F. Hoffmann-La Roche. and has received royalties from Guilford Publications and Sage Publications. T.F. has received federal funding research support from, acted as a consultant to, received travel support from, and/or received a speaker’s honorarium from the Brain and Behaviour Research Foundation, Bristol-Myers Squibb, the Cole Family Research Fund, EcoEos, Forest Laboratories, Ingalls Foundation, IntegraGen, Kugona LLC, the National Institutes of Health, Roche Pharma, Shire Development and the Simons Foundation. J.L.T. receives compensation from Sage Publishers for editorial work. A.P. receives royalties from Imperial College Press, Oxford University Press and Western Psychological Services. M.W.S. serves on the scientific advisory boards and has stock or stock options for Arett Pharmaceuticals and BlackThorn Therapeutics. J.V.-V. has consulted or served on an advisory board for Novartis, Roche Pharmaceuticals and SynapDx, has received research funding from Forest, Novartis, Roche Pharmaceuticals, Seaside Therapeutics, SynapDx, and has received an editorial stipend from Springer and Wiley. All other authors declare no competing interests.
Additional information
Peer review information.
Nature Reviews Disease Primers thanks S. Spence, P. Szatmari and the other, anonymous, reviewer(s) for their contribution to the peer review of this work.
Publisher’s note
Springer Nature remains neutral with regard to jurisdictional claims in published maps and institutional affiliations.
Rights and permissions
Reprints and permissions
About this article
Cite this article.
Lord, C., Brugha, T.S., Charman, T. et al. Autism spectrum disorder. Nat Rev Dis Primers 6 , 5 (2020). https://doi.org/10.1038/s41572-019-0138-4
Download citation
Accepted : 26 November 2019
Published : 16 January 2020
DOI : https://doi.org/10.1038/s41572-019-0138-4
Share this article
Anyone you share the following link with will be able to read this content:
Sorry, a shareable link is not currently available for this article.
Provided by the Springer Nature SharedIt content-sharing initiative
This article is cited by
Spontaneous instrumental approach-avoidance learning in social contexts in autism.
- Morgan Beaurenaut
- Klara Kovarski
- Julie Grèzes
Molecular Autism (2024)
Pharmacological and non-pharmacological interventions for irritability in autism spectrum disorder: a systematic review and meta-analysis with the GRADE assessment
- Hangnyoung Choi
- Jae Han Kim
- Marco Solmi
The gut metabolite indole-3-propionic acid activates ERK1 to restore social function and hippocampal inhibitory synaptic transmission in a 16p11.2 microdeletion mouse model
- Dilong Wang
- Ningning Li
Microbiome (2024)
Effects of mini-basketball training program on social communication impairments and regional homogeneity of brain functions in preschool children with autism spectrum disorder
- Dandan Chen
BMC Sports Science, Medicine and Rehabilitation (2024)
Dietary intake and gastrointestinal symptoms are altered in children with Autism Spectrum Disorder: the relative contribution of autism-linked traits
- Saijun Huang
Nutrition Journal (2024)
Quick links
- Explore articles by subject
- Guide to authors
- Editorial policies
Sign up for the Nature Briefing newsletter — what matters in science, free to your inbox daily.

Want to create or adapt books like this? Learn more about how Pressbooks supports open publishing practices.
Autism Spectrum Disorder
Learning objectives.
- Describe the symptoms, risk factors, influences, and prevalence of autism spectrum disorder
A seminal paper published in 1943 by psychiatrist Leo Kanner described an unusual neurodevelopmental condition he observed in a group of children. Kanner called this condition early infantile autism, and it was characterized mainly by an inability to form close emotional ties with others, speech and language abnormalities, repetitive behaviors, and an intolerance of minor changes in the environment and in normal routines (Bregman, 2005). What the DSM-5 refers to as autism spectrum disorder today is a direct extension of Kanner’s work.
In 2013, the DSM-5 replaced the previous subgroups of autistic disorder (Asperger syndrome, pervasive developmental disorder not otherwise specified (PDD-NOS), and childhood disintegrative disorder) with the single term autism spectrum disorder .
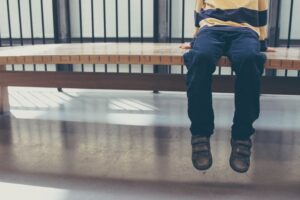
Autism spectrum disorder (ASD) is probably the most misunderstood and puzzling of neurodevelopmental disorders . Children with this disorder show signs of significant disturbances in three main areas: (a) deficits in social interaction, (b) deficits in communication, and (c) repetitive patterns of behavior or interests. These disturbances appear early in life and cause serious impairments in functioning (APA, 2013). The child with autism spectrum disorder might exhibit deficits in social interaction by not initiating conversations with other children or turning their head away when spoken to. These children do not make eye contact with others and seem to prefer playing alone rather than with others. In a certain sense, it is almost as though these individuals live in a personal and isolated social world others are simply not privy to or able to penetrate. Communication deficits can range from a complete lack of speech, to one-word responses (e.g., saying “Yes” or “No” when replying to questions or statements that require additional elaboration), to echoed speech (e.g., parroting what another person says, either immediately or several hours or even days later), to difficulty maintaining a conversation because of an inability to reciprocate others’ comments. These deficits can also include problems in using and understanding nonverbal cues (e.g., facial expressions, gestures, and postures) that facilitate normal communication.
Repetitive patterns of behavior or interests can be exhibited in a number of ways. A child with autism spectrum disorder might engage in stereotyped, repetitive movements (rocking, head-banging, or repeatedly dropping an object and then picking it up) or might show great distress at small changes in routine or the environment. For example, the child might throw a temper tantrum if an object is not in its proper place or if a regularly-scheduled activity is rescheduled. In some cases, the person with autism spectrum disorder might show highly restricted and fixated interests that appear to be abnormal in their intensity. For instance, the person might learn and memorize every detail about something even though doing so serves no apparent purpose. Importantly, autism spectrum disorder is not the same thing as intellectual development disorder (intellectual disability), although these two conditions are often comorbid. The DSM-5 specifies that the symptoms of autism spectrum disorder are not caused or explained by intellectual development disorder.
Life Problems from Autism Spectrum Disorder
Autism spectrum disorder is referred to in everyday language as autism; in fact, the disorder was termed autistic disorder in earlier editions of the DSM, and its diagnostic criteria were much narrower than those of autism spectrum disorder. The qualifier spectrum in autism spectrum disorder is used to indicate that individuals with the disorder can show a range, or spectrum, of symptoms that vary in their magnitude and severity: some severe, others less severe. The previous edition of the DSM included a diagnosis of Asperger’s disorder, generally recognized as a less severe form of autistic disorder; individuals diagnosed with Asperger’s disorder were described as having average or high intelligence and a strong vocabulary, but exhibiting impairments in social interaction and social communication, such as talking only about their special interests (Wing, Gould, & Gillberg, 2011). However, because research has failed to demonstrate that Asperger’s disorder differs qualitatively from autistic disorder, the DSM-5 dropped the distinction. Some individuals with autism spectrum disorder, particularly those with better language and intellectual skills, can live and work independently as adults. However, most do not because the symptoms remain sufficient to cause serious impairment in many areas of life (APA, 2013).
This video provides an overview of what you’ve read, describing its diagnostic criteria and the differences between the DSM-4 and DSM-5 terms for ASD.
You can view the transcript for “Autism Spectrum Disorder | Clinical Presentation” here (opens in new window) .
Epidemiology
Current estimates from the Center for Disease Control and Prevention’s Autism and Developmental Disabilities Monitoring Network indicate that one in 59 children in the United States has autism spectrum disorder; the disorder is four times more common among boys (one in 38) than in girls (one in 152) (Baio et al, 2018). Rates of autistic spectrum disorder have increased dramatically since the 1980s. For example, California saw an increase of 273% in reported cases from 1987 through 1998 (Byrd, 2002); between 2000 and 2008, the rate of autism diagnoses in the United States increased 78% (CDC, 2012). Although it is difficult to interpret this increase, it is possible that the rise in prevalence is the result of the broadening of the diagnosis, increased efforts to identify cases in the community, and greater awareness and acceptance of the diagnosis. In addition, mental health professionals are now more knowledgeable about autism spectrum disorder and are better equipped to make the diagnosis, even in subtle cases (Novella, 2008).
Causes of Autism Spectrum Disorder
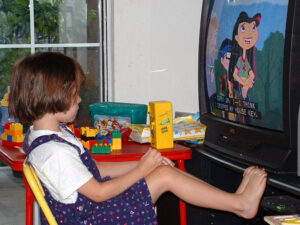
Early theories of autism placed the blame squarely on the shoulders of the child’s parents, particularly the mother. Bruno Bettelheim (an Austrian-born American child psychologist who was heavily influenced by Sigmund Freud’s ideas) suggested that a mother’s ambivalent attitudes and her frozen and rigid emotions toward her child were the main causal factors in childhood autism. In what must certainly stand as one of the more controversial assertions in psychology over the last 50 years, Bettelheim wrote, “I state my belief that the precipitating factor in infantile autism is the parent’s wish that his child should not exist” (Bettelheim, 1967, p. 125). As you might imagine, Bettelheim did not endear himself to a lot of people with this position; incidentally, no scientific evidence exists supporting his claims.
The exact causes of autism spectrum disorder remain unknown despite massive research efforts over the last two decades (Meek, Lemery-Chalfant, Jahromi, & Valiente, 2013). Autism appears to be strongly influenced by genetics, as identical twins show concordance rates of 60–90%, whereas concordance rates for fraternal twins and siblings are five to 10% (Autism Genome Project Consortium, 2007). Many different genes and gene mutations have been implicated in autism (Meek et al., 2013). Among the genes involved are those important in the formation of synaptic circuits that facilitate communication between different areas of the brain (Gauthier et al., 2011). A number of environmental factors are also thought to be associated with increased risk for autism spectrum disorder, at least in part, because they contribute to new mutations. These factors include exposure to pollutants, such as plant emissions and mercury, urban versus rural residence, and vitamin D deficiency (Kinney, Barch, Chayka, Napoleon, & Munir, 2009).
Certain prenatal and perinatal complications have been reported as possible risk factors for autism. These risk factors include maternal gestational diabetes, maternal and paternal age over 30, bleeding after the first trimester, use of prescription medication (e.g. valproate) during pregnancy, and meconium in the amniotic fluid. While research is not conclusive on the relation of these factors to autism, each of these factors has been identified more frequently in children with autism compared to their siblings who do not have autism, and other typically developing youth. While it is unclear if any single factors during the prenatal phase affect the risk of autism, complications during pregnancy may be a risk. Another hypothesized risk factor is low vitamin D levels in early development.
Child Vaccinations and Autism Spectrum Disorder
In the late 1990s, a prestigious medical journal published an article purportedly showing that autism is triggered by the MMR (measles, mumps, and rubella) vaccine. These findings were very controversial and drew a great deal of attention, sparking an international forum on whether children should be vaccinated. In a shocking turn of events, some years later, the article was retracted by the journal that had published it after accusations of fraud on the part of the lead researcher. Despite the retraction, the reporting in popular media led to concerns about a possible link between vaccines and autism persisting. A recent survey of parents, for example, found that roughly a third of respondents expressed such a concern (Kennedy, LaVail, Nowak, Basket, & Landry, 2011); and perhaps fearing that their children would develop autism, more than 10% of parents of young children refuse or delay vaccinations (Dempsey et al., 2011). Some parents of children with autism mounted a campaign against scientists who refuted the vaccine-autism link. Even politicians and several well-known celebrities weighed in; for example, actress Jenny McCarthy (who believed that a vaccination caused her son’s autism) co-authored a book on the matter. However, there is no scientific evidence that a link exists between autism and vaccinations (Hughes, 2007). Indeed, a recent study compared the vaccination histories of 256 children with autism spectrum disorder with that of 752 control children across three time periods during their first two years of life (birth to three months, birth to seven months, and birth to two years) (DeStefano, Price, & Weintraub, 2013). At the time of the study, the children were between six and 13 years old, and their prior vaccination records were obtained. Because vaccines contain immunogens (substances that fight infections), the investigators examined medical records to see how many immunogens children received to determine if those children who received more immunogens were at greater risk for developing autism spectrum disorder. The results of this study, a portion of which are shown in Figure 3 and Table 1, clearly demonstrate that the quantity of immunogens from vaccines received during the first two years of life were not at all related to the development of autism spectrum disorder. There is not a relationship between vaccinations and autism spectrum disorders.
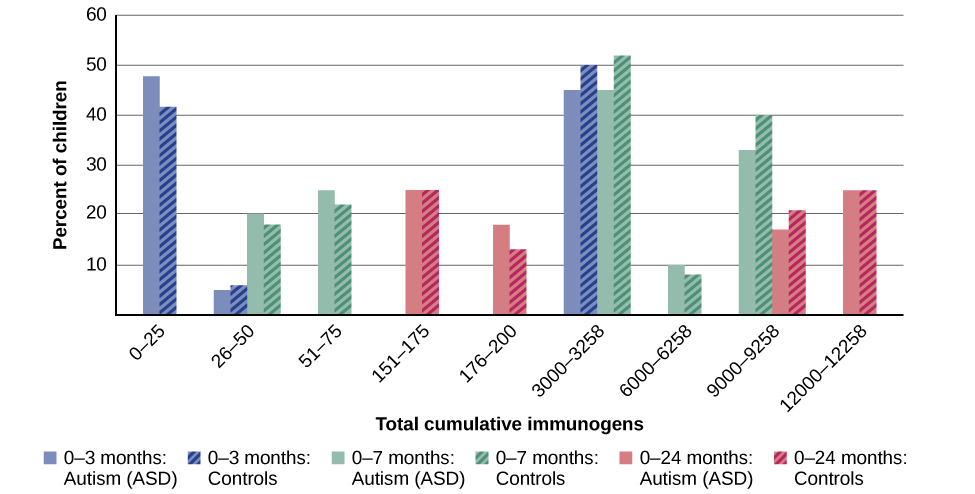
Total Cumulative Immunogens | 0 to 3 Months: Autism (ASD) | 0 to 3 Months: Controls | 0 to 7 Months: Autism (ASD) | 0 to 7 Months: Controls | 0 to 24 Months: Autism (ASD) | 0 to 24 Months: Controls |
---|---|---|---|---|---|---|
0 to 25 | 48% | 41% | – | – | – | – |
26 to 50 | 5% | 6% | 20% | 18% | – | – |
51 to 75 | – | – | 25% | 22% | – | – |
Why does concern over vaccines and autism spectrum disorder persist? Since the proliferation of the internet in the 1990s, parents have been constantly bombarded with online information that can become magnified and take on a life of its own. The enormous volume of electronic information pertaining to autism spectrum disorder, combined with how difficult it can be to grasp complex scientific concepts, can make separating good research from bad challenging (Downs, 2008). Notably, the study that fueled the controversy reported that eight out of 12 children—according to their parents—developed symptoms consistent with autism spectrum disorder shortly after receiving a vaccination. To conclude that vaccines cause autism spectrum disorder on this basis, as many did, is clearly incorrect for a number of reasons, not the least of which is because correlation does not imply causation, as you’ve learned.
Additionally, as was the case with diet and ADHD in the 1970s, the notion that autism spectrum disorder is caused by vaccinations is appealing to some because it provides a simple explanation for this condition. Like all disorders, however, there are no simple explanations for autism spectrum disorder. Although the research discussed above has shed some light on its causes, science is still a long way from a complete understanding of the disorder.
Treatments for Autism Spectrum Disorder
Several interventions can help children with autism, and the main goals of treatment are to lessen associated deficits and family distress and to increase quality of life and functional independence. In general, higher IQs are correlated with greater responsiveness to treatment and improved treatment outcomes. Although evidence-based interventions for autistic children vary in their methods, many adopt a psychoeducational approach to enhancing cognitive, communication, and social skills while minimizing problem behaviors. It’s important to keep in mind that no single treatment is best, there is no “one-size-fits-all” solution, and treatment should be tailored to the child’s needs. Intensive, sustained special education or remedial education programs and behavior therapy early in life can help children acquire self-care, social, and job skills. Available approaches include applied behavior analysis (ABA), developmental models, structured teaching, speech and language therapy, social skills therapy, and occupational therapy. Among these approaches, interventions either treat autistic features comprehensively or focus treatment on a specific area of deficit.
There has been increasing attention to the development of evidence-based interventions for young children with ASD. Two theoretical frameworks outlined for early childhood intervention include applied behavioral analysis (ABA) and the developmental social-pragmatic model (DSP). Although applied behavioral analysis (ABA) therapy has a strong evidence base, particularly in regard to early intensive home-based therapy, ABA’s effectiveness may be limited by diagnostic severity and the IQ of the person affected by ASD.
Neurodiversity
The autism rights movement promotes the concept of neurodiversity , which views autism as a natural variation of the brain rather than a disorder to be cured. In response to the rising idea that autism (and other similar disorders) are an epidemic, the concept of neurodiversity shines a new perspective on the subject.
Coined in 1998 by Australian sociologist Judy Singer, who helped popularize the concept along with American journalist Harvey Blume, neurodiversity emerged as a challenge to prevailing views that certain neurodevelopmental disorders are inherently pathological and instead adopts the social model of disability, in which societal barriers are the main contributing factor that disables people. The term represented a move away from previous “mother-blaming” theories about the cause of autism.
Neurodiversity advocates point out that neurodiverse people often have exceptional abilities alongside their weaknesses. For example, a person with ADHD may hyperfocus on some tasks while struggling to focus on others, or an autistic person may have exceptional memory or even savant skills. In light of these facts, advocates argue for recognition of strengths as well as weaknesses in neurodiverse people, and that a variety of neurological conditions that are currently classified as disorders are better regarded as differences. This view is especially popular within the autism rights movement.
Neurodiversity advocates denounce the framing of autism, ADHD, dyslexia, and other neurodevelopmental disorders as requiring medical intervention to “cure” or “fix” them and instead promote support systems, such as inclusion-focused services, accommodations, communication, and assistive technologies, occupational training, and independent living support. The intention is for individuals to receive support that honors authentic forms of human diversity, self-expression, and being, rather than treatment that coerces or forces them to adopt normative ideas of normality or to conform to a clinical ideal. Proponents of neurodiversity strive to reconceptualize autism and related conditions in society by the following measures: acknowledging that neurodiversity does not require a cure; changing the language from the current “condition, disease, disorder, or illness”-based nomenclature, and “broaden[ing] the understanding of healthy or independent living”; acknowledging new types of autonomy; and giving non-neurotypical individuals more control over their treatment, including the type, timing, and whether there should be treatment at all.
However, the neurodiversity paradigm has been controversial among disability advocates, with opponents saying that its conceptualization doesn’t reflect the realities of individuals who have high-support needs.
Key Takeaways: Autism Spectrum Disorder
autism spectrum disorder: childhood disorder characterized by deficits in social interaction and communication and repetitive patterns of behavior or interests
neurodevelopmental disorder: disorders that are first diagnosed in childhood and involve developmental problems in academic, intellectual, and social functioning
CC Licensed Content, Shared Previously
- “Autism Spectrum Disorder.” Authored by : Chrissy Hicks for Lumen Learning. Provided by : Lumen Learning. License : CC BY-SA: Attribution-ShareAlike
- Autism spectrum. Provided by : Wikipedia. Located at : https://en.wikipedia.org/wiki/Autism_spectrum . License : CC BY-SA: Attribution-ShareAlike
- Disorders in Childhood. Authored by : OpenStax College. Located at : http://cnx.org/contents/[email protected]:uASZ6djG@5/Disorders-in-Childhood . License : CC BY: Attribution . License Terms : Download for free at http://cnx.org/content/col11629/latest/.
- Girl watching tv. Authored by : oddharmonic. Provided by : Wikimedia. Located at : https://commons.wikimedia.org/wiki/File:2405784549_264fe67e22_z_copy.jpg . License : CC BY-SA: Attribution-ShareAlike
- pastel neurodiversity image. Authored by : mrmw. Provided by : Wikipedia. Located at : https://en.wikipedia.org/wiki/Autism_rights_movement#/media/File:Neurodiversity_Symbol.svg . License : CC0: No Rights Reserved
All Rights Reserved Content
- Autism Spectrum Disorder | Clinical Presentation. Provided by : MedScape. Located at : https://www.youtube.com/watch?time_continue=1&v=FCejya1WWC8&feature=emb_logo . License : Other . License Terms : Standard YouTube License
Public Domain Content
- boy sitting. Provided by : Pxhere. Located at : https://pxhere.com/en/photo/1402620 . License : CC0: No Rights Reserved
Autism Spectrum Disorder Copyright © by Meredith Palm is licensed under a Creative Commons Attribution 4.0 International License , except where otherwise noted.
Share This Book
This week: the arXiv Accessibility Forum
Help | Advanced Search
Computer Science > Machine Learning
Title: mcdgln: masked connection-based dynamic graph learning network for autism spectrum disorder.
Abstract: Autism Spectrum Disorder (ASD) is a neurodevelopmental disorder characterized by complex physiological processes. Previous research has predominantly focused on static cerebral interactions, often neglecting the brain's dynamic nature and the challenges posed by network noise. To address these gaps, we introduce the Masked Connection-based Dynamic Graph Learning Network (MCDGLN). Our approach first segments BOLD signals using sliding temporal windows to capture dynamic brain characteristics. We then employ a specialized weighted edge aggregation (WEA) module, which uses the cross convolution with channel-wise element-wise convolutional kernel, to integrate dynamic functional connectivity and to isolating task-relevant connections. This is followed by topological feature extraction via a hierarchical graph convolutional network (HGCN), with key attributes highlighted by a self-attention module. Crucially, we refine static functional connections using a customized task-specific mask, reducing noise and pruning irrelevant links. The attention-based connection encoder (ACE) then enhances critical connections and compresses static features. The combined features are subsequently used for classification. Applied to the Autism Brain Imaging Data Exchange I (ABIDE I) dataset, our framework achieves a 73.3\% classification accuracy between ASD and Typical Control (TC) groups among 1,035 subjects. The pivotal roles of WEA and ACE in refining connectivity and enhancing classification accuracy underscore their importance in capturing ASD-specific features, offering new insights into the disorder.
Comments: | 8 pages, 7 figures |
Subjects: | Machine Learning (cs.LG); Artificial Intelligence (cs.AI) |
Cite as: | [cs.LG] |
(or [cs.LG] for this version) | |
Focus to learn more arXiv-issued DOI via DataCite |
Submission history
Access paper:.
- HTML (experimental)
- Other Formats
References & Citations
- Google Scholar
- Semantic Scholar
BibTeX formatted citation

Bibliographic and Citation Tools
Code, data and media associated with this article, recommenders and search tools.
- Institution
arXivLabs: experimental projects with community collaborators
arXivLabs is a framework that allows collaborators to develop and share new arXiv features directly on our website.
Both individuals and organizations that work with arXivLabs have embraced and accepted our values of openness, community, excellence, and user data privacy. arXiv is committed to these values and only works with partners that adhere to them.
Have an idea for a project that will add value for arXiv's community? Learn more about arXivLabs .
Pardon Our Interruption
As you were browsing something about your browser made us think you were a bot. There are a few reasons this might happen:
- You've disabled JavaScript in your web browser.
- You're a power user moving through this website with super-human speed.
- You've disabled cookies in your web browser.
- A third-party browser plugin, such as Ghostery or NoScript, is preventing JavaScript from running. Additional information is available in this support article .
To regain access, please make sure that cookies and JavaScript are enabled before reloading the page.

An official website of the United States government
The .gov means it’s official. Federal government websites often end in .gov or .mil. Before sharing sensitive information, make sure you’re on a federal government site.
The site is secure. The https:// ensures that you are connecting to the official website and that any information you provide is encrypted and transmitted securely.
- Publications
- Account settings
Preview improvements coming to the PMC website in October 2024. Learn More or Try it out now .
- Advanced Search
- Journal List
- v.2017; Jan-Feb 2017
Examining the Causes of Autism
Editor’s note:.
Autism is a broad, complex, and increasingly important brain disorder. New data from the Center for Disease Control and Prevention indicate that one in sixty-eight children is born with some degree of autism. Autism is also more common in males by a four to one ratio. Making it especially difficult to discuss in finite, conclusive terms is the fact that there is no biological test for autism; diagnosis is based on behavior, and the only verified treatment is intensive behavior therapy. Our author, one of the nation’s foremost researchers on autism, examines the prenatal factors that contribute to the disorder .
As an autism researcher, I often try to put myself in the shoes of parents who have just been told that their child has autism. More and more families in the United States and around the world are facing this difficult news. The families that I’ve seen go through this often respond emotionally at first. Some go through denial; others are sad or furious. But emotions soon give way to questions. What caused my child’s autism? Was I to blame? Which treatments will help? And what does the future hold?
Autism research has made tremendous progress over the last 20 years, but yet we still can’t provide definitive answers to most of these questions. I find the autism community to be proactive, combative, and opinionated. The complexity and ambiguity of autism has spawned myriad speculations about causes—many of which have little supportive evidence. It seems clear at this point, however, that when all is said and done, we will find that autism has multiple causes that occur in diverse combinations.
To begin with, many people struggle to understand the nature of a condition so wide ranging in its severity. Autism Spectrum Disorder (ASD) or autism is a behaviorally defined neurodevelopmental disorder characterized by 1) persistent deficits in social communication and interaction across multiple contexts, and 2) restricted, repetitive patterns of behavior, interests, or activities. Few would dispute that the causes of ASD include both genetic and environmental factors. Indeed, more than 100 genes are known to confer risk 1 , 2 and 1,000 or more may ultimately be identified. 3 A wide range of potential environmental challenges have also been associated with autism, although studies in this area lag behind genomics research. A short overview of data supports genetic and environmental contributions to ASD etiology. A focus on prenatal events will hopefully clarify that the cause of autism, in the vast majority of cases, occurs prenatally, even if behavioral signs first appear several years after birth.
Twin Studies
Strong evidence against the unfounded view that autism results from neglectful parenting came in 1977 from Folstein and Rutter and the first systematic, detailed study of twin pairs containing at least one child with autism. 4 In this study, 11 of the twin pairs were monozygotic (nearly identical genetics) and 10 were dizygotic (shared approximately half of their genome with each other). The major finding was that four of the monozygotic twin pairs were concordant (both had autism), whereas none of the dyzygotic twins were. Beyond autism, nine of the eleven monozygotic pairs were concordant for some form of cognitive impairment, compared to one of ten of the dyzygotic pairs.
The researchers concluded that autism and other neurodevelopmental disorders have a strong genetic component. But environmental factors must also contribute to autism etiology, they pointed out. For the 17 twin pairs that were discordant for autism—one child had a diagnosis and the other did not—the authors speculated that direct damage to the brain might have affected the diagnosed twin. They identified five features known to be associated with brain damage, such as severe hemolytic disease, a delay in breathing of at least five minutes after birth, and neonatal convulsions. In six of the pairs, one twin—always the autistic one—experienced one or more of these insults. Looking further, they found that one of an expanded list of “biological hazards” (e.g., discrepancies in birth weight, a pathologically narrow umbilical cord) occurred in the autistic twin in 6 of the 11 remaining discordant pairs and never in the non-autistic twin. The authors concluded that “some form of biological impairment, usually in the perinatal period, strongly predisposed to the development of autism.”
Since the Folstein and Rutter paper cited above, there have been a total of 13 twin studies focused on autism. All find genetic and environmental contributions to autism, although conclusions about the proportions of the two factors and interpretations have varied substantially. One research team, 5 for example, concluded that a large proportion of the variance in liability (55 percent for strictly defined autism and 58 percent under a broader definition) can be explained by shared environmental factors, whereas genetic heritability accounts for 37 percent. This somewhat surprising finding—that environmental factors contribute more substantially than genetics—has been challenged by a more recent, large-scale twin study, 6 which found that the largest contribution to autism liability comes from additive genetic effects. And, a recent meta-analysis 7 concludes that the causes of autism are due to strong genetic effects, and that shared environmental influences are seen only if autism is very narrowly defined. A brief synopsis of the history of autism twin studies 8 finds that concordance for monozygotic twins is roughly 45 percent, versus 16 percent for dizygotic twins.
The reason for this short review of autism twin studies is to emphasize that even the best evidence for both genetic and environmental etiologies of autism leads to inconsistent conclusions about their proportional contributions. Moreover, twin studies do not typically consider that the cause of autism may involve genetic and environmental factors working together (the so-called gene by environment effect); i.e., certain environmental exposures only cause autism in individuals with a particular genetic composition. The second point is that if autism had a completely genetic etiology, we would expect a much higher concordance rate in monozygotic twins; the actual rate may reflect, in part, that even monozygotic twins do not share an identical environment prenatally. 9 , 10 Therefore, one must seriously search for environmental factors that either alone, or in combination with genetic predisposition, can increase autism risk. What are these factors?
Maternal Infection
If twin studies provide the best evidence for a genetic basis of autism, then naturally occurring pathogen exposures offer the strongest evidence of environmental etiology. The best example is maternal rubella (German measles) infection during pregnancy. Before development and widespread dissemination of effective vaccines, major pandemics occurred every 10 to 30 years. 11 The last of these was from 1963 to 1965 and infected an estimated 10 percent of pregnant women, resulting in more than 13,000 fetal or early infant deaths; 20,000 infants born with major birth defects and 10,000 to 30,000 infants born with moderate to severe neurodevelopmental disorders. Stella Chess, a child psychiatrist at New York University, studied 243 children exposed to rubella during pregnancy 12 , 13 and found that the largest category of neurodevelopmental disorder was intellectual disability, which affected 37 percent of the sample. Nine of these children were also diagnosed with autism; another, without intellectual disability, had a possible diagnosis; and eight a partial syndrome of autism. These numbers would translate to an autism prevalence of 741 per 10,000 rubella-exposed children, just over seven percent. This is striking in comparison to published prevalence rates, at the time of the study, of two to three per 10,000 in the general population. Fortunately, rubella epidemics have ended due to widespread dissemination of the measles, mumps and rubella vaccines and the association of autism with other viral or bacterial infections is weaker than with rubella. 14
Collier et al 15 have pointed out that nearly 64 percent of women surveyed in the US have experienced an infection during their pregnancies. This obviously does not lead to autism or any other neurodevelopmental disorder in most cases.
Examining prenatal environmental factors is best conducted in very large cohorts of subjects that have excellent health care records. This can be done in Scandinavian countries with their nationalized health care systems, and in large health care providers in the US.
One such study, conducted in Denmark, found no association between maternal bacterial or viral infection during pregnancy and diagnosis of ASD in the offspring, 16 although viral infection during the first trimester, or admission to the hospital due to infection during the second trimester were associated with the diagnosis. In a more recent study 17 Atladottir and colleagues found little evidence, overall, that common infectious diseases or fevers (lasting more than seven days) during pregnancy increased the risk of autism—noting, however, that influenza increased the risk of having an autistic child twofold. Use of antibiotics also increased risk. The link between influenza exposure during fetal life and increased risk for autism is in line with a series of animal studies 18 , 19 suggesting that the influenza virus activates the maternal immune system, which may be harmful to fetal brain development. But the Danish researchers seem to downplay even their statistically significant findings, suggesting that their results do not indicate that either mild infection or the use of antibiotics represent strong risk factors for autism.
A parallel set of studies has been carried out by Zerbo and colleagues in California. The first, 20 based on 1,122 children, found no association between maternal influenza and ASD but (in contrast to Atladottir et al), the occurrence of maternal fever did increase risk. A second study 21 of 2,482 children (407 with ASD) found that mothers of children with ASD were diagnosed with viral infections during pregnancy no more often than mothers of non-autistic children. Maternal bacterial infections during the second trimester and the third trimester, however, were associated with a twofold increase in ASD risk, and two or more infections diagnosed in the third trimester with even higher risk, again suggesting a link with more severe infection during pregnancy. The most recent study, 22 based on a large cohort of children (196,929) born between 2000 and 2010, found that neither maternal influenza infection during pregnancy nor influenza vaccination were associated with increased risk for ASD.
In conclusion: Some infections during pregnancy, such as German measles, clearly increase the risk of ASD. However, there seems relatively little evidence that today’s widely experienced infectious illnesses, such as influenza, during pregnancy substantially increase the risk of ASD. Perhaps the signal is weak because of gene by environment effects [as seems to be the case for different strains of mice 23 , 24 ]. If so, evidence will need to come from studies that combine large scale epidemiology with sophisticated genomic analyses.
Maternal Antibodies
Autoimmune diseases (in which immune cells erroneously identify cells in the body as foreign and attack them) mediated by circulating antibodies currently affect as much as nine percent of the world’s population, 25 and the notion that autoimmunity may be associated with neurological and psychiatric disorders goes back to the 1930s. Reviewing this contentious area of research, Goldsmith and Rogers 26 conclude that the literature, though conflicting, “contains a large amount of circumstantial, but not conclusive, evidence for immune dysfunction in patients with schizophrenia.” Interestingly, an auto-immune disorder with antibodies directed at the NMDA receptor causes an encephalopathy, which in its early stages can be indistinguishable from schizophrenia. 27
Precedents for antibody-related CNS disorders include Rasmussen encephalitis, stiff-person syndrome, neuromyelitis optica, post streptococcal movement disorders (Sydenham’s chorea and PANDAS), and systemic lupus erythematosus. 28 Judy Van de Water, of UC Davis, the main proponent of the idea that circulating antibodies may cause some forms of autism, first reported in 2008 that 12 percent of mothers of children with ASD have unusual antibodies directed at fetal brain proteins. 29 Based on more specific assays for these antibodies, she has since proposed that Maternal Antibody-Related (MAR) causes may be associated with as many as 22 percent of autism cases, suggesting that this may be a preventable form of ASD. 30 This area of research is exciting because it suggests potential therapeutic targets. Although many questions remain (e.g., how antibodies would enter the fetal brain, what neurodevelopmental processes they may alter), it is entirely possible that circulating antibodies represent prenatal environmental risk factors for ASD.
Efforts to understand the increased prevalence of autism spectrum disorder have led some to wonder whether the use of various drugs during pregnancy might be partly responsible. Historically, a strong case could be made for an association between autism and thalidomide, a potent sedative that was used (for several years around 1960) during pregnancy for the relief of nausea. A study of 100 adult Swedish patients whose mothers had taken thalidomide while pregnant 31 found that at least four had clear autistic characteristics. This was the first evidence that a drug ingested during pregnancy could substantially increase autism risk. More recently, concerns have been raised about valproic acid and serotonin reuptake inhibitors.
Valproic acid, an approved drug since the early 1960s, is primarily prescribed for epilepsy and seizure control, but also used for ailments ranging from migraine headaches to bipolar disorder. Both animal and human epidemiological studies have raised concerns that valproic acid is a teratogen. The largest epidemiological study to date 32 tracked 415 children, 201 of whom were born to mothers who took antiepileptic medication during their pregnancies. Nearly 7.5 percent of the children of the treated women had a neurodevelopmental disorder, primarily some form of autism, versus 1.9 percent in the non-epileptic women.
A recent concern has been the use of serotonin reuptake inhibitors (SSRIs) for the treatment of depression during pregnancy. Serotonin is an important brain neurotransmitter that plays a significant role in functions ranging from sleep to mood to appetite, and whose dysregulation during early fetal life can have serious negative consequences for brain development. 33 As the name implies, SSRIs, which have been in use since the late 1980s, delay the reuptake of serotonin from the synaptic cleft into the presynaptic terminal and thus enhances its effect on the postsynaptic receptors. A recent review and meta-analysis of six case-control studies and four cohort studies concluded that SSRI use during pregnancy 34 was significantly associated with increased risk of ASD in offspring.
The effect was most prominent with use of the drugs during the first and second trimesters of pregnancy. Interestingly, the researchers found that preconceptual exposure to SSRIs was also associated with increased ASD risk—as was the use of non-SSRI antidepressants. They note that a large cohort study found that, while ASD rates in the SSRI-exposed group were significantly higher than in the unexposed group, the rates in the SSRI-exposed group did not significantly differ from those among mothers with unmedicated psychiatric disorder and those who had discontinued SSRIs. It currently appears impossible to disentangle the deleterious effect of SSRIs from the fact of a maternal condition that necessitates the drug. Many authors also comment on the potentially worse effect on pregnancies of untreated maternal depression.
In sum, a brief review of the literature indicates that ingesting some drugs during pregnancy increases the risk of ASD, suggesting the need for more careful evaluation of drug safety during fetal development prior to widespread medical use.
Environmental Toxicants
Beyond viral and bacterial pathogens and medically prescribed drugs, researchers have begun investigating environmental toxicants. These range from automobile-produced air pollution to cigarette smoke to heavy metals and pesticides. 35 , 36 Small increases in autism risk have been reported if, for example, a family lives closer to a freeway or to an agricultural area during pregnancy. The field of autism environmental epidemiology is still in its infancy and techniques to comprehensively establish a prenatal “exposome” (i.e., all environmental factors affecting a fetus during pregnancy) are still under development. That said, given the unlikelihood that all autism will be explained by genetic factors, the determination of environmental causes, some of which might be avoided or minimized, may have far greater translational impact than the much better funded genetic studies. Strategies for exploring gene-by-environment interactions need to be enhanced with haste.
Postnatal Factors
Since autism is a neurological disorder that undoubtedly reflects altered brain function, it is possible that the insult to the brain occurs after birth. There is currently very little evidence for this. One historical concern was that vaccines, such as the measles, mumps, and rubella (MMR) vaccine, administered initially when the child is about one-year old, might transform a healthy child into one with autism. This fear was fueled by regressive onset in some cases—a child seems fine for the first year or so, then loses social and language function and regresses into a classical autistic syndrome. But we have found that even in children who demonstrate this regressive form of autism, brain changes begin by four to six months, long before behavior changes. 37 Moreover, many large-scale epidemiologic studies have unequivocally demonstrated no link between MMR administration and the risk of ASD (summarized in 38), the same conclusion that the US National Academy of Sciences reached in a thorough review carried out in 2011. 39
The only other postnatal experience that has been linked to the onset of ASD is profound social isolation in institution-reared children, such as those in the Romanian orphanage system. 40 Rutter and colleagues 41 found that nearly 10 percent of children raised in Romanian orphanages and adopted by British families showed some features of autism. These children were very poorly treated in the orphanage (most were underweight and had intellectual disability and various medical problems). While fully qualifying for an autism diagnosis at age 4, they showed substantial improvement and less severe autism symptoms by age 6. Is this truly autism? The authors conclude: “The characteristics of these children with autistic features, although phenomenologically similar in some respects to those found in “ordinary” autism, differed sharply in the marked improvement evident between 4 and 6 years of age and in the degree of social interest... The quasi-autistic pattern seemed to be associated with a prolonged experience of perceptual and experiential privation, with a lack of opportunity to develop attachment relationships, and with cognitive impairment.”
This sad epoch demonstrates both the potential for severely abnormal rearing practices to influence brain regions that are affected by typical causes of autism, and the resilience of the brain in compensating and restoring once the individual is placed in a more normal environment. But it does not provide evidence for the postnatal genesis of autism.
The research picture regarding the causes for Autism Spectrum Disorder remains complex, although there is certainly a very strong genetic component. While there are some genes, such as CHD8, the mutation of which almost always cause autism in a very low percentage of cases 42 most mutations seem to confer small increases in risk. Similarly, while some environmental factors, such as rubella infection or fetal exposure to valproic acid, have been highly associated with autism risk, the increase in risk associated with others, such as living close to a highway, is small. It is very likely that the answer to what causes autism will not reside solely in genetics or in environment but in a combination of the two. Whatever factors go into the mix, they most likely have their effect during fetal life: a person with autism is born with autism.
David G. Amaral , Ph.D., is a Distinguished Professor in the Department of Psychiatry and Behavioral Sciences at UC Davis. He is also the Beneto Foundation Chair and Research Director of the MIND Institute, which is dedicated to studying autism and other neurodevelopmental disorders. As research director, he coordinates a multidisciplinary analysis of children with autism called the Autism Phenome Project to define clinically significant subtypes of autism. More recently, Amaral has become Director of Autism BrainNet, a collaborative effort to solicit postmortem brain tissue to facilitate autism research. In April of 2015, Amaral became editor-in-chief of Autism Research , the journal of the International Society for Autism Research. In 2016, he was appointed to the Interagency Autism Coordinating Committee by the Secretary of Health and Human Services. Amaral received a joint Ph.D. in neuroscience and psychology from the University of Rochester and conducted postdoctoral research at the Department of Anatomy and Neurobiology at Washington University. He also conducted research at the Salk Institute for Biological Studies and served as an adjunct professor in the Department of Psychiatry at UC San Diego.
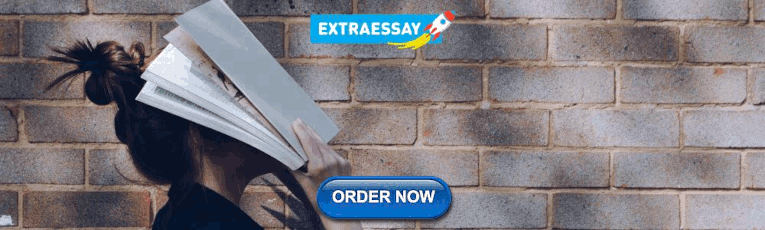
IMAGES
VIDEO
COMMENTS
Abstract. Autism spectrum disorder (ASD) is a neurodevelopmental disorder characterized by deficits in social communication and the presence of restricted interests and repetitive behaviors. There have been recent concerns about increased prevalence, and this article seeks to elaborate on factors that may influence prevalence rates, including ...
About the journal. Research in Autism Spectrum Disorders (RASD) publishes high quality empirical articles and reviews that contribute to a better understanding of Autism Spectrum Disorders (ASD) at all levels of description; genetic, neurobiological, cognitive, and behavioral. The primary focus of the journal is to …. View full aims & scope.
Autism spectrum disorders (ASD) are a group of neurodevelopmental disorders that affect individuals' social interactions, communication skills, and behavioral patterns, with significant individual differences and complex etiology. This article reviews the definition and characteristics of ASD, epidemiological profile, early research and diagnostic history, etiological studies, advances in ...
Autism spectrum disorders articles from across Nature Portfolio. Autism spectrum disorders are a group of neurodevelopmental disorders that are characterized by impaired social interaction and ...
Prevalence figures that referred to 4.5 per 10,000 in the 1960s have been replaced by newer estimates suggesting that 1 in 59 children (16 per 1,000) present with an autism spectrum disorder (ASD) in 2014 . The widening of the definition of autism has undoubtedly contributed to the significant increase in the numbers of people being diagnosed.
Autism spectrum disorder (ASD) is a neuro-developmental disorder that results various impairments in social interaction, communication, and the existence of unvaried patterns of behaviour in ...
In late 2001-early 2002 we received four exciting papers with findings on the genetics of autism that were published together in our March 2002 issue, with an accompanying editorial [2,3,4,5,6 ...
Autism spectrum disorder (ASD) is a complex neurodevelopmental disorder with an estimated prevalence of one in 54 (1.85%; (Maenner et al., 2020)), 52 million cases worldwide and 7.7 million disability adjusted life years (Baxter et al., 2014).Although ASD can be diagnosed as early as 18 months of age (Hyman et al., 2020), the latest review indicated that, globally, the mean age at ASD ...
Research Papers. select article Cardiovascular risk and emotion regulation contribute to depression symptomatology in middle-aged and older autistic adults. ... select article Higher anesthetic dose requirement for sedation in children with autism spectrum disorder compared to neuro-atypical controls- a prospective observational study.
Introduction. Autism spectrum disorder (ASD) refers to a group of early-onset, lifelong, heterogeneous neurodevelopmental conditions with complex mechanisms of emergence ().The prevalence of ASD has increased from 1 in 69 by 2012 to 1 in 44 by 2018, as reported by the Centers for Disease Control and Prevention for 2012-2018 (2, 3).Recent research estimates the male-to-female ratio is closer ...
Research Papers. select article The association between motor coordination impairment and restricted/repetitive behaviors in autistic children: The partial mediating effect of executive function ... select article Methodological considerations in the use of standardized motor assessment tools for children with autism spectrum disorder: A ...
Autism is a major, peer-reviewed, international journal, published 8 times a year, publishing research of direct and practical relevance to help improve the quality of life for individuals with autism or autism-related disorders. It is interdisciplinary in nature, focusing on research in many areas, including: intervention; diagnosis; training; education; translational issues related to ...
This paper presents the work done in implementing a NAO humanoid robot in a research experiment with ASD children. ... Autism spectrum disorder (ASD) is a highly heritable neurodevelopment disease ...
IntroductionThe wide range of psychosocial interventions designed to assist people with Autism Spectrum Disorder (ASD) makes it challenging to compile and hierarchize the scientific evidence that supports the efficacy of these interventions. ... Internet survey of treatments used by parents of children with autism. Research in Developmental ...
Advances in autism research, 2021: continuing to decipher the secrets of autism. 1427. trimester average and maximal daily exposure to ne air. fi. particulate matter of diameter ≤2.5 μm (PM2.5 ...
Autism spectrum disorder (ASD) is a lifelong condition characterised by social communication difficulties and repetitive behaviours (American Psychiatric Association 2013). ... The final assurance of inclusive research was the involvement in the research and paper authorship of a parent with lived experiences of educational support for a young ...
Recent Research Progress in Autism Spectrum Disorder. On April 2, 2017, the world will celebrate the ninth annual World Autism Awareness Day. In honor of this occasion, Neuroscience Bulletin has put together a Special Issue of reviews and primary research articles focusing on autism spectrum disorder (ASD). ASD is a heterogeneous developmental ...
AL-Muthanna, Iraq. Abstract. Autism is a lifelong neuro developmental condition. It is characterised by differences in behavior, social. interaction, communication, special interests and sensory ...
Autism Spectrum Disorder (ASD) is a neurological disorder which might have a lifelong impact on the language learning, speech, cognitive, and social skills of an individual. ... The main objective of our paper is to thus determine if the child is susceptible to ASD in its nascent stages, which would help streamline the diagnosis process ...
Abstract. Autism spectrum disorder is a construct used to describe individuals with a specific combination of impairments in social communication and repetitive behaviours, highly restricted ...
Autism Spectrum Disorder. A seminal paper published in 1943 by psychiatrist Leo Kanner described an unusual neurodevelopmental condition he observed in a group of children. ... there are no simple explanations for autism spectrum disorder. Although the research discussed above has shed some light on its causes, science is still a long way from ...
Abstract. Autism spectrum disorder (ASD) is a set of neurodevelopmental disorders characterized by a deficit in social behaviors and nonverbal interactions such as reduced eye contact, facial expression, and body gestures in the first 3 years of life. It is not a single disorder, and it is broadly considered to be a multi-factorial disorder ...
Autism Spectrum Disorder (ASD) is a neurodevelopmental disorder characterized by complex physiological processes. Previous research has predominantly focused on static cerebral interactions, often neglecting the brain's dynamic nature and the challenges posed by network noise. To address these gaps, we introduce the Masked Connection-based Dynamic Graph Learning Network (MCDGLN). Our approach ...
2 Autism Spectrum Disorder Blog for Parents and Family Members Autism is a complicated neurodevelopmental disorder that affects how people interact with the outside environment and other people. It is also known as Autism Spectrum Disorder (ASD). It is called a spectrum because it encompasses a broad variety of behaviors, difficulties, and abilities.
Introduction. Autism spectrum disorders (ASDs) are a group of pervasive developmental disabilities known as the most prevalent, serious, and yet unknown disorders during childhood.[1,2] Children with ASD are identified by a range of problems in their emotional, physical, and interactional skills, daily routines and playing, language development and natural speech, as well as imitative ability.[]
The research picture regarding the causes for Autism Spectrum Disorder remains complex, although there is certainly a very strong genetic component. While there are some genes, such as CHD8, the mutation of which almost always cause autism in a very low percentage of cases 42 most mutations seem to confer small increases in risk.