An official website of the United States government
Official websites use .gov A .gov website belongs to an official government organization in the United States.
Secure .gov websites use HTTPS A lock ( Lock Locked padlock icon ) or https:// means you've safely connected to the .gov website. Share sensitive information only on official, secure websites.
- Publications
- Account settings
- Advanced Search
- Journal List

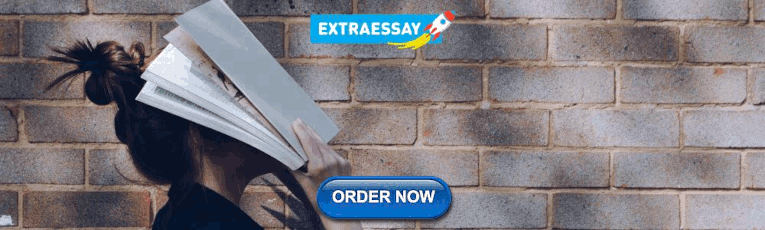
Decision-Making Processes in Social Contexts
Elizabeth bruch, fred feinberg.
- Author information
- Article notes
- Copyright and License information
Please direct correspondence to Elizabeth Bruch at: 500 S. State Street, Department of Sociology, University of Michigan, Ann Arbor, MI, 48104
Issue date 2017 Jul.
Over the past half-century, scholars in the interdisciplinary field of Judgment and Decision Making have amassed a trove of findings, theories, and prescriptions regarding the processes ordinary people enact when making choices. But this body of knowledge has had little influence on sociology. Sociological research on choice emphasizes how features of the social environment shape individual behavior, not people’s underlying decision processes. Our aim in this article is to provide an overview of selected ideas, models, and data sources from decision research that can fuel new lines of inquiry on how socially situated actors navigate both everyday and major life choices. We also highlight opportunities and challenges for cross-fertilization between sociology and decision research that can allow the methods, findings, and contexts of each field to expand their joint range of inquiry.
Keywords: heuristics, decision making, micro-sociology, discrete choice
INTRODUCTION
Over the past several decades, there has been an explosion of interest in, and recognition of the importance of, how people make decisions. From Daniel Kahneman’s 2002 Nobel Prize for his work on “Heuristics and Biases,” to the rise in prominence of Behavioral Economics, to the burgeoning policy applications of behavioral “nudges” ( Kahneman 2003 ; Camerer & Loewenstein 2004 ; Shafir 2013 ), both scholars and policy makers increasingly focus on choice processes as a key domain of research and intervention. Researchers in the interdisciplinary field of Judgment and Decision Making (JDM)—which primarily comprises cognitive science, behavioral economics, academic marketing, and organizational behavior—have generated a wealth of findings, insights, and prescriptions regarding how people make choices. In addition, with the advent of rich observational data from purchase histories, a related line of work has revolutionized statistical models of decision making that aim to represent underlying choice process.
But for the most part these models and ideas have not penetrated sociology. 1 We believe there are several reasons for this. First, JDM research has largely been focused on contrasting how a fully informed, computationally unlimited (i.e., “rational”) person would behave to how people actually behave, and pointing out systematic deviations from this normative model ( Loewenstein 2001 ). Since sociology never fully embraced the rational choice model of behavior, debunking it is less of a disciplinary priority. 2 Second, JDM research is best known for its focus on problems that involve risk , where the outcome is probabilistic and the payoff probabilities are known ( Kahneman & Tversky 1979 , 1982 , 1984 ), and ambiguity , where the outcome is probabilistic and the decision-maker does not have complete information on payoff probabilities ( Ellsberg 1961 ; Einhorn & Hogarth 1988 ; Camerer & Weber 1992 ). In both these cases, there is an optimal choice to be made, and the research explores how people’s choices deviate from that answer. But most sociological problems—such as choosing a romantic partner, neighborhood, or college—are characterized by obscurity : there is no single, obvious, optimal, or correct answer.
Perhaps most critically, the JDM literature has by and large minimized the role of social context in decision processes. This is deliberate. Most experiments performed by psychologists are designed to isolate processes that can be connected with features of decision tasks or brain functioning; it is incumbent on researchers working in this tradition to “de-socialize” the environment and reduce it to a single aspect or theoretically predicted confluence of factors. Although there is a rich body of work on how heuristics are matched to particular decision environments ( Gigerenzer & Gaissmaier 2011 ), these environments are by necessity often highly stylized laboratory constructs aimed at exerting control over key features of the environment. 3 This line of work intentionally de-emphasizes or eliminates aspects of realistic social environments, which limits its obvious relevance for sociologists.
Finally, there is the challenge of data availability: sociologists typically do not observe the intermediate stages by which people arrive at decision outcomes. For example, researchers can fairly easily determine what college a person attended, what job they chose, or whom they married, but they rarely observe how they got to that decision—that is, how people learned about and evaluated available options, and which options were excluded either because they were infeasible or unacceptable. But such process data can be collected in a number of different ways, as detailed later in this article. Moreover, opportunities to study sociologically relevant decision processes are rapidly expanding, owing to the advent of disintermediated sources like the Internet and smart phones, which allow researchers to observe human behavior at a much finer level of temporal and geographic granularity than ever before. Equally important, these data often contain information on which options people considered , but ultimately decided against. Such activity data provide a rich source of information on sociologically relevant decision processes ( Bruch et al. 2016 ).
We believe that the time is ripe for a new line of work that draws on insights from cognitive science and decision theory to examine choice processes and how they play out in social environments. As we discuss in the next section, sociology and decision research offer complementary perspectives on decision-making and there is much to be gained from combining them. One benefit of this union is that it can deepen sociologists’ understanding of how and why individual outcomes differ across contexts. By leveraging insights on how contextual factors and aspects of choice problems influence decision strategies, sociologists can better pinpoint how, why, and when features of the social environment trigger and shape human behavior. This also presents a unique opportunity for cross-fertilization. While sociologists can draw from the choice literature’s rich understanding of and suite of tools to probe decision processes, work on decision-making can also benefit from sociologists’ insights into how social context enables or constrains behavior.
The literature on judgment and decision-making is enormous; our goal here is to offer a curated introduction aimed at social scientists new to this area. In addition to citing recent studies, we deliberately reference the classic and integrative literature in this field so that researchers can acquaint themselves with the works that introduced these ideas, and gain a comfortable overview to it. We highlight empirical studies of decision making that help address how people make critical life decisions, such as choosing a neighborhood, college, life partner, or occupation. Thus, our focus is on research that is relevant for understanding decision processes characterized by obscurity, where there is no obvious correct or optimal answer. Due to its selective nature, our review does not include a discussion of several major areas of the JDM literature, most notably Prospect Theory, which focuses on how people can distort both probabilities and outcome values when these are known (to the researcher) with certainty; we also do not discuss the wide range of anomalies documented in human cognition, for example mental accounting, the endowment effect, and biases such as availability or anchoring ( Tversky & Kahneman 1973 ; Kahneman & Tversky 1973 , 1981 ; Kahneman et al. 1991 ).
The balance of the article is as follows. We first explain how decision research emerged as a critique of rational choice theory, and show how these models of behavior complement existing work on action and decision-making in sociology. The core of the paper provides an overview of how cognitive, emotional, and contextual factors shape decision processes. We then introduce the data and methods commonly used to study choice processes. Decision research relies on a variety of data sources, including results from lab and field experiments, surveys, brain scans, and observations of in-store shopping and other behavior. We discuss their relative merits, and provide a brief introduction to statistical modeling approaches. We close with some thoughts about opportunities and challenges for sociologists wanting to incorporate insights and methods from the decision literature into their research programs.
SOCIOLOGICAL AND PSYCHOLOGICAL PERSEPCTIVES ON DECISION PROCESSES
To understand how sociology and psychology offer distinct but complementary views of decision processes, we begin with a brief introduction to the dominant model of human decision-making in the social sciences: rational choice theory. This model, endemic to neoclassical economic analyses, has permeated into many fields including sociology, anthropology, political science, philosophy, history, and law ( Coleman 1991 ; Gely & Spiller 1990 ; Satz & Ferejohn 1994 ; Levy 1997 ). In its classic form, the rational choice model of behavior assumes that decision makers have full knowledge of the relevant aspects of their environment, a stable set of preferences for evaluating choice alternatives, and unlimited skill in computation ( Samuelson 1947 ; Von Neumann & Morgenstern 2007 ; Becker 1993 ). Actors are assumed to have a complete inventory of possible alternatives of action; there is no allowance for focus of attention or a search for new alternatives ( Simon 1991 , p. 4). Indeed, a distinguishing feature of the classic model is its lack of attention to the process of decision-making. Preference maximization is a synonym for choice ( McFadden 2001 , p. 77).
Rational choice has a long tradition in sociology, but its popularity increased in the 1980s and 1990s, partly as a response to concern within sociology about the growing gap between social theory and quantitative empirical research ( Coleman 1986 ). Quantitative data analysis, despite focusing primarily on individual-level outcomes, is typically conducted without any reference to—let alone a model of—individual action ( Goldthorpe 1996 ; Esser 1996 ). Rational choice provides a theory of action that can anchor empirical research in meaningful descriptions of individuals’ behavior ( Hedström & Swedberg 1996 ). Importantly, the choice behavior of rational actors can also be straightforwardly implemented in regression-based models readily available in statistical software packages. Indeed while some scholars explicitly embrace rational choice as a model of behavior ( Hechter and Kanazawa 1997 ; Kroneberg and Kalter 2012 ), many others implicitly adopt it in their quantitative models of individual behavior.
Beyond Rational Choice
Sociologists have critiqued and extended the classical rational choice model in a number of ways. They have observed that people are not always selfish actors who behave in their own best interests ( England 1989 ; Margolis 1982 ), that preferences are not fixed characteristics of individuals ( Lindenberg and Frey 1993 ; Munch 1992 ), and that individuals do not always behave in ways that are purposive or optimal ( Somers 1989 ; Vaughan 1998 ). Most relevant to this article, sociologists have argued that the focus in classical rational choice on the individual as the primary unit of decision-making represents a fundamentally asocial representation of behavior. In moving beyond rational choice, theories of decision-making in sociology highlight the importance of social interactions and relationships in shaping behavior ( Pescosolido 1992 ; Emirbayer 1997 ). A large body of empirical work reveals how social context shapes people’s behavior across a wide range of domains, from neighborhood and school choice to decisions about friendship and intimacy to choices about eating, drinking, and other health-related behaviors ( Carrillo et al. 2016 ; Perna and Titus 2005 ; Small 2009 ; Pachucki et al. 2011 ; Rosenquist et al. 2010 ).
But This focus on social environments and social interactions has inevitably led to less attention being paid to the individual-level processes that underlie decision-making. In contrast, psychologists and decision theorists aiming to move beyond rational choice have focused their attention squarely on how individuals make decisions. In doing so, they have amassed several decades of work showing that the rational choice model is a poor representation of this process. 4 Their fundamental critique is that decision-making, as envisioned in the rational choice paradigm, would make overwhelming demands on our capacity to process information ( Bettman 1979 ; Miller 1956 ; Payne 1976 ). Decision-makers have limited time for learning about choice alternatives, limited working memory, and limited computational capabilities ( Miller 1956 ; Payne et al. 1993) As a result, they use heuristics that keep the information-processing demands of a task within the bounds of their limited cognitive capacity. 5 It is now widely recognized that the central process in human problem solving is to apply heuristics that carry out highly selective navigations of problem spaces ( Newell & Simon 1972 ).
However, in their efforts to zero in on the strategies people use to gather and process information, psychological studies of decision-making have focused largely on individuals in isolation. Thus, sociological and psychological perspectives on choice are complementary in that they each emphasize a feature of decision-making that the other field has left largely undeveloped. For this reason, and as we articulate further in the conclusion, we believe there is great potential for cross-fertilization between these areas of research. Because our central aim is to introduce sociologists to the JDM literature, we do not provide an exhaustive discussion of sociological work relevant to understanding decision processes. Rather, we highlight studies that illustrate the fruitful connections between sociological concerns and JDM research.
In the next sections, we discuss the role of different factors—cognitive, emotional, and contextual—in heuristic decision processes.
THE ROLE OF COGNITIVE FACTORS IN DECISION PROCESSES
There are two major challenges in processing decision-related information: first, each choice is typically characterized by multiple attributes, and no alternative is optimal on all dimensions; and, second, more than a tiny handful of information can overwhelm the cognitive capacity of decision makers ( Cowan 2010 ). Consider the problem of choosing among three competing job offers. Job 1 has high salary, but a moderate commuting time and a family-unfriendly workplace. Job 2 offers a low salary, but has a family-friendly workplace and short commuting time. Job 3 has a family-friendly workplace but a moderate salary and long commuting time. This choice would be easy if one alternative clearly dominated on all attributes. But, as is often the case, they all involve making tradeoffs and require the decision maker to weigh the relative importance of each attribute. Now imagine that, instead of three choices, there were ten, a hundred, or even a thousand potential alternatives. This illustrates the cognitive challenge faced by people trying to decide among neighborhoods, potential romantic partners, job opportunities, or health care plans.
We focus in this section on choices that involve deliberation, for example deciding where to live, what major to pursue in college, or what jobs to apply for. 6 (This is in contrast to decisions that are made more spontaneously, such as the choice to disclose personal information to a confidant [ Small & Sukhu 2016 ].) Commencing with the pioneering work of Howard & Sheth (1969) , scholars have accumulated substantial empirical evidence for the idea that such decisions are typically made sequentially , with each stage reducing the set of potential options ( Swait 1984 ; Roberts & Lattin 1991 , 1997 ). For a given individual, the set of potential options can first be divided into the set that he or she knows about, and those of which he or she is unaware. This “awareness set” is further divided into options the person would consider, and those that are irrelevant or unattainable. This smaller set is referred to as the consideration set , and the final decision is restricted to options within that set.
Research in consumer behavior suggests that the decision to include certain alternatives in the consideration set can be based on markedly different heuristics and criteria than the final choice decision (e.g., Payne 1976 ; Bettman & Park 1980 ; Salisbury & Feinberg 2012 ). In many cases, people use simple rules to restrict the energy involved in searching for options, or to eliminate options from future consideration. For example, a high school student applying to college may only consider schools within commuting distance of home, or schools where someone she knows has attended. Essentially, people favor less cognitively taxing rules that use a small number of choice attributes earlier in the decision process to eliminate almost all potential alternatives, but take into account a wider range of choice attributes when evaluating the few remaining alternatives for the final decision ( Liu & Dukes 2013 ).
Once the decision maker has narrowed down his or her options, the final choice decision may allow different dimensions of alternatives to be compensatory; in other words, a less attractive value on one attribute may be offset by a more attractive value on another attribute. However, a large body of decision research demonstrates that strategies to screen potential options for consideration are non-compensatory ; a decision-maker’s choice to eliminate from or include for consideration based on one attribute will not be compensated by the value of other attributes. In other words, compensatory decision rules are “continuous,” while non-compensatory decision rules are discontinuous or threshold ( Swait 2001 ; Gilbride & Allenby 2004 ).
Compensatory Decision Rules
The implicit decision rule used in statistical models of individual choice and the normative decision rule for rational choice is the weighted additive rule. Under this choice regime, decision-makers compute a weighted sum of all relevant attributes of potential alternatives. Choosers develop an overall assessment of each choice alternative by multiplying the attribute weight by the attribute level (for each salient attribute), and then sum over all attributes. This produces a single utility value for each alternative. The alternative with the highest value is selected, by assumption. Any conflict in values is assumed to be confronted and resolved by explicitly considering the extent to which one is willing to trade off attribute values, as reflected by the relative importance or beta coefficients ( Payne et al. 1993 , p. 24). Using this rule involves substantial computational effort and processing of information.
A simpler compensatory decision rule is the tallying rule, known to most of us as a “pro and con” list ( Alba & Marmorstein 1987 ). This strategy ignores information about the relative importance of each attribute. To implement this heuristic, a decision maker decides which attribute values are desirable or undesirable. Then she counts up the number desirable versus undesirable attributes. Strictly speaking, this rule forces people to make trade-offs among different attributes. However, it is less cognitively demanding than the weighted additive rule, as it does not require people to specify precise weights associated with each attribute. But both rules require people to examine all information for each alternative, determine the sums associated with each alternative, and compare those sums.
Non-Compensatory Decision Rules
Non-compensatory decision rules do not require decision makers to explicitly consider all salient attributes of an alternative, assign numeric weights to each attribute, or compute weighted sums in one’s head. Thus they are far less cognitively taxing than compensatory rules. The decision maker need only examine the attributes that define cutoffs in order to make a decision (to exclude options for a conjunctive rule, or to include them for a disjunctive one). The fewer attributes that are used to evaluate a choice alternative, the less taxing the rule will be.
Conjunctive rules require that an alternative must be acceptable on one or more salient attributes. For example, in the context of residential choice, a house that is unaffordable will never be chosen, no matter how attractive it is. Similarly, a man looking for romantic partners on an online dating website may only search for women who are within a 25-mile radius and do not have children. Potential partners who are unacceptable on either dimension are eliminated from consideration. So conjunctive screening rules identify “deal-breakers”; being acceptable on all dimensions is a necessary but not sufficient criterion for being chosen.
A disjunctive rule dictates that an alternative is considered if at least one of its attributes is acceptable to chooser i. For example, a sociology department hiring committee may always interview candidates with four or more American Journal of Sociology publications, regardless of their teaching record or quality of recommendations. Similarly (an especially evocative yet somewhat fanciful example), a disjunctive rule might occur for the stereotypical “gold-digger” or “gigolo,” who targets all potential mates with very high incomes regardless of their other qualities. Disjunctive heuristics are also known as “take-the-best” or “one good reason” heuristics that base their decision on a single overriding factor, ignoring all other attributes of decision outcomes ( Gigerenzer & Goldstein 1999 ; Gigerenzer & Gaissmaier 2011 ; Gigerenzer 2008 ).
While sociologists studying various forms of deliberative choice do not typically identify the decision rules used, a handful of empirical studies demonstrate that people do not consider all salient attributes of all potential choice alternatives. For example, Krysan and Bader (2009) find that white Chicago residents have pronounced neighborhood “blind spots” that essentially restrict their knowledge of the city to a small number of ethnically homogeneous neighborhoods. Daws and Brown (2002 Daws and Brown (2004) find that, when choosing a college, UK students’ awareness and choice sets differ systematically by socioeconomic status. Finally, in a recent study of online mate choice, Bruch and colleagues (2016) build on insights from marketing and decision research to develop a statistical model that allows for multistage decision processes with different (potentially noncompensatory) decision rules at each stage. They find that conjunctive screeners are common at the initial stage of online mate pursuit, and precise cutoffs differ by gender and other factors.
THE ROLE OF EMOTIONAL FACTORS IN DECISION PROCESSES
Early decision research emphasized the role of cognitive processes in decision-making (e.g., Newell & Simon 1972 ). But more recent work shows that emotions—not just strong emotions like anger and fear, but also “faint whispers of emotions” known as affect ( Slovic et al. 2004 , p. 312)—play an important role in decision-making. Decisions are cast with a certain valence, and this shapes the choice process on both conscious and unconscious levels. In other words, even seemingly deliberative decisions, like what school to attend or job to take, may be made not just through careful processing of information, but based on intuitive judgments of how a particular outcome feels ( Loewenstein & Lerner 2003 ; Lerner et al. 2015 ). This is true even in situations where there is numeric information about the likelihood of certain events ( Denes-Raj & Epstein 1994 ; Windschitl & Weber 1999 ; Slovic et al. 2000 ). This section focuses on two topics central to this area: first, that people dislike making emotional tradeoffs, and will go to great lengths to avoid them; and second, how emotional factors serve as direct inputs into decision processes. 7
Emotions Shape Strategies for Processing Information
In the previous section, we emphasized that compensatory decision rules that involve tradeoffs require a great deal of cognitive effort. But there are other reasons why people avoid making explicit tradeoffs on choice attributes. For one, some tradeoffs are more emotionally difficult than others, for example the decision whether to stay at home with one’s children or put them in day care. Some choices also involve attributes that are considered sacred or protected ( Baron & Spranca 1997 ). People prefer not to make these emotionally difficult tradeoffs, and that shapes decision strategy selection ( Hogarth 1991 ; Baron 1986 ; Baron & Spranca 1997 ). Experiments on these types of emotional decisions have shown that, when facing emotionally difficult decisions, decision-makers avoid compensatory evaluation and instead select the alternative that is most attractive on whatever dimension is difficult to trade off ( Luce et al. 2001 ; Luce et al. 1999 ). Thus, the emotional valence of specific options shapes decision strategies.
Emotions concerning the set of all choice alternatives—specifically, whether they are perceived as overall favorable or unfavorable—also affects strategy selection. Early work with rats suggests that decisions are relatively easy when choosing between two desirable options with no downsides ( Miller 1959 ). However, when deciding between options with both desirable and undesirable attributes, the choice becomes harder. When deciding between two undesirable options, the choice is hardest of all. Subsequent work reveals that this finding extends to human choice. For instance, people invoke different choice strategies when forced to choose “the lesser of two evils.” In their experiments on housing choice, Luce and colleagues (2000) found that when faced with a set of substandard options, people are far more likely to engage in “maximizing” behavior and select the alternative with the best value on whatever is perceived as the dominant substandard feature. In other words, having a suboptimal choice set reduces the likelihood of tradeoffs on multiple attributes. Extending this idea to a different sociological context, a woman confronted with a dating pool filled with what she perceives as arrogant men may focus her attention on selecting the least arrogant of the group.
Emotions as Information
Emotions also serve as direct inputs into the decision process. A large body of work on perceptions of risk shows that a key way people evaluate the risks and benefits of a given situation is through their emotional response ( Slovic et al. 2004 ; Slovic and Peters 2006 ; Loewenstein et al. 2001 ). In a foundational and generative study, Fischhoff et al. (1978) discovered that people’s perceptions of risks decline as perceived benefits increase. This is puzzling, because risks and benefits tend to be positively correlated. The authors also noted that the attribute most highly correlated with perceived risk was the extent to which the item in question evoked a feeling of dread. This finding has been confirmed in many other studies (e.g., McDaniels et al. 1997 ). Subsequent work also showed that this inverse relationship is linked to the strength of positive or negative affect associated with the stimulus. In other words, stronger negative responses led to perception of greater risk and lower benefits ( Alhakami & Slovic 1994 ; Slovic & Peters 2006 ).
This has led to a large body of work on the affect heuristic , which is grounded in the idea that people have positive and negative associations with different stimuli, and they consult this “affect pool” when making judgments. This shortcut is often more efficient and easier than cognitive strategies such as weighing pros and cons or even disjunctive rules for evaluating the relative merits of each choice outcome ( Slovic et al. 2004 ). Affect— particularly how it relates to decision-making—is rooted in dual process accounts of human behavior. The basic idea is that people experience the world in two different ways: one that is fast, intuitive, automatic, and unconscious, and another that is slow, analytical, deliberate, and verbal ( Evans 2008 ; Kahneman 2011 ). A defining characteristic of the intuitive, automatic system is its affective basis ( Epstein 1994 ). Indeed, affective reactions to stimuli are often the very first reactions people have. Having determined what is salient in a given situation, affect thus guides subsequent processes, such as information processing, that are central to cognition ( Zajonc 1980 ).
Over the past two decades, sociologists—particularly in the study of culture—have incorporated insights from dual process theory to understand how actions may be both deliberate and automatic (e.g., Vaisey 2009 ). Small and Sukhu (2016) argue that dual processes may play an important role in the mobilization of support networks. Kroneberg and Esser ( Kroneberg 2014 ; Esser and Kroneberg 2015 ) explore how automatic and deliberative processes shape how people select the “frame” for making sense of a particular situation. Although some scholars debate whether automatic and deliberative processes are more like polar extremes or a smooth spectrum (for an example of this critique within sociology, see Leschziner and Green 2013 ), the dual process model remains a useful framework for theorizing about behavior.
THE ROLE OF CONTEXTUAL FACTORS IN DECISION PROCESSES
Sociologists have long been interested in how social environments—for example, living in a poor neighborhood, attending an affluent school, or growing up in a single-parent household—shape life outcomes such as high school graduation, non-marital fertility, and career aspirations ( Sharkey and Faber 2014 ; Lee et al. 1993 ; Astone and McLanahan 1991 ). Social environments shape behavior directly through various forms of influence such as peer pressure and social learning, and indirectly by dictating what opportunities or social positions are available ( Blalock 1984 ; Manski 2000 ; Schelling 1971 ). But while the sociological literature on contextual effects is vast, the subset of that work which focuses on decisions emphasizes the causes or consequences of those decisions more than the processes through which they are made.
Decision researchers devote considerable attention to contextual effects, but typically “context” in this field refers to architectural features of choice environments such as the number of alternatives; whether time pressures limit the effort that can be put into a decision; and what option is emphasized as the default. (In the world, of course, these features are socially determined. But this is less emphasized in decision research, much of which occurs in a laboratory setting.) The overwhelming finding from these studies is that people’s choices are highly sensitive to context. This insight has led to an influential literature on the “Construction of Preferences” (see Sidebar) as well as a great deal of interest in policy interventions that manipulate features of choice environments ( Thaler and Sunstein 2008 ; Shafir 2013 ). Recently, decision researchers have begun to look at how decisions are shaped by more explicitly social environments such as poverty (e.g., Mullainathan and Shafir 2013 ). In this section, we discuss how four aspects of social context—what opportunities are available, the importance of the “default” option, time pressure and constrained resources, and the choices of others—shape decision processes.
Choice Sets and Defaults
A classic assumption of conventional choice models is that the ratio of choice probabilities for any two options is independent of what other options are available ( Luce 1959 ). (In the literature on statistical models of choice, this is known as the principle of Independence of Irrelevant Alternatives [IIA].) But it is well established that people’s choices depend heavily on the relative merits of a particular set of options rather than their absolute values ( Tversky & Simonson 1993 ). For example, people tend to avoid more extreme values in alternatives (the “compromise effect”); thus, adding a new option to the mix can lead choosers to shift their views about what constitutes a reasonable choice ( Simonson 1989 ; Simonson & Tversky 1992 ). In a similar vein, a robust finding is that adding a new “asymmetrically dominated” alternative – one dominated by some items in the set but not by others – can actually increase the choice probabilities of the items that dominate it. Such a “decoy effect” ( Huber et al. 1982 ) should be impossible under IIA, and in fact violates regularity (i.e., new items cannot increase probabilities of existing ones). Both of these effects have been attributed to the fact that people making choices are trying to justify them based on reasons ( Simonson 1989 ; Dhar et al. 2000 ); changing the distribution of options may alter how compelling a particular reason might be.
Choice outcomes are also highly influenced by what option is identified as the “default.” Defaults are whatever happens in a decision if the chooser “decides not to decide” ( Feinberg & Huber 1996 ). Defaults exert a strong effect on people’s choices, even when the stakes of the decision are high ( Johnson et al. 1993 ; Johnson & Goldstein 2013 ). Defaults also tap into other, well-established features of human decision making: procrastination, bias for the status quo, and inertial behavior ( Samuelson & Zeckhauser 1988 ; Kahneman et al. 1991 ). In recent years, manipulating the default option—for example making retirement savings or organ donation something people opt out of rather than opt into—has been identified as a potentially low cost, highly impact policy intervention ( Shafir 2013 ). Defaults are also of potentially great sociological interest. A number of sociological studies have theorized about how people “drift” into particular outcomes or situations (e.g., Matza 1967 ); defaults exist in part because choices are embedded in specific environments that emphasize one set of options over others. 8
Scarcity and Social Influence
A number of recent studies examine how conditions of scarcity— with regard to time, resources, and energy—shape decision-making. Consistent with studies of cognitive effort and decision-making, a variety of experimental results demonstrate that time pressure reduces people’s tendency to make tradeoffs ( Wright & Weitz 1977 ; Edland 1989 ; Rieskamp & Hoffrage 2008 ). This finding is especially interesting in light of recent line of work by Shah et al. (2015) , who show via experiments that resource scarcity forces people to make tradeoffs, e.g., “If I buy dessert, I can’t afford to take the bus home.” Weighing tradeoffs is cognitively costly; they deplete people’s resources for other decision tasks, which overall reduces their ability to engage in deliberative decision-making ( Pocheptsova et al. 2009 ). Given the fact that people living in conditions of extreme scarcity are typically limited in both time and financial resources, a cognitive perspective suggests that they are “doubly taxed” in terms of cognitive effort, and offers important insights in understanding how conditions of poverty shape, and are shaped by, people’s choices ( Bertrand et al. 2004 ). In short, the context of poverty depletes people’s cognitive resources in an environment where mistakes are costly ( Mullainathan & Shafir 2013 , Gennetian & Shafir 2015 ).
There are also a small number of studies that examine how the actions of other people influence decision processes. They focus on the role of descriptive norms ( Cialdini 2003 ; Cialdini & Goldstein 2004 ), which are information about what other people are doing. The key finding is that people are more likely to adopt a particular behavior—such as conserving energy in one’s home or avoiding changing sheets and towels at a hotel—if they learn that others are doing the same ( Goldstein et al. 2008 ; Nolan et al. 2008 ). The more similar the comparison situation is to one’s own, the more powerful the effect of others’ behavior on one’s own. For instance, people are more likely to be influenced if the descriptive norm references their neighbors or others who share social spaces ( Schultz et al. 2007 ). The finding that descriptive norms are most powerful when they are immediate is reinforced by a study of charitable giving, which shows that people’s behavior is disproportionately influenced by information about what others have given, especially the most recent, non-specified donor ( Shang & Croson 2009 ).
This work on social influence is consistent with a classic literature in sociology that emphasizes how people’s beliefs about the sort of situation they are in shape their behavior (e.g., Thomas & Znaniecki 1918 ; Goffman 1974 ). Social cues provide information about what choices are consistent with desired or appropriate behavior. For example, a classic study demonstrates that whether a Prisoner’s dilemma game was presented to research subjects as a simulated “Wall Street” or “Community” shaped subsequent playing decisions ( Liberman et al. 2004 ; Camerer & Thaler 1993 ). In other words, there is an interpretive component to decision-making that informs one’s views about the kind of response that is appropriate.
STUDYING DECISION PROCESSES
Psychologists have devised a number of techniques to shed light on human decision processes in conjunction with targeted stimuli. Process tracing is a venerated suite of methods broadly aimed at extracting how people go about acquiring, integrating, and evaluating information, as well as physiological, neurological, and concomitants of cognitive processes ( Svenson 1979 ; Schulte-Mecklenbeck et al. 2010 ). (Note that this approach is quite different from what political scientists and sociologists typically refer to as process tracing [ Mahoney 2012 ; Collier 2011 ].) In a classic study, Lohse & Johnson (1996) examine individual-level information acquisition using both computerized tracing and eye-tracking across multiple process measures (e.g., total time, number of fixations, accuracy, etc.)
More recently, the use of unobtrusive eye-trackers has allowed researchers to discern which information is being sought and assimilated in the sort of stimuli-rich environments that typify online interactions, without querying respondents’ knowledge or intermediate goals ( Duchowski 2007 , Wedel & Pieters 2008 ). Also, it has recently become possible to use neuroimaging techniques like PET, EEG, and fMRI ( Yoon et al. 2006 ) to observe decision processes in vivo , although at a high cost of invasiveness. Such studies offer the benefit of sidestepping questions about, for example, the emotional reactions experienced by decision-makers, by observing which portions of the brain are active when information is being accessed and processed, as well as final decisions arrived at.
Stated and Revealed Preferences
While not directly focused on the process of decision-making (i.e., in terms of identifying decision strategies), a large literature assumes a linear compensatory model and aims to capture the weights people ascribe to different choice attributes (see Louviere et al. 1999 for a broad overview; also Train 2009 ). These methods, long known to social scientists ( Samuelson 1948 ; Manski and McFadden 1981 ; Bruch and Mare 2012 ), rely on both field data—where the analyst records decision-makers’ “revealed preferences” as reflected in their actions—and choice experiments, where analysts enact control over key elements of the decision environment through vignettes. Stated choice experiments have two advantages that are relevant in modeling choice processes: their ability to (1) present decision-makers with options unavailable in practice or outside their usual purview; and (2) record multiple (hypothetical) choices for each decision-maker, even for scenarios like mate choice or home purchase that are made few times in a lifespan. The downside is that they are difficult to fully contextualize or make incentive-compatible; for example, experiment participants are routinely more willing to spend simulated experimental money than their own hard-won cash ( Carlsson & Martinsson 2001 ).
Among the main statistical methods for enacting choice experiments is conjoint analysis ( Green and Srinivasan 1978 , Green et al. 2001 ), a broad suite of techniques, implemented widely in dedicated software (e.g., Sawtooth), to measure stated preferences and how they vary across a target population. Conjoint works by decomposing options into their attributes , each of which can have several levels. For example, housing options each provide cooking facilities, sleeping quarters, bathrooms, among other attributes, and each of these varies in terms of their quality levels (e.g., larger vs. smaller; number overall; and categorical attributes like type of heating, color, location, etc.) The goal of conjoint is to assign a utility or part-worth to each level of each attribute, with higher numbers representing more preferred attribute levels; for example, one might say that, for families with several children, a home with four bedrooms has a much higher utility than one with two. Conjoint approaches can be—and have been ( Wittink & Cattin 1989 ; Wittink et al. 1994 )—applied to a wide range of settings where it is useful to measure the importance of specific choice attributes.
Field data such as residential, work, or relationship histories have the advantage of reflecting actual choices made in real contexts ( Louviere et al. 1999 ). However, such data suffer from several drawbacks: (1) variables necessarily covary (i.e., higher neighborhood prices correlate with levels of many attributes at once); (2) we cannot infer how people would respond to possible novel options, like affordable, diverse neighborhoods that may not yet exist; and (3) each person typically makes just one choice. Such problems are exacerbated when researchers cannot in principle know the entire “consideration set” of options available and actively mulled over by each decision-maker, which is typically the case in sociological applications. 9 When such confounds are present in field data, conjoint and other experimental methods allow researchers to control and “orthogonalize” the attributes and levels used in choice experiments, to achieve maximal efficiency and avoid presenting each participant with more than a couple of dozen hypothetical choice scenarios ( Chrzan & Orme 2000 ). 10
Two other choice assessment methods deserve brief mention, as they leverage some of the best features of experimental and field research: natural experiments and field experiments. In a natural experiment, an event exogenous to the outcome of interest affects only certain individuals, or affects different individuals to varying degrees. One example is the effect of natural disasters, like the 2005 flooding of New Orleans in the aftermath of Hurricane Katrina, on migration decisions (Kirk 2009). In field experiments, researchers exert control over focal aspects of people’s choice environments. Although it may seem difficult to study sociologically relevant choice processes this way, the use of web sites as search tools enables a nontrivial degree of experimental control over choice environments (see, for example, Bapna et al. 2016).
Statistical Models of Choice Processes
The bedrock formulation of statistical analyses of choice is the random utility model , which posits that each option available to a decision-maker affords a certain value (“utility”), which can be decomposed into that explicable by the analyst and a (“random”) error ( Ben-Akiva & Lerman 1985 ; Train 2009 ). 11 The former can be related through regression-based techniques to other observed covariates on the options, decision-maker, and environment, whereas the latter can be due to systematically unavailable information (e.g., income or education) or intrinsic unobservables (e.g., the mood of the decision-maker). Of particular importance is McFadden’s conditional logit model ( McFadden 1973 ), 12 which allows attributes of options to vary across choice occasions (e.g., prices or waiting times for different transportation modes; neighborhoods or room sizes for renting vs. buying a home; etc.) Because this model is supported in much commercial statistical software, and converges rapidly even for large data sets, it is by far the most widely deployed in choice applications.
Statistical models of choice have been grounded primarily in rational utility theory, with concessions towards efficient estimation. As such, the utility specifications underlying such models have tended to be linear, to include all available options, and incorporate full information on those options. Much research reveals not only the psychological process accuracy, but the statistical superiority, of relaxing these assumptions by incorporating limits on information, cognitive penalties, and nonlinear/noncompensatory utility. For example, the formal incorporation of consideration sets into choice modeling ( Horowitz, & Louviere 1995 ; Louviere, Hensher, & Swait 2000 , section 10.3) has demonstrated superior fit and predictive accuracy for explicit models of exclusion of certain options from detailed processing, with some analyses (see Hauser & Wernerfelt 1990 ) attributing in excess of 75% of the choice model’s fit to such restrictions. Similarly, lab studies have confirmed that decision-makers wish to conserve cognitive resources, and formal statistical models (e.g., Shugan 1980 , Roberts & Lattin 1991 ) have attempted to account for and measure a “cost of thinking” or consideration from real-world data.
However, despite a decades-deep literature on noncompensatory evaluation processes ( Einhorn 1970 ), the practical estimation of such models is largely in its infancy, due to complexities of specification and data needs (e.g., Elrod et al. 2004 ). Although nonlinearity can be captured using polynomial functions of covariates, these impose smoothness of response; by contrast, conjunctive and disjunctive processes impose cutoffs beyond which evaluation is either wholly positive or insurmountably negative ( Bruch et al. , 2016 ). We view the development of this area as critical to the widespread acceptance of formal choice models to social scientists and sociologists in particular, who typically wish to know which neighborhoods we would never live in, jobs we would never take, etc.
CHALLENGES AND OPPORTUNITIES FOR FUTURE RESEARCH
Sociology has long been interested in individuals’ choices and their implications for social life, and there is renewed interest in theories that can explain human action (e.g., Kroneberg 2014 ; Gross 2009 ). Our hope is that this review enables interested scholars to pursue a more nuanced and structurally accurate representation of the choice process. Greater insight into human behavior will also allow for greater insights into the dynamic relationship between micro- and meso-level processes and their larger-scale implications ( Hedstrom and Bearman 2009 ). Consider how choice sets are constructed in the first place. People may rule out certain options due to preferences, affordability and/or time constraints—classic cognitive, temporal, and economic variables—but also the anticipation of unfair treatment or discrimination. For example, a high school student searching for colleges may eliminate those that are too expensive, too far from home, or are perceived as unwelcoming. Identifying the criteria through which people rule themselves (or others) out can illuminate more precise mechanisms through which people’s actions shape, and are shaped by, larger-scale inequality structures.
We also believe that many of the data limitations that hampered sociologists’ ability to study decision processes in the past are becoming far less of an issue. This is due at least in part to increasingly available data sources that can aid sociologists in studying choice processes. These so-called “big data” are often behavioral data: specific actions taken by individuals that can shed light on processes of information search, assimilation, and choice. Moreover, the online environments through which we increasingly communicate and interact enable not only observational “field” data, but also targeted, unobtrusive experiments where the decision environment is directly altered. The latter possibility offers greater precision than ever before in isolating both idiosyncratic and potentially universal features of behavior, as well as better understanding the interplay between context and human action.
However, existing approaches from marketing and psychology are often not suited to sociological inquiry directly out of the box, creating both challenges and opportunities for future work. For example, most extant models were designed to capture more prosaic decisions, like supermarket shopping, where attributes are known, options are stable, and “stakes” are modest. Although some choices of sociological interest may fit this pattern, most do not. For example, many pivotal life decisions—purchasing a home (where sellers and buyers must agree on terms); college admissions; dating and marriage decisions; employment offers—require a partnership , wherein each decision maker must “choose you back.” Sociologists must therefore be circumspect in applying models developed for choice among “inert” options (such as flavors of yogurt) to the data they most commonly analyze.
The fact that so many sociological choices are characterized by obscurity (i.e., there is no obvious or single optimal choice to be made) is also a good reason to proceed with caution in applying ideas from JDM to sociological research. Take, for example, the literature discussed earlier on interventions that manipulate people’s default option. It is one thing to encourage healthier eating by putting apples before chocolate cake in the cafeteria line; it is quite another to nudge someone towards a particular neighborhood or school. Sociologists rarely have a clear sense of what choice will be optimal for a group of people, let alone particular individuals. On the other hand, few would argue that growing up surrounded by violence isn’t universally harmful (Harding 2009; Sharkey et al. 2013). Policies can only be improved by a more nuanced understanding of how choices unfold in particular environments, and how the default options are shaped by contextual factors.
Thus, this challenge also creates the opportunity to build knowledge on how heuristics operate under conditions of obscurity. JDM research has largely focused on documenting whether and how heuristics fall short of some correct or optimal answer. In sociology, by contrast, we typically lack a defensible metric for the “suboptimality” of decisions. While some decisions may be worse than others, it is impossible to know, for example, whether one has chosen the “right” spouse or peer group, or how to set up appropriate counterfactual scenarios as yardsticks against which specific decisions could be dispassionately assessed. By branching into decision domains where the quality or optimality of outcomes cannot be easily quantified (or in some cases even coherently conceptualized), sociologists can not only actively extend the range of applications of decision research, but also break new theoretical ground.
SIDEBAR 1: THE CONSTRUCTION OF PREFERENCES.
Decision researchers have amassed several lines of evidence to suggest that, rather than being stable constructs that are retrieved on demand, preferences are constructed in the moment of elicitation ( Lichtenstein & Slovic 2006 ; Bettman, Luce, and Payne 1998 ). This finding is echoed in studies of judgments and attitudes, which are also sensitive to contextual cues ( Ross and Nisbett 1991 ; Schwarz 1999 , 2007 ). Preference variation can be generated from simple anchors or changes in question wording (e.g., Mandel & Johnson 2002 ). A classic finding is that people exhibit preference reversals when making multiattribute choices; in one context they indicate a preference of A over B, but in another context they indicate they prefer B over A (e.g., Cox & Grether 1996 ; Seidl 2002 ). Several theories have been put forward to explain this effect (see Lichtenstein & Slovic 2006 , Chapter 1 for a review and synthesis). One explanation is that people’s preferences, attitudes, judgments reflect what comes to mind; and what is salient at one time point may not be salient at another ( Higgins 1996 ; Schwarz, Strack, and Mai 1991 ).
Acknowledgments
We are grateful to Scott Rick Mario Small, and an anonymous reviewer for helpful feedback on this manuscript. This work was supported by a training grant (K01-HD-079554) from the National Institute of Child and Human Developent
A notable exception is Herb Simon’s concept of “satisficing,” which many influential sociological works—especially in the subfield of economic sociology—have incorporated into models of action and behavior (e.g., Baker 1984 ; Granovetter 1985 ; Uzzi 1997 ; Beckert 1996 ).
Although rational choice has had a strong influence sociological research—for example, Coleman’s (1994) Foundations of Social Theory has almost 30,000 citations and there is a journal, Rationality and Society , devoted to related topics—this framework never overtook the discipline as it did economics.
While this is accurate as a broad characterization, there are studies that examine decision-making “in the wild” through observation or field experiments (e.g., Camerer 2004 ; Barberis 2013 ).
The best-known critique of the rational choice model within JDM comes from the “Heuristics and Biases” school of research (Tversky 1972; Kahneman & Tversky 1979 ; Tversky & Kahneman 1981 ). Their studies show that decision makers: (1) have trouble processing information; (2) use decision-making heuristics that do not maximize preferences; (3) are sensitive to context and process; and (4) systematically misperceive features of their environment. Since then, a large body of work provides convincing evidence that individuals are “limited information processing systems” ( Newell & Simon 1972 ; Kahneman 2003 ).
Heuristics are “problem-solving methods that tend to produce efficient solutions to difficult problems by restricting the search through the space of possible solutions, on the basis of some evaluation of the structure of the problem” ( Braunstein 1972 , p. 520).
The decision strategies presented in this section are sometimes known as “reasons” heuristics (c.f., Gigerenzer 2004 ) because they are the reasons people give for why they chose the way that they did. As such, they are less applicable in situations where people have few if any options. For example, a person evicted from their home may have a single alternative to homelessness: staying with a family member. In this case, the difficulty of the decision is not information processing.
There is a rich literature in sociology on how emotions are inputs to and outcomes of social processes (e.g., Hochschild 1975; Scheff 1988 ). While this work has not historically been integrated with psychology ( Turner and Stets 2014 , p. 2), this may be a fruitful direction for future research.
We are grateful for conversations with Rob Sampson and Mario Small that led to this insight.
Newer online data sources—for example, websites for housing search and dating—generate highly granular, intermediate data on consideration sets. This parallels a revolution that occurred among choice modelers in marketing 30 years ago: the introduction of in-store product code scanners and household panels whose longitudinal histories provided information not only on which options were eventually chosen, but also which were actually available at the time of purchase, but rejected.
A review of the vast choice experiment design literature is beyond the scope of this article, but turnkey solutions for designing, deploying, and estimating discrete choice models for online panels are commercially available (e.g., Sawtooth Software’s “Discover”).
There is an enormous literature on the analysis of discrete choice data—items chosen from a known array with recorded attributes. Our treatment here is necessarily brief and highly selective. For more comprehensive introductions to this topic, we refer the reader to the many treatments available (e.g., Ben-Akiva & Lerman 1985 , Hensher et al. 2005 , Louviere et al. 2000 , Train 2009 ).
Empirical work typically refers to this as the multinomial logit model, although economists often distinguish the latter as applicable to when attributes change for the decision-maker (e.g., age, income), not the options themselves.
Contributor Information
Elizabeth Bruch, Department of Sociology and Complex Systems.
Fred Feinberg, Ross School of Business and Statistics.
LITERATURE CITED
- Alba J, Marmorstein H. The effects of frequency knowledge on consumer decision making. Journal of Consumer Research. 1987;14:14–25. [ Google Scholar ]
- Alhakami A, Slovic P. A psychological study of the inverse relationship between perceived risk and perceived benefit. Risk analysis. 1994;14:1085–1096. doi: 10.1111/j.1539-6924.1994.tb00080.x. [ DOI ] [ PubMed ] [ Google Scholar ]
- Aribarg A, Arora N, Bodur H. Understanding the role of preference revision and concession in group decisions. Journal of Marketing Research. 2002;39:336–349. [ Google Scholar ]
- Astone N, McLanahan S. Family structure, parental practices and high school completion. American Sociological Review. 1991;56:309–320. [ Google Scholar ]
- Baker W. The social structure of a national securities market. American Journal of Sociology. 1984;89:775–811. [ Google Scholar ]
- Barberis N. Thirty years of prospect theory in economics: A review and assessment. The Journal of Economic Perspectives. 2013;27:173–95. [ Google Scholar ]
- Baron J, Spranca M. Protected values. Organizational behavior and human decision processes. 1997;70:1–16. doi: 10.1006/obhd.1999.2839. [ DOI ] [ PubMed ] [ Google Scholar ]
- Baron J. Tradeoffs among reasons for action. Journal for the theory of social behavior. 1986;16:173–195. [ Google Scholar ]
- Beckert J. What is sociological about economic sociology? Uncertainty and the embeddedness of economic action. Theory and Society. 1996;25:803–840. [ Google Scholar ]
- Ben-Akiva M, Lerman S. Discrete Choice Analysis: Theory and Application to Travel Demand. Cambridge: MIT Press; 1985. [ Google Scholar ]
- Bertrand M, Mullainathan S, Shafir E. A behavioral-economics view of poverty. The American Economic Review. 2004;94:419–423. [ Google Scholar ]
- Bettman J, Luce M, Payne J. Constructive consumer choice processes. Journal of Consumer Research. 1998;25:187–217. [ Google Scholar ]
- Bettman J, Park C. Effects of prior knowledge and experience and phase of the choice process on consumer decision processes: A protocol analysis. Journal of Consumer Research. 1980;7:234–248. [ Google Scholar ]
- Blalock H. Contextual-effects models: theoretical and methodological issues. Annual Review of Sociology. 1984;10:353–372. [ Google Scholar ]
- Boudon R. Beyond rational choice theory. Annual Review of Sociology. 2003;29:1–21. [ Google Scholar ]
- Braunstein M. Perception of rotation in depth: A process model. Psychological Review. 1972;79:510–524. doi: 10.1037/h0033459. [ DOI ] [ PubMed ] [ Google Scholar ]
- Brownstone D, Bunch D, Train K. Joint mixed logit models of stated and revealed preferences for alternative-fuel vehicles. Transportation Research Part B: Methodological. 2000;34:315–338. [ Google Scholar ]
- Bruch E, Feinberg F, Lee K. Extracting Multistage Screening Rules from Online Dating Activity Data. Published online before print at Proceedings of the National Academy of Sciences. 2016 doi: 10.1073/pnas.1522494113. [ DOI ] [ PMC free article ] [ PubMed ] [ Google Scholar ]
- Camerer C, Weber M. Recent developments in modeling preferences: Uncertainty and ambiguity. Journal of Risk and Uncertainty. 1992;5:325–370. [ Google Scholar ]
- Camerer C, Thaler R. Anomalies: Ultimatums, dictators and manners. The Journal of Economic Perspectives. 1995;9:209–219. [ Google Scholar ]
- Camerer C, Loewenstein G. Behavioral economics: Past, present, future. In: Camerer C, Loewenstein G, Rabin M, editors. Advances in behavioral economics. Princeton: Princeton University Press; 2004. pp. 3–51. [ Google Scholar ]
- Camerer C. Prospect theory in the wild: Evidence from the field. In: Camerer C, Loewenstein G, Rabin M, editors. Advances in behavioral economics. Princeton: Princeton University Press; 2004. pp. 148–61. [ Google Scholar ]
- Carrillo L, Pattillo M, Hardy E, Acevedo-Garcia D. Housing Decisions Among Low-Income Hispanic Households in Chicago. Cityscape. 2016;18:109–137. [ Google Scholar ]
- Carlsson F, Martinsson P. Do hypothetical and actual marginal willingness to pay differ in choice experiments? Application to the valuation of the environment. Journal of Environmental Economics and Management. 2001;41:179–192. [ Google Scholar ]
- Cerulo K. Culture in mind: Toward a sociology of culture and cognition. New York: Routledge; 2002. [ Google Scholar ]
- Chrzan K, Orme B. An overview and comparison of design strategies for choice-based conjoint analysis. Sawtooth software research paper series 2000 [ Google Scholar ]
- Cialdini R. Crafting normative messages to protect the environment. Current Directions in Psychological Science. 2003;12:105–109. [ Google Scholar ]
- Cialdini R, Goldstein N. Social influence: Compliance and conformity. Annual Review of Psychology. 2004;55:591–621. doi: 10.1146/annurev.psych.55.090902.142015. [ DOI ] [ PubMed ] [ Google Scholar ]
- Coleman J. Foundations of Social Theory. Cambridge: Harvard University Press; 1994. [ Google Scholar ]
- Coleman J. Social theory, social research, and a theory of action. American journal of Sociology. 91:1309–1335. [ Google Scholar ]
- Collier D. Understanding process tracing. Political Science & Politics. 2011;44:823–830. [ Google Scholar ]
- Cowan N. The magical mystery four how is working memory capacity limited, and why? Current directions in psychological science. 2010;19:51–57. doi: 10.1177/0963721409359277. [ DOI ] [ PMC free article ] [ PubMed ] [ Google Scholar ]
- Cox J, Grether D. The preference reversal phenomenon: Response mode, markets and incentives. Economic Theory. 1996;7:381–405. [ Google Scholar ]
- Dawes P, Brown J. Determinants of awareness, consideration, and choice set size in university choice. Journal of Marketing for Higher Education. 2002;12:49–75. [ Google Scholar ]
- Dawes P, Brown J. The composition of consideration and choice sets in undergraduate university choice: An exploratory study. Journal of Marketing for Higher Education. 14:37–59. [ Google Scholar ]
- Dhar R, Nowlis S, Sherman S. Trying hard or hardly trying: An analysis of context effects in choice. Journal of Consumer Psychology. 2000;9:189–200. [ Google Scholar ]
- Denes-Raj V, Epstein S. Conflict between intuitive and rational processing: when people behave against their better judgment. Journal of personality and social psychology. 1994;66:819. doi: 10.1037//0022-3514.66.5.819. [ DOI ] [ PubMed ] [ Google Scholar ]
- Dimaggio P. Culture and Cognition. Annual Review of Sociology. 1997;23:263–287. [ Google Scholar ]
- Duchowski A. Eye tracking methodology: Theory and Practice. Springer Science & Business Media; 2007. [ Google Scholar ]
- Edland A. On cognitive processes under time stress: A selective review of the literature on time stress and related stress. Department [Psykologiska inst., Stockholms univ.]; 1989. [ Google Scholar ]
- Einhorn H. The use of nonlinear, noncompensatory models in decision-making. Psychological bulletin. 1970;73:221. doi: 10.1037/h0028695. [ DOI ] [ PubMed ] [ Google Scholar ]
- Einhorn H, Hogarth R. Decision making under ambiguity: A note. In: Munier B, editor. Risk, Decision and Rationality. Dordrecht, Holland: Reidel; 1988. pp. 327–336. [ Google Scholar ]
- Ellsberg D. Risk Ambiguity, and the Savage Axioms. Quarterly Journal of Economics. 1961;75:643–669. [ Google Scholar ]
- Elrod T, Johnson R, White J. A new integrated model of noncompensatory and compensatory decision strategies. Organizational Behavior and Human Decision Processes. 2004;95:1–19. [ Google Scholar ]
- Emirbayer M. Manifesto for a relational sociology. American Journal of Sociology. 1997;103:281–317. [ Google Scholar ]
- England P. A feminist critique of rational-choice theories: Implications for sociology. The American Sociologist. 1989;20:14–28. [ Google Scholar ]
- Epstein S. Integration of the cognitive and the psychodynamic unconscious. American Psychologist. 1994;49:709. doi: 10.1037//0003-066x.49.8.709. [ DOI ] [ PubMed ] [ Google Scholar ]
- Esser H, Kroneberg C. An Integrative Theory of Action: The Model of Frame Selection. In: Lawler E, Thye S, Yoon J, editors. Order at the Edge of Chaos: Social Psychology and the Problem of Social Order. Cambridge: Cambridge University Press; 2015. pp. 63–85. [ Google Scholar ]
- Evans J. Dual-processing accounts of reasoning, judgment, and social cognition. Annual Review of Psychology. 2008;59:255–78. doi: 10.1146/annurev.psych.59.103006.093629. [ DOI ] [ PubMed ] [ Google Scholar ]
- Feinberg F, Huber J. A theory of cutoff formation under imperfect information. Management Science. 1996;42:65–84. [ Google Scholar ]
- Fischhoff B, Slovic P, Lichtenstein S, Read S, Combs B. How safe is safe enough? A psychometric study of attitudes towards technological risks and benefits. Policy Sciences. 1978;9:127–152. [ Google Scholar ]
- Gely R, Spiller P. A Rational Choice Theory of Supreme Court Statutory Decisions with Applications to the State Farm and Grove City Cases. Journal of Law, Economics, & Organization. 1990;6:263–300. [ Google Scholar ]
- Gennetian L, Eldar S. The Persistence of Poverty in the Context of Financial Instability: A Behavioral Perspective. Journal of Policy Analysis and Management. 2015;34:904–936. [ Google Scholar ]
- Gigerenzer G, Gaissmaier W. Heuristic decision making. Annual Review of Psychology. 2011;62:451–82. doi: 10.1146/annurev-psych-120709-145346. [ DOI ] [ PubMed ] [ Google Scholar ]
- Gigerenzer G, Brighton H. Homo heuristicus: Why biased minds make better inferences. Topics in Cognitive Science. 2009;1:107–143. doi: 10.1111/j.1756-8765.2008.01006.x. [ DOI ] [ PubMed ] [ Google Scholar ]
- Gigerenzer G, Goldstein D. Betting on one good reason: The take the best heuristic. In: Gigerenzer G, Todd P, editors. Simple Heuristics that Make Us Smart. Oxford: Oxford University Press; 1999. pp. 75–95. [ Google Scholar ]
- Gigerenzer G, Todd P. Simple heuristics that make us smart. Oxford University Press; USA: 1999. [ DOI ] [ PubMed ] [ Google Scholar ]
- Gigerenzer G. Fast and frugal heuristics: The tools of bounded rationality. Blackwell handbook of judgment and decision making. 2004:62–88. [ Google Scholar ]
- Gigerenzer G. Why heuristics work. Perspectives on Psychological Science. 2008;3:20–29. doi: 10.1111/j.1745-6916.2008.00058.x. [ DOI ] [ PubMed ] [ Google Scholar ]
- Gilbride T, Allenby G. A choice model with conjunctive, disjunctive, and compensatory screening rules. Marketing Science. 2004;23:391–406. [ Google Scholar ]
- Goffman E. Frame analysis: An essay on the organization of experience. Cambridge: Harvard University Press; 1974. [ Google Scholar ]
- Goldstein D, Johnson E, Herrmann A, Heitmann M. Nudge your Customers towards Better Choices. Harvard Business Review. 2008:100–105. [ Google Scholar ]
- Goldstein N, Cialdini R, Griskevicius V. A room with a viewpoint: Using social norms to motivate environmental conservation in hotels. Journal of Consumer Research. 2008;35:472–82. [ Google Scholar ]
- Goldthorpe J. The quantitative analysis of large-scale data-sets and rational action theory: for a sociological alliance. European Sociological Review. 1996;12:109–26. [ Google Scholar ]
- Granovetter M. Economic action and social structure: The problem of embeddedness. American Journal of Sociology. 1985;91:481–510. [ Google Scholar ]
- Green P, Srinivasan V. Conjoint analysis in consumer research: issues and outlook. Journal of consumer research. 1978;5:103–123. [ Google Scholar ]
- Green P, Krieger A, Wind Y. Thirty years of conjoint analysis: Reflections and prospects. Interfaces. 2001;31:S56–S73. [ Google Scholar ]
- Grether D, Plott C. Economic theory of choice and the preference reversal phenomenon. American Economic Review. 1979;69:623–638. [ Google Scholar ]
- Gross N. A pragmatist theory of social mechanisms. American Sociological Review. 2009;74:358–79. [ Google Scholar ]
- Guadagni P, Little J. A logit model of brand choice calibrated on scanner data. Marketing Science. 1983;2:203–238. [ Google Scholar ]
- Hauser J, Wernerfelt B. An evaluation cost model of consideration sets. Journal of Consumer Research. 1990;16:393–408. [ Google Scholar ]
- Hechter M, Kanazawa S. Sociological rational choice theory. Annual Review of Sociology. 1997;23:191–214. [ Google Scholar ]
- Hedström P, Swedberg R. Rational Choice, Empirical Research, and the Sociological tradition. European Sociological Review. 1996;12:127–146. [ Google Scholar ]
- Hedstrom P. Dissecting the Social: On the Principles of Analytical Sociology. Cambridge: Cambridge University Press; 2005. [ Google Scholar ]
- Hedström P, Bearman P. The Oxford handbook of analytical sociology. Oxford: Oxford University Press; 2009. [ Google Scholar ]
- Henrich J, Heine S, Norenzayan A. The weirdest people in the world? Behavioral and Brain Sciences. 2010;33:61–83. doi: 10.1017/S0140525X0999152X. [ DOI ] [ PubMed ] [ Google Scholar ]
- Hensher D, Rose J, Greene W. Applied Choice Analysis: A Primer. Cambridge: Cambridge University Press; 2005. [ Google Scholar ]
- Higgins E. Knowledge activation: Accessibility, applicability, and salience. In: Higgins E, Kruglanski A, editors. Social Psychology: Handbook of Basic Principles. New York: Guilford Press; 1996. pp. 133–68. [ Google Scholar ]
- Hogarth R. Judgment and choice: The psychology of decision. Chichester: Wiley; 1991. [ Google Scholar ]
- Hochschild A. The Second Shift: Working Families and the Revolution at Home. New York: Penguin Books; [ Google Scholar ]
- Horowitz J, Louviere J. What is the role of consideration sets in choice modeling? International Journal of Research in Marketing. 1995;12:39–54. [ Google Scholar ]
- Howard J, Jagdish N. The theory of buyer behavior. New York: Wiley; 1969. [ Google Scholar ]
- Huber J, Payne J, Puto C. Adding asymmetrically dominated alternatives: Violations of regularity and the similarity hypothesis. Journal of Consumer Research. 1982;9:90–98. [ Google Scholar ]
- Johnson E, Goldstein D. Do defaults save lives? Science. 2003;302:1338–1339. doi: 10.1126/science.1091721. [ DOI ] [ PubMed ] [ Google Scholar ]
- Johnson E, Goldstein D. Decisions by Default. In: Shafir E, editor. The Behavioral Foundations of Public Policy. Princeton: Princeton University Press; 2013. pp. 417–427. [ Google Scholar ]
- Johnson E, Payne J. Effort and accuracy in choice. Management science. 1985;31(4):395–414. [ Google Scholar ]
- Johnson E, Hershey J, Meszaros J, Kunreuther H. Making Decisions About Liability And Insurance. Springer; Netherlands: 1993. Framing, probability distortions, and insurance decisions; pp. 35–51. [ Google Scholar ]
- Jones D. A WEIRD view of human nature skews psychologists’ studies. Science. 2010;328:1627–1627. doi: 10.1126/science.328.5986.1627. [ DOI ] [ PubMed ] [ Google Scholar ]
- Kahneman D, Tversky A. Prospect theory: An analysis of decision under risk. Econometrica. 1979:263–291. [ Google Scholar ]
- Kahneman D, Tversky A. Variants of uncertainty. Cognition. 1982;11:143–57. doi: 10.1016/0010-0277(82)90023-3. [ DOI ] [ PubMed ] [ Google Scholar ]
- Kahneman D, Tversky A. Choices, Values, and Frames. American Psychologist. 1984;39:341–350. [ Google Scholar ]
- Kahneman D. Maps of bounded rationality: Psychology for behavioral economics. American economic review. 2003;93:1449–75. [ Google Scholar ]
- Kahneman D. Thinking, fast and slow. Macmillan; 2011. [ Google Scholar ]
- Kahneman D, Knetsch J, Thaler R. Anomalies: The endowment effect, loss aversion, and status quo bias. The Journal of Economic Perspectives. 1991;5:193–206. [ Google Scholar ]
- Kroneberg C. Frames, Scripts, and Variable rationality: An integrative Theory of Action. In: Manzo G, editor. Analytical Sociology. Chichester, UK: John Wiley and Sons; 2014. pp. 95–123. [ Google Scholar ]
- Kroneberg C, Kalter F. Rational choice theory and empirical research: Methodological and theoretical contributions in Europe. Annual Review of Sociology. 2012;38:73–92. [ Google Scholar ]
- Krysan M, Bader M. Racial blind spots: Black-white-Latino differences in community knowledge. Social Problems. 2009;56:677–701. [ Google Scholar ]
- Lee V, Bryk A, Smith J. The organization of effective secondary schools. Review of Research in Education. 1993;19:171–267. [ Google Scholar ]
- Leschziner V, Green A. Thinking about Food and Sex: Deliberate Cognition in the Routine Practices of a Field. Sociological Theory. 2013;31:116–144. [ Google Scholar ]
- Lerner J, Li Y, Valdesolo P, Kassam K. Emotion and decision making. Annual Review of Psychology. 2015;66:799–823. doi: 10.1146/annurev-psych-010213-115043. [ DOI ] [ PubMed ] [ Google Scholar ]
- Levy J. Prospect theory, rational choice, and international relations. International Studies Quarterly. 1997;41:87–112. [ Google Scholar ]
- Liberman V, Samuels S, Ross L. The name of the game: Predictive power of reputations versus situational labels in determining prisoner’s dilemma game moves. Personality and social psychology bulletin. 2004;30:1175–1185. doi: 10.1177/0146167204264004. [ DOI ] [ PubMed ] [ Google Scholar ]
- Lichtenstein S, Slovic P. The construction of preference. Cambridge: Cambridge University Press; 2006. [ Google Scholar ]
- Lindenberg S, Frey B. Alternatives, frames, and relative prices: A broader view of rational choice theory. Acta Sociologica. 1993;36:191–205. [ Google Scholar ]
- Liu L, Dukes A. Consideration set formation with multiproduct firms: The case of within-firm and across-firm evaluation costs. Management Science. 2013;59:1871–1886. [ Google Scholar ]
- Lizardo O. Beyond the Comtean Schema: The Sociology of Culture and Cognition Versus Cognitive Social Science. Sociological Forum. 2014;29:983–989. [ Google Scholar ]
- Loewenstein G, Lerner J. The role of affect in decision making. Handbook of affective science. 2003;619:3. [ Google Scholar ]
- Loewenstein G. The creative destruction of decision research. Journal of Consumer Research. 2001;28:499–505. [ Google Scholar ]
- Loewenstein G, Weber E, Hsee C, Welch N. Risk as feelings. Psychological bulletin. 2001;127:267. doi: 10.1037/0033-2909.127.2.267. [ DOI ] [ PubMed ] [ Google Scholar ]
- Lohse G, Johnson E. A comparison of two process tracing methods for choice tasks. Organizational Behavior and Human Decision Processes. 1996;68(1):28–43. [ Google Scholar ]
- Louviere J, Hensher D, Swait J. Stated choice methods: analysis and applications. Cambridge: Cambridge University Press; 2000. [ Google Scholar ]
- Louviere J, Meyer R, Bunch D, Carson R, Dellaert B, Hanemann W, Hensher D, Irwin J. Combining sources of preference data for modeling complex decision processes. Marketing Letters. 1999;10:205–217. [ Google Scholar ]
- Luce R. Individual Choice Behavior: A Theoretical Analysis. New York: Wiley; 1959. [ Google Scholar ]
- Luce M, Bettman J, Payne J. Emotional decisions: Tradeoff difficulty and coping in consumer choice. Monographs of the Journal of Consumer Research. 2001;1:1–209. [ Google Scholar ]
- Luce M, Payne J, Bettman J. Emotional trade-off difficulty and choice. Journal of Marketing Research. 1999:143–159. [ Google Scholar ]
- Luce M, Payne J, Bettman J. Coping with unfavorable attribute values in choice. Organizational behavior and human decision processes. 81:274–99. doi: 10.1006/obhd.1999.2872. [ DOI ] [ PubMed ] [ Google Scholar ]
- Mahoney J. The logic of process tracing tests in the social sciences. Sociological Methods & Research. 2012;41:570–597. [ Google Scholar ]
- Mandel N, Johnson E. When web pages influence choice: Effects of visual primes on experts and novices. Journal of consumer research. 2002;29:235–245. [ Google Scholar ]
- Manski C. Economic Analysis of Social Interactions. The Journal of Economic Perspectives. 14:115–136. [ Google Scholar ]
- Manzo G. Is rational choice theory still a rational choice of theory? A response to Opp. Social Science Information. 2013;52:361–382. [ Google Scholar ]
- Margolis H. Selfishness and Altruism. Chicago: University of Chicago Press; 1982. [ Google Scholar ]
- Matza D. Delinquency and Drift. Transaction Publishers; 1967. [ Google Scholar ]
- McDaniels T, Axelrod L, Cavanagh N, Slovic P. Perception of ecological risk to water environments. Risk analysis. 1997;17:341–352. doi: 10.1111/j.1539-6924.1997.tb00872.x. [ DOI ] [ PubMed ] [ Google Scholar ]
- McFadden D. Conditional Logit Analysis of Qualitative Choice Behavior. In: Zarembka P, editor. Frontiers in Econometrics. New York: Academic Press; 1973. pp. 105–142. [ Google Scholar ]
- McFadden D. Rationality for economists? Journal of Risk and Uncertainty. 1999;19:73–105. [ Google Scholar ]
- McFadden D. Economic Choices. The American Economic Review. 2001;91:351–378. [ Google Scholar ]
- Miller G. The magical number seven, plus or minus two: Some limits on our capacity for processing information. Psychological review. 1956;63:81. [ PubMed ] [ Google Scholar ]
- Miller N. Liberalization of Basic SR Concepts: Extensions to Conflict Behavior, Motivation, and Social Learning. McGraw-Hill Company; 1959. [ Google Scholar ]
- Munch R. Rational Choice Theory: A Critical Assessment of Its Explanatory Power. In: Coleman J, Fararo T, editors. Rational Choice Theory: Advocacy and Critique. London: Sage Publications; 1992. pp. 137–161. [ Google Scholar ]
- Mullainathan S, Shafir E. Scarcity: Why having too little means so much. New York: Times Books; 2013. [ Google Scholar ]
- Newell A, Simon H. Human Problem Solving. Englewood Cliffs, NJ: Prentice-Hall; 1972. [ Google Scholar ]
- Nolan J, Schultz P, Cialdini R, Goldstein N, Griskevicius V. Normative social influence is underdetected. Personality and social psychology bulletin. 2008;34:913–923. doi: 10.1177/0146167208316691. [ DOI ] [ PubMed ] [ Google Scholar ]
- Pachucki M, Jacques P, Christakis N. Social network concordance in food choice among spouses, friends, and siblings. American Journal of Public Health. 2011;101:2170–2177. doi: 10.2105/AJPH.2011.300282. [ DOI ] [ PMC free article ] [ PubMed ] [ Google Scholar ]
- Payne J, Bettman J. Walking with the scarecrow: The information-processing approach to decision research. In: Koehler D, Harvey N, editors. Blackwell handbook of judgment and decision making. Malden, MA: Blackwell; 2004. pp. 110–132. [ Google Scholar ]
- Payne J. Task complexity and contingent processing in decision making: An information search and protocol analysis. Organizational behavior and human performance. 1976;16:366–387. [ Google Scholar ]
- Payne J, Bettman J, Johnson E. The adaptive decision maker. Cambridge: Cambridge University Press; 1993. [ Google Scholar ]
- Perna L, Titus M. The relationship between parental involvement as social capital and college enrollment: An examination of racial/ethnic group differences. Journal of Higher Education. 2005;76:485–518. [ Google Scholar ]
- Pescosolido B. Beyond rational choice: The social dynamics of how people seek help. American Journal of Sociology. 1992;97:1096–1138. [ Google Scholar ]
- Pocheptsova A, Amir O, Dhar R, Baumeister R. Deciding without resources: Resource depletion and choice in context. Journal of Marketing Research. 2009;46:344–55. [ Google Scholar ]
- Rieskamp J, Hoffrage U. Inferences under time pressure: How opportunity costs affect strategy selection. Acta psychological. 2008;127:258–76. doi: 10.1016/j.actpsy.2007.05.004. [ DOI ] [ PubMed ] [ Google Scholar ]
- Rieskamp J, Otto P. SSL: a theory of how people learn to select strategies. Journal of Experimental Psychology: General. 2006;135:207. doi: 10.1037/0096-3445.135.2.207. [ DOI ] [ PubMed ] [ Google Scholar ]
- Roberts J, Lattin J. Development and testing of a model of consideration set composition. Journal of Marketing Research. 1991:429–440. [ Google Scholar ]
- Roberts J, Lattin J. Consideration: Review of research and prospects for future insights. Journal of Marketing Research. 1997:406–410. [ Google Scholar ]
- Rosenquist N, Murabito J, Fowler J, Christakis N. The spread of alcohol consumption behavior in a large social network. Annals of Internal Medicine. 2010;152:426–433. doi: 10.1059/0003-4819-152-7-201004060-00007. [ DOI ] [ PMC free article ] [ PubMed ] [ Google Scholar ]
- Nisbett R, Ross L. The person and the situation. New York: McGraw Hill; 1991. [ Google Scholar ]
- Rossi P, Allenby G, McCulloch R. Bayesian Statistics and Marketing. Wiley, Chichester; 2005. [ Google Scholar ]
- Salisbury L, Feinberg F. All things considered? The role of choice set formation in diversification. Journal of Marketing Research. 2012;49(3):320–335. [ Google Scholar ]
- Sampson R, Morenoff J, Gannon-Rowley T. Assessing Neighborhood Effects: Social Processes and New Directions in Research. Annual Review of Sociology. 2002;28:443–478. [ Google Scholar ]
- Samuelson P. Some Implications of Linearity. The Review of Economic Studies. 1947;15:88–90. [ Google Scholar ]
- Samuelson P. Consumption theory in terms of revealed preference. Economica. 1948;15:243–253. [ Google Scholar ]
- Samuelson W, Zeckhauser R. Status quo bias in decision making. Journal of risk and uncertainty. 1988;1:7–59. [ Google Scholar ]
- Satz D, Ferejohn J. Rational choice and social theory. The Journal of Philosophy. 1994;91:71–87. [ Google Scholar ]
- Scheff T. Shame and conformity: The deference-emotion system. American Sociological Review. 1988;53:395–406. [ Google Scholar ]
- Schelling T. Dynamic models of segregation. Journal of Mathematical Sociology. 1971;1:143–186. [ Google Scholar ]
- Schneider S. Framing and conflict: aspiration level contingency, the status quo, and current theories of risky choice. Journal of Experimental Psychology: Learning, Memory, and Cognition. 1992;18:1040. doi: 10.1037//0278-7393.18.5.1040. [ DOI ] [ PubMed ] [ Google Scholar ]
- Schulte-Mecklenbeck M, Kuehberger A, Ranyard R. A Handbook of Process Tracing Methods for Decision Research: A Critical Review and User’s Guide (The Society for Judgment and Decision Making Series) Psychology Press; 2010. [ Google Scholar ]
- Schultz P, Wesley J, Nolan M, Cialdini R, Goldstein N, Griskevicius V. The Constructive, Destructive, and Reconstructive Power of Social Norms. Psychological science. 2007;18:429–434. doi: 10.1111/j.1467-9280.2007.01917.x. [ DOI ] [ PubMed ] [ Google Scholar ]
- Schwarz N. Self-reports: how the questions shape the answers. American Psychologist. 1999;54:93–105. [ Google Scholar ]
- Schwarz N. Attitude construction: Evaluation in context. Social Cognition. 2007;25:638–56. [ Google Scholar ]
- Schwarz N, Strack F, Mai H. Assimilation and contrast effects in part-whole question sequences: A conversational logic analysis. Public Opinion Quarterly. 1991;55:3–23. [ Google Scholar ]
- Seidl C. Preference reversal. Journal of Economic Surveys. 2002;16:621–55. [ Google Scholar ]
- Shafir E. The Behavioral Foundations of Public Policy. Princeton: Princeton University Press; 2013. [ Google Scholar ]
- Shah A, Shafir E, Mullainathan S. Scarcity frames value. Psychological Science. 2015;26:402–412. doi: 10.1177/0956797614563958. [ DOI ] [ PubMed ] [ Google Scholar ]
- Shang J, Croson R. A field experiment in charitable contribution: The impact of social information on the voluntary provision of public goods. The Economic Journal. 2009;119:1422–39. [ Google Scholar ]
- Sharkey P, Faber J. Where, when, why, and for whom do residential contexts matter? Moving away from the dichotomous understanding of neighborhood effects. Annual Review of Sociology. 2014;40:559–579. [ Google Scholar ]
- Shugan S. The Cost of Thinking. Journal of consumer Research. 1980;7:99–111. [ Google Scholar ]
- Simon H. Rational choice and the structure of the environment. Psychological Review. 1956;63:129. doi: 10.1037/h0042769. [ DOI ] [ PubMed ] [ Google Scholar ]
- Simon H. Models of bounded rationality: Empirically grounded economic reason. MIT press; [ Google Scholar ]
- Simon H. Invariants of human behavior. Annual review of psychology. 1991;41:1–20. doi: 10.1146/annurev.ps.41.020190.000245. [ DOI ] [ PubMed ] [ Google Scholar ]
- Simonson I. Choice based on reasons: The case of attraction and compromise effects. Journal of Consumer Research. 1989;16:158–174. [ Google Scholar ]
- Simonson I, Tversky A. Choice in context: Tradeoff contrast and extremeness aversion. Journal of Marketing Research. 1992;29:281–95. [ Google Scholar ]
- Slovic P. Perception of Risk. Science. 1987;236:280–285. doi: 10.1126/science.3563507. [ DOI ] [ PubMed ] [ Google Scholar ]
- Slovic P. The Construction of Preference. American Psychologist. 1995;50:364. [ Google Scholar ]
- Slovic P. The Perception of Risk. Earthscan Publications; 2000. [ Google Scholar ]
- Slovic P, Monahan J, MacGregor D. Violence risk assessment and risk communication: the effects of using actual cases, providing instruction, and employing probability versus frequency formats. Law and human behavior. 2000;24:271. doi: 10.1023/a:1005595519944. [ DOI ] [ PubMed ] [ Google Scholar ]
- Slovic P, Peters E. Risk perception and Affect. Current Directions in Psychological Science. 2006;15:322–325. [ Google Scholar ]
- Slovic P, Finucane M, Peters E, MacGregor D. Risk as analysis and risk as feelings: Some thoughts about affect, reason, risk, and rationality. Risk analysis. 2004;24:311–322. doi: 10.1111/j.0272-4332.2004.00433.x. [ DOI ] [ PubMed ] [ Google Scholar ]
- Small M. Unanticipated gains: Origins of Network Inequality in Everyday Life. Oxford: Oxford University Press; 2009. [ Google Scholar ]
- Small M, Sukhu C. Because they were there: Access, deliberation, and the mobilization of networks for support. Social Networks. 2016;47:73–84. [ Google Scholar ]
- Somers M. “We’re No Angels”: Realism, Rational Choice, and Relationality in Social Science. American Journal of Sociology. 1998;104:722–784. [ Google Scholar ]
- Stets J, Turner J. Handbook of the Sociology of Emotions: Volume II. New York: Springer; 2014. [ Google Scholar ]
- Stigler G. The development of utility theory. I and II. The Journal of Political Economy. 1950:307–327. [ Google Scholar ]
- Strough J, Karns T, Schlosnagle L. Decision-making heuristics and biases across the life span. Annals of the New York Academy of Sciences. 2011;1235:57–74. doi: 10.1111/j.1749-6632.2011.06208.x. [ DOI ] [ PMC free article ] [ PubMed ] [ Google Scholar ]
- Svenson O. Process descriptions of decision making. Organizational behavior and human performance. 1979;23(1):86–112. [ Google Scholar ]
- Swait J. A non-compensatory choice model incorporating attribute cutoffs. Transportation Research Part B: Methodological. 2001;35(10):903–928. [ Google Scholar ]
- Swait J. Doctoral dissertation. Massachusetts Institute of Technology; 1984. Probabilistic choice set generation in transportation demand models. [ Google Scholar ]
- Thaler R, Sunstein C. Nudge: Improving Decisions about Health, Wealth, and Happiness. New York: Penguin Books; 2009. [ Google Scholar ]
- Thomas W, Znaniecki F. The Polish peasant in Europe and America: Monograph of an immigrant group. Chicago: University of Chicago Press; 1918. [ Google Scholar ]
- Train K. Discrete Choice Methods with Simulation. 2nd. Cambridge: Cambridge University Press; 2009. [ Google Scholar ]
- Tversky A, Kahneman D. Availability: A heuristic for judging frequency and probability. Cognitive Psychology. 1973;5:207–32. [ Google Scholar ]
- Tversky A, Kahneman D. The Framing of decisions and the psychology of choice. Science. 1981;211(4481):453–458. doi: 10.1126/science.7455683. [ DOI ] [ PubMed ] [ Google Scholar ]
- Tversky A, Kahneman D. Rational choice and the Framing of Decisions. Journal of Business. 1986:S251–S278. [ Google Scholar ]
- Tversky A, Sattath S, Slovic P. Contingent Weighting in Judgment and Choice. Psychological Review. 1988;95:371–384. [ Google Scholar ]
- Tversky A, Simonson I. Context-dependent preferences. Management Science. 1993;39:1179–1189. [ Google Scholar ]
- Uzzi B. Social Structure and Competition in Interfirm Networks: The Paradox of Embeddedness. Administrative Science Quarterly. 1997;1:35–67. [ Google Scholar ]
- Vaisey S. Motivation and Justification: a Dual-Process Model of Culture in Action. American Journal of Sociology. 2009;114:1675–1715. doi: 10.1086/597179. [ DOI ] [ PubMed ] [ Google Scholar ]
- Vaughan D. Rational choice, situated action, and the social control of organizations. Law and Society Review. 1998:23–61. [ Google Scholar ]
- Von Neumann J, Morgenstern O. Theory of games and economic behavior. Princeton university press; 2007. [ Google Scholar ]
- Ward A, Ross L, Reed E, Turiel E, Brown T. Naive realism in everyday life: Implications for social conflict and misunderstanding. Values and knowledge. 1997:103–135. [ Google Scholar ]
- Weber E, Johnson E. Mindful judgment and decision making. Annual review of psychology. 2009;60:53. doi: 10.1146/annurev.psych.60.110707.163633. [ DOI ] [ PubMed ] [ Google Scholar ]
- Wedel M, Pieters R. A review of eye-tracking research in marketing. Review of marketing research. 2008;4:123–147. [ Google Scholar ]
- Windschitl P, Weber E. The interpretation of likely depends on the context, but 70% is 70%—right? The influence of associative processes on perceived certainty. Journal of Experimental Psychology: Learning, Memory, and Cognition. 1999;25:1514. doi: 10.1037//0278-7393.25.6.1514. [ DOI ] [ PubMed ] [ Google Scholar ]
- Wittink D, Cattin P. Commercial use of conjoint analysis: An update. The Journal of Marketing. 1989:91–96. [ Google Scholar ]
- Wittink D, Vriens M, Burhenne W. Commercial use of conjoint analysis in Europe: Results and critical reflections. International journal of Research in Marketing. 1994;11:41–52. [ Google Scholar ]
- Yoon C, Gutchess A, Feinberg F, Polk T. A functional magnetic resonance imaging study of neural dissociations between brand and person judgments. Journal of Consumer Research. 2006;33(1):31–40. [ Google Scholar ]
- Wright P, Weitz B. Time Horizon Effects on Product Evaluation Strategies. Journal of Marketing Research. 1977;14:429–443. [ Google Scholar ]
- Zajonc R. Feeling and thinking: Preferences need no inferences. American psychologist. 1980;35:151. [ Google Scholar ]
- View on publisher site
- PDF (122.8 KB)
- Collections
Similar articles
Cited by other articles, links to ncbi databases.
- Download .nbib .nbib
- Format: AMA APA MLA NLM
Add to Collections
Decision Making: a Theoretical Review
- Regular Article
- Published: 15 November 2021
- Volume 56 , pages 609–629, ( 2022 )
Cite this article
- Matteo Morelli 1 ,
- Maria Casagrande ORCID: orcid.org/0000-0002-4430-3367 2 &
- Giuseppe Forte 1 , 3
9289 Accesses
21 Citations
1 Altmetric
Explore all metrics
Decision-making is a crucial skill that has a central role in everyday life and is necessary for adaptation to the environment and autonomy. It is the ability to choose between two or more options, and it has been studied through several theoretical approaches and by different disciplines. In this overview article, we contend a theoretical review regarding most theorizing and research on decision-making. Specifically, we focused on different levels of analyses, including different theoretical approaches and neuropsychological aspects. Moreover, common methodological measures adopted to study decision-making were reported. This theoretical review emphasizes multiple levels of analysis and aims to summarize evidence regarding this fundamental human process. Although several aspects of the field are reported, more features of decision-making process remain uncertain and need to be clarified. Further experimental studies are necessary for understanding this process better and for integrating and refining the existing theories.
This is a preview of subscription content, log in via an institution to check access.
Access this article
Subscribe and save.
- Get 10 units per month
- Download Article/Chapter or eBook
- 1 Unit = 1 Article or 1 Chapter
- Cancel anytime
Price excludes VAT (USA) Tax calculation will be finalised during checkout.
Instant access to the full article PDF.
Rent this article via DeepDyve
Institutional subscriptions
Similar content being viewed by others
Theoretical Perspectives on Decision Making
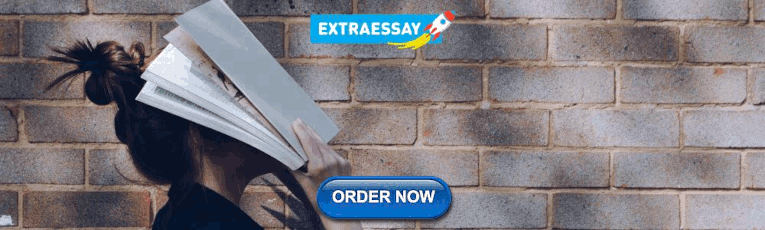
Decision-Making
Psychological Determinants of Decision Making
André, M., Borgquist, L., Foldevi, M., & Mölstad, S. (2002). Asking for ‘rules of thumb’: a way to discover tacit knowledge in general practice. Family Practice, 19 (6), 617–22. https://doi.org/10.1093/fampra/19.6.617
Article PubMed Google Scholar
Bechara, A., Damasio, A. R., Damasio, H., & Anderson, S. W. (1994). Insensitivity to future consequences following damage to human prefrontal cortex. Cognition, 50 (1–3), 7–15. https://doi.org/10.1016/0010-0277(94)90018-3
Bechara, A., Damasio, H., Tranel, D., & Damasio, A. R. (1997). Deciding advantageously before knowing the advantageous strategy. Science, 275 (5304), 1293–5. https://doi.org/10.1126/science.275.5304.1293
Bechara, A., Damasio, H., & Damasio, A. R. (2000a). Emotion, decision making and the orbitofrontal cortex. Cerebral cortex, 10 (3), 295–307.
Bechara, A., Tranel, D., & Damasio, H. (2000b). Characterization of the decision-making deficit of patients with ventromedial prefrontal cortex lesions. Brain, 123 (Pt 11), 2189–202. https://doi.org/10.1093/brain/123.11.2189
Bechara, A., & Damasio, A. R. (2005). The somatic marker hypothesis: a neural theory of economic decision. Games and Economic Behavior, 52, 336–372. https://doi.org/10.1016/j.geb.2004.06.010
Article Google Scholar
Blanchard, T. C., Strait, C. E., & Hayden, B. Y. (2015). Ramping ensemble activity in dorsal anterior cingulate neurons during persistent commitment to a decision. Journal of Neurophysiology, 114 (4), 2439–49. https://doi.org/10.1152/jn.00711.2015
Article PubMed PubMed Central Google Scholar
Bohanec, M. (2009). Decision making: A computer-science and information-technology viewpoint. Interdisciplinary Description of Complex Systems, 7 (2), 22–37
Google Scholar
Brand, M., Fujiwara, E., Borsutzky, S., Kalbe, E., Kessler, J., & Markowitsch, H. J. (2005). Decision-Making deficits of korsakoff patients in a new gambling task with explicit rules: associations with executive functions. Neuropsychology, 19 (3), 267–277. https://doi.org/10.1037/0894-4105.19.3.267
Broche-Pérez, Y., Jiménez, H., & Omar-Martínez, E. (2016). Neural substrates of decision-making. Neurologia, 31 (5), 319–25. https://doi.org/10.1016/j.nrl.2015.03.001
Byrnes, J. P. (2013). The nature and development of decision-making: A self-regulation model . Psychology Press
Clark, L., & Manes, F. (2004). Social and emotional decision-making following frontal lobe injury. Neurocase, 10 (5), 398–403. https://doi.org/10.1080/13554790490882799
Cummings, J. L. (1995). Anatomic and behavioral aspects of frontal-subcortical circuits a. Annals of the New York Academy of Sciences, 769 (1), 1–14
Dale, S. (2015). Heuristics and biases: The science of decision-making. Business Information Review, 32 (2), 93–99. https://doi.org/10.1177/0266382115592536
Damasio, A. R. (1996). The somatic marker hypothesis and the possible functions of the prefrontal cortex. Philosophical Transactions of the Royal Society of London. Series B, Biological Sciences, 351 (1346), 1413–20. https://doi.org/10.1098/rstb.1996.0125
Dewberry, C., Juanchich, M., & Narendran, S. (2013). Decision-making competence in everyday life: The roles of general cognitive styles, decision-making styles and personality. Personality and Individual Differences, 55 (7), 783–788. https://doi.org/10.1016/j.paid.2013.06.012
Doya, K. (2008). Modulators of decision making. Nature Neuroscience, 11 (4), 410–6. https://doi.org/10.1038/nn2077
Dunn, B. D., Dalgleish, T., & Lawrence, A. D. (2006). The somatic marker hypothesis: a critical evaluation. Neuroscience & Biobehavioral Reviews, 30 (2), 239–71. https://doi.org/10.1016/j.neubiorev.2005.07.001
Elliott, R., Rees, G., & Dolan, R. J. (1999). Ventromedial prefrontal cortex mediates guessing. Neuropsychologia, 37 (4), 403–411
Ernst, M., Bolla, K., Mouratidis, M., Contoreggi, C., Matochik, J. A., Kurian, V., et al. (2002). Decision-making in a risk-taking task: a PET study. Neuropsychopharmacology, 26 (5), 682–91. https://doi.org/10.1016/S0893-133X(01)00414-6
Ernst, M., & Paulus, M. P. (2005). Neurobiology of decision making: a selective review from a neurocognitive and clinical perspective. Biological Psychiatry, 58 (8), 597–604. https://doi.org/10.1016/j.biopsych.2005.06.004
Evans, J. S. (2008). Dual-processing accounts of reasoning, judgment, and social cognition. Annual Review of Psychology, 59, 255–78. https://doi.org/10.1146/annurev.psych.59.103006.093629
Fellows, L. K. (2004). The cognitive neuroscience of human decision making: A review and conceptual framework. Behavioral & Cognitive Neuroscience Reviews, 3 (3), 159–72. https://doi.org/10.1177/1534582304273251
Fellows, L. K., & Farah, M. J. (2007). The role of ventromedial prefrontal cortex in decision making: judgment under uncertainty or judgment per se? Cerebral Cortex, 17 (11), 2669–74. https://doi.org/10.1093/cercor/bhl176
Fehr, E., & Camerer, C. F. (2007). Social neuroeconomics: the neural circuitry of social preferences. Trends in Cognitive Sciences, 11 (10), 419–27. https://doi.org/10.1016/j.tics.2007.09.002
Finucane, M. L., Alhakami, A., Slovic, P., & Johnson, S. M. (2000). The affect heuristic in judgments of risks and benefits. Journal of Behavioral Decision Making, 13, 1–17. https://doi.org/10.1002/(SICI)1099-0771(200001/03)13:1<1::AID-BDM333>3.0.CO;2-S
Fischhoff, B. (2010). Judgment and decision making. Wiley Interdisciplinary Reviews: Cognitive Science, 1 (5), 724–735. https://doi.org/10.1002/wcs.65
Forte, G., Favieri, F., & Casagrande, M. (2019). Heart rate variability and cognitive function: a systematic review. Frontiers in Neuroscience, 13, 710
Forte, G., Morelli, M., & Casagrande, M. (2021). Heart rate variability and decision-making: autonomic responses in making decisions. Brain Sciences, 11 (2), 243
Forte, G., Favieri, F., Oliha, E. O., Marotta, A., & Casagrande, M. (2021). Anxiety and attentional processes: the role of resting heart rate variability. Brain Sciences, 11 (4), 480
Frith, C. D., & Singer, T. (2008). The role of social cognition in decision making. Philosophical Transactions of the Royal Society of London. Series B, Biological Sciences, 363 (1511), 3875–86. https://doi.org/10.1098/rstb.2008.0156
Galotti, K. M. (2002). Making decisions that matter: How people face important life choices . Lawrence Erlbaum Associates Publishers
Gigerenzer, G., & Gaissmaier, W. (2011). Heuristic decision making. Annual Review of Psychology, 62, 451–82. https://doi.org/10.1146/annurev-psych-120709-145346
Gigerenzer, G., & Selten, R. (Eds.). (2001). Bounded Rationality: The Adaptive Toolbox . MIT Press
Goel, V., Gold, B., Kapur, S., & Houle, S. (1998). Neuroanatomical correlates of human reasoning. Journal of Cognitive Neuroscience, 10 (3), 293–302
Gold, J. I., & Shadlen, M. N. (2007). The neural basis of decision making. Annual Review of Neuroscience, 30, 535–74. https://doi.org/10.1146/annurev.neuro.29.051605.113038
Gottlieb, J. (2007). From thought to action: the parietal cortex as a bridge between perception, action, and cognition. Neuron, 53 (1), 9–16. https://doi.org/10.1016/j.neuron.2006.12.009
Gozli, D. G. (2017). Behaviour versus performance: The veiled commitment of experimental psychology. Theory & Psychology, 27, 741–758
Gozli, D. (2019). Free Choice. Experimental Psychology and Human Agency . Springer. https://doi.org/10.1007/978-3-030-20422-8_6
Group, T. M. A. D., Fawcett, T. W., Fallenstein, B., Higginson, A. D., Houston, A. I., Mallpress, D. E., & McNamara, J. M., …. (2014). The evolution of decision rules in complex environments. Trends in Cognitive Sciences , 18 (3), 153–161
Guess, C. (2004). Decision making in individualistic and collectivistic cultures. Online Readings in Psychology and Culture , 4 (1). https://doi.org/10.9707/2307-0919.1032
Gupta, R., Koscik, T. R., Bechara, A., & Tranel, D. (2011). The amygdala and decision-making. Neuropsychologia, 49 (4), 760–6. https://doi.org/10.1016/j.neuropsychologia.2010.09.029
Heilbronner, S. R., & Hayden, B. Y. (2016). Dorsal anterior cingulate cortex: a bottom-up view. Annual Review of Neuroscience, 39, 149–70. https://doi.org/10.1146/annurev-neuro-070815-013952
Hickson, L., & Khemka, I. (2014). The psychology of decision making. International review of research in developmental disabilities (Vol 47, pp. 185–229). Academic
Johnson, J. G., & Busemeyer, J. R. (2010). Decision making under risk and uncertainty. Wiley Interdisciplinary Reviews: Cognitive Science, 1 (5), 736–749. https://doi.org/10.1002/wcs.76
Kable, J. W., & Glimcher, P. W. (2009). The neurobiology of decision: consensus and controversy. Neuron , 63 (6),733–45. https://doi.org/10.1016/j.neuron.2009.09.003
Kahneman, D. (2003). A perspective on judgment and choice. Mapping bounded rationality. American Psychologist, 58 (9), 697–720. https://doi.org/10.1037/0003-066X.58.9.697
Kahneman, D. (2011). P ensieri lenti e veloci . Trad.it. a cura di Serra, L., Arnoldo Mondadori Editore
Kahneman, D., & Tversky, A. (1979). Prospect theory: an analysis of decision under risk. Econometrica, 47 (2), 263–292
Kheramin, S., Body, S., Mobini, S., Ho, M. Y., Velázquez-Martinez, D. N., Bradshaw, C. M., et al. (2002). Effects of quinolinic acid-induced lesions of the orbital prefrontal cortex on inter-temporal choice: a quantitative analysis. Psychopharmacology (Berl), 165 (1), 9–17. https://doi.org/10.1007/s00213-002-1228-6
Lee, V. K., & Harris, L. T. (2013). How social cognition can inform social decision making. Frontiers in Neuroscience, 7, 259. https://doi.org/10.3389/fnins.2013.00259
Lerner, J. S., Li, Y., Valdesolo, P., & Kassam, K. S. (2015). Emotion and decision making. Annual Review of Psychology, 66 , 799–823
Loewenstein, G., Weber, E. U., Hsee, C. K., & Welch, N. (2001). Risk as feelings. Psychological Bulletin, 127 (2), 267–286. https://doi.org/10.1037/0033-2909.127.2.267
Mather, M. (2006). A review of decision-making processes: weighing the risks and benefits of aging. In Carstensen, L. L., & Hartel, C. R. (Eds.), & Committee on Aging Frontiers in Social Psychology, Personality, and Adult Developmental Psychology, Board on Behavioral, Cognitive, and Sensory Sciences, When I’m 64 (pp. 145–173). National Academies Press
Mazzucchi, L. (2012). La riabilitazione neuropsicologica: Premesse teoriche e applicazioni cliniche (3rd ed.). EDRA
Mishra, S. (2014). Decision-making under risk: integrating perspectives from biology, economics, and psychology. Personality and Social Psychology Review, 18 (3), 280–307. https://doi.org/10.1177/1088868314530517
Moreira, C. (2018). Unifying decision-making: a review on evolutionary theories on rationality and cognitive biases. arXiv preprint arXiv:1811.12455
Naqvi, N., Shiv, B., & Bechara, A. (2006). The role of emotion in decision making: a cognitive neuroscience perspective. Current Directions in Psychological Science, 15 (5), 260–264. https://doi.org/10.1111/j.1467-8721.2006.00448.x
O’Doherty, J. P., Buchanan, T. W., Seymour, B., & Dolan, R. J. (2006). Predictive neural coding of reward preference involves dissociable responses in human ventral midbrain and ventral striatum. Neuron, 49 (1), 157–66. https://doi.org/10.1016/j.neuron.2005.11.014
Padoa-Schioppa, C., & Assad, J. A. (2008). The representation of economic value in the orbitofrontal cortex is invariant for changes of menu. Nature Neuroscience, 11 (1), 95–102. https://doi.org/10.1038/nn2020
Palombo, D. J., Keane, M. M., & Verfaellie, M. (2015). How does the hippocampus shape decisions? Neurobiology of Learning and Memory, 125, 93–7. https://doi.org/10.1016/j.nlm.2015.08.005
Pardo-Vazquez, J. L., Padron, I., Fernandez-Rey, J., & Acuña, C. (2011). Decision-making in the ventral premotor cortex harbinger of action. Frontiers in Integrative Neuroscience, 5, 54. https://doi.org/10.3389/fnint.2011.00054
Paulus, M. P., & Yu, A. J. (2012). Emotion and decision-making: affect-driven belief systems in anxiety and depression. Trends in Cognitive Science, 16, 476–483. https://doi.org/10.1016/j.tics.2012.07.009
Payne, J. W. (1973). Alternative approaches to decision making under risk: Moments versus risk dimensions. Psychological Bulletin, 80 (6), 439–453. https://doi.org/10.1037/h0035260
Payne, J. W., Payne, J. W., Bettman, J. R., & Johnson, E. J. (1993). The adaptive decision maker . Cambridge University Press
Phelps, E. A., Lempert, K. M., & Sokol-Hessner, P. (2014). Emotion and decision making: multiple modulatory neural circuits. Annual Review of Neuroscience, 37, 263–287
Pronin, E. (2007). Perception and misperception of bias in human judgment. Trends in Cognitive Sciences, 11 (1), 37–43
Rangel, A., Camerer, C., & Read Montague, P. (2008). Neuroeconomics: The neurobiology of value-based decision-making. Nature Reviews Neuroscience, 9 (7), 545–556. https://doi.org/10.1038/nrn2357
Reyna, V. F., & Lloyd, F. J. (2006). Physician decision making and cardiac risk: Effects of knowledge, risk perception, risk tolerance, and fuzzy processing. Journal of Experimental Psychology: Applied, 12 (3), 179–195. https://doi.org/10.1037/1076-898X.12.3.179
Rilling, J. K., & Sanfey, A. G. (2011). The neuroscience of social decision-making. Annual Review of Psychology, 62, 23–48. https://doi.org/10.1146/annurev.psych.121208.131647
Robinson, D. N. (2016). Explanation and the “brain sciences". Theory & Psychology, 26 (3), 324–332
Robbins, T. W., James, M., Owen, A. M., Sahakian, B. J., McInnes, L., & Rabbitt, P. (1994). Cambridge Neuropsychological Test Automated Battery (CANTAB): a factor analytic study of a large sample of normal elderly volunteers. Dementia, 5 (5), 266–81. https://doi.org/10.1159/000106735
Rogers, R. D., Owen, A. M., Middleton, H. C., Williams, E. J., Pickard, J. D., Sahakian, B. J., et al. (1999). Choosing between small, likely rewards and large, unlikely rewards activates inferior and orbital prefrontal cortex. The Journal of Neuroscience, 19 (20), 9029–9038. https://doi.org/10.1523/JNEUROSCI.19-20-09029.1999
Rolls, E. T., & Baylis, L. L. (1994). Gustatory, olfactory, and visual convergence within the primate orbitofrontal cortex. The Journal of Neuroscience, 14 (9), 5437–52. https://doi.org/10.1523/JNEUROSCI.14-09-05437.1994
Rolls, E. T., Critchley, H. D., Browning, A. S., Hernadi, I., & Lenard, L. (1999). Responses to the sensory properties of fat of neurons in the primate orbitofrontal cortex. The Journal of Neuroscience, 19 (4), 1532–40. https://doi.org/10.1523/JNEUROSCI.19-04-01532.1999
Rosenbloom, M. H., Schmahmann, J. D., & Price, B. H. (2012). The functional neuroanatomy of decision-making. The Journal of Neuropsychiatry and Clinical Neurosciences, 24 (3), 266–77. https://doi.org/10.1176/appi.neuropsych.11060139
Rushworth, M. F., & Behrens, T. E. (2008). Choice, uncertainty and value in prefrontal and cingulate cortex. Nature Neuroscience, 11 (4), 389–97. https://doi.org/10.1038/nn2066
Sanfey, A. G. (2007). Social decision-making: insights from game theory and neuroscience. Science, 318 (5850), 598–602. https://doi.org/10.1126/science.1142996
Serra, L., Bruschini, M., Ottaviani, C., Di Domenico, C., Fadda, L., Caltagirone, C., et al. (2019). Thalamocortical disconnection affects the somatic marker and social cognition: a case report. Neurocase, 25 (1–2), 1–9. https://doi.org/10.1080/13554794.2019.1599025
Shahsavarani, A. M., & Abadi, E. A. M. (2015). The bases, principles, and methods of decision-making: A review of literature. International Journal of Medical Reviews, 2 (1), 214–225
Slovic, P., Finucane, M. L., Peters, E., & MacGregor, D. G. (2002). Rational actors or rational fools: Implications of the affect heuristic for behavioral economics. Journal of Socio-Economics, 31 (4), 329–342. https://doi.org/10.1016/S1053-5357(02)00174-9
Slovic, P., Finucane, M. L., Peters, E., & MacGregor, D. G. (2004). Risk as analysis and risk as feelings: some thoughts about affect, reason, risk, and rationality. Risk Analysis, 24, 311–322. https://doi.org/10.1111/j.0272-4332.2004.00433.x
Staerklé, C. (2015). Political Psychology. International Encyclopedia of the Social & Behavioral Sciences , 427–433. https://doi.org/10.1016/B978-0-08-097086-8.24079-8
Tremblay, S., Sharika, K. M., & Platt, M. L. (2017). Social decision-making and the brain: a comparative perspective. Trends in Cognitive Sciences, 21 (4), 265–276. https://doi.org/10.1016/j.tics.2017.01.007
Trepel, C., Fox, C. R., & Poldrack, R. A. (2005). Prospect theory on the brain? Toward a cognitive neuroscience of decision under risk. Brain Research. Cognitive Brain Research, 23 (1), 34–50. https://doi.org/10.1016/j.cogbrainres.2005.01.016
Van Der Pligt, J. (2015). Decision making, psychology of. International Encyclopedia of the Social & Behavioral Sciences, 2 (5), 917–922. https://doi.org/10.1016/B978-0-08-097086-8.24014-2
Von Neumann, J., & Morgenstern, O. (1944). Theory of games and economic behavior . Princeton University Press
Weber, E. U., & Hsee, C. K. (2000). Culture and individual judgment and decision making. Applied Psychology: An International Journal, 49, 32–61. https://doi.org/10.1111/1464-0597.00005
Weller, J. A., Levin, I. P., Shiv, B., & Bechara, A. (2009). The effects of insula damage on decision-making for risky gains and losses. Society for Neuroscience, 4 (4), 347–58. https://doi.org/10.1080/17470910902934400
Williams, D. J., & Noyes, J. M. (2007). How does our perception of risk influence decision-making? Implications for the design of risk information. Theoretical Issues in Ergonomics Science, 8, 1–35. https://doi.org/10.1080/14639220500484419
Yamada, H., Inokawa, H., Matsumoto, N., Ueda, Y., & Kimura, M. (2011). Neuronal basis for evaluating selected action in the primate striatum. European Journal of Neuroscience, 34 (3), 489–506. https://doi.org/10.1111/j.1460-9568.2011.07771.x
Download references
Author information
Authors and affiliations.
Dipartimento di Psicologia, Università di Roma “Sapienza”, Via dei Marsi. 78, 00185, Rome, Italy
Matteo Morelli & Giuseppe Forte
Dipartimento di Psicologia Dinamica, Clinica e Salute, Università di Roma “Sapienza”, Via degli Apuli, 1, 00185, Rome, Italy
Maria Casagrande
Body and Action Lab, IRCCS Fondazione Santa Lucia, Rome, Italy
Giuseppe Forte
You can also search for this author in PubMed Google Scholar
Corresponding authors
Correspondence to Maria Casagrande or Giuseppe Forte .
Additional information
Publisher’s note.
Springer Nature remains neutral with regard to jurisdictional claims in published maps and institutional affiliations.
Rights and permissions
Reprints and permissions
About this article
Morelli, M., Casagrande, M. & Forte, G. Decision Making: a Theoretical Review. Integr. psych. behav. 56 , 609–629 (2022). https://doi.org/10.1007/s12124-021-09669-x
Download citation
Accepted : 09 November 2021
Published : 15 November 2021
Issue Date : September 2022
DOI : https://doi.org/10.1007/s12124-021-09669-x
Share this article
Anyone you share the following link with will be able to read this content:
Sorry, a shareable link is not currently available for this article.
Provided by the Springer Nature SharedIt content-sharing initiative
- Decision making
- Neural correlates of decision making
- Decision-making tasks
- Decision-making theories
- Find a journal
- Publish with us
- Track your research
REVIEW article
The impact of cognitive biases on professionals’ decision-making: a review of four occupational areas.
- 1 Université de Lorraine, 2LPN, Nancy, France
- 2 Psychology and Neuroscience Lab, Centre d’Économie de la Sorbonne, Université de Lorraine, CNRS UMR 8174, Paris, France
The author reviewed the research on the impact of cognitive biases on professionals’ decision-making in four occupational areas (management, finance, medicine, and law). Two main findings emerged. First, the literature reviewed shows that a dozen of cognitive biases has an impact on professionals’ decisions in these four areas, overconfidence being the most recurrent bias. Second, the level of evidence supporting the claim that cognitive biases impact professional decision-making differs across the areas covered. Research in finance relied primarily upon secondary data while research in medicine and law relied mainly upon primary data from vignette studies (both levels of evidence are found in management). Two research gaps are highlighted. The first one is a potential lack of ecological validity of the findings from vignette studies, which are numerous. The second is the neglect of individual differences in cognitive biases, which might lead to the false idea that all professionals are susceptible to biases, to the same extent. To address that issue, we suggest that reliable, specific measures of cognitive biases need to be improved or developed.
Introduction
When making judgments or decisions, people often rely on simplified information processing strategies called heuristics, which may result in systematic, predictable errors called cognitive biases (hereafter CB). For instance, people tend to overestimate the accuracy of their judgments (overconfidence bias), to perceive events as being more predictable once they have occurred (hindsight bias), or to seek and interpret evidence in ways that are partial to existing beliefs and expectations (confirmation bias). In fact, the seminal work of Kahneman and Tversky on judgment and decision-making in the 1970s opened up a vast research program on how decision-making deviates from normative standards (e.g., Tversky and Kahneman, 1974 ; Kahneman et al., 1982 ; Gilovich et al., 2002 ).
The “heuristics and biases” program has been remarkably fruitful, leading to unveiling dozens of CB and heuristics in decision-making (e.g., Baron, 2008 , listed 53 such biases). While this research turned out to have a large impact in the academic field and beyond ( Kahneman, 2011 ), it is worth noting that it led to some debate ( Vranas, 2000 ; Pohl, 2017 ). In particular, Gigerenzer (1991 , 1996) ( Gigerenzer et al., 2008 ) outlined that Kahneman and Tversky relied upon a narrow view of normative rules (probability theory), leading them to ask participants to make artificial judgments (e.g., estimating the probability of single events) likely to result in so-called “errors.” Gigerenzer also pointed out the overemphasis on decision errors and the lack of theory behind the heuristics-and-biases approach, which eventually results in a list of cognitive errors with no theoretical framework. However, there have been several attempts to overcome this shortcoming, such as the reframing of the heuristics-and-biases literature in terms of the concept of attribute substitution ( Kahneman and Frederick, 2002 ) and the various taxonomies of CB advanced based on dual-process models (e.g., Stanovich et al., 2008 ).
While early research on CB was conducted on lay participants to investigate decision-making in general, there has been a large interest in how such biases may impede professional decision-making in areas, such as management (e.g., Maule and Hodgkinson, 2002 ), finance (e.g., Baker and Nofsinger, 2002 ), medicine (e.g., Blumenthal-Barby and Krieger, 2015 ), and law (e.g., Rachlinski, 2018 ). Consider, for example, the framing effect, when making risky decisions, people prefer sure gains over more risky ones, whereas they prefer risky losses over sure ones ( Kahneman and Tversky, 1979 ). Therefore, framing a problem in terms of gains versus losses can significantly impact decision-making. In most lawsuits for instance, plaintiffs choose between a sure gain (the settlement payment) and a potential larger gain (in the case of further litigation) while defendants choose between a sure loss (the settlement payment) and a potential larger loss (in the case of further litigation). In fact, when considering whether the parties should settle the case, judges evaluating the case from the plaintiff’s perspective are more likely to recommend settlement than those evaluating the case from the defendant’s perspective ( Guthrie et al., 2001 ). Likewise, when asking to rate the effectiveness of a drug, presenting the results of a hypothetical clinical trial in terms of absolute survival (gain), absolute mortality (loss), or relative mortality reduction (gain) influences the ratings of doctors ( Perneger and Agoritsas, 2011 ).
For the sake of convenience, we list below the common definition of the main CB considered in this review.
Anchoring Bias is the tendency to adjust our judgments (especially numerical judgments) toward the first piece of information ( Tversky and Kahneman, 1974 ).
Availability bias is the tendency by which a person evaluates the probability of events by the ease with which relevant instances come to mind ( Tversky and Kahneman, 1973 ).
Confirmation bias is the tendency to search for, to interpret, to favor, and to recall information that confirms or supports one’s prior personal beliefs ( Nickerson, 1998 ).
Disposition effect is the tendency among investors to sell stock market winners too soon and hold on to losers too long ( Shefrin and Statman, 1985 ). This tendency is typically related to loss aversion ( Kahneman and Tversky, 1979 ).
Hindsight bias is a propensity to perceive events as being more predictable, once they have occurred ( Fischhoff, 1975 ).
Omission bias is the preference for harm caused by omissions over equal or lesser harm caused by acts ( Baron and Ritov, 2004 ).
Outcome bias is the tendency to judge the quality of a decision based on the information about the outcome of that decision. These judgments are erroneous in respect to normative assumption that “information that is available only after decision is made is irrelevant to the quality of the decision” ( Baron and Hershey, 1988 , p. 569).
Overconfidence bias is a common inclination of people to overestimate their own abilities to successfully perform a particular task ( Brenner et al., 1996 ).
Relative risk bias is a stronger inclination to choose a particular treatment when presented with the relative risk than when presented with the same information described in terms of the absolute risk ( Forrow et al., 1992 ).
Susceptibility to framing is the tendency for people to react differently to a single choice depending on whether it is presented as a loss or a gain ( Tversky and Kahneman, 1981 ).
In the present paper, we review the research on the impact of CB on professional decision-making in four areas: management, finance, medicine, and law. Those applied areas were selected as they have led to the highest number of publications on this topic so far (see “Materials and Methods”). This study aims to address the following research questions:
1. Assess the claim that CB impact professionals’ decision-making
2. Assess the level of evidence reported in the empirical studies
3. Identify the research gaps
We take a narrative approach to synthesizing the key publications and representative empirical studies to answer these research questions. To the best of our knowledge, this study is the first literature review on this research topic covering multiple areas together. This review is narrative, as opposed to a systematic review, which is one of its limitations. However, it aims to be useful both to researchers and professionals working in the areas covered.
The present paper is structured as follows. The Methods section provides details about the methodology used to conduct the literature review. In the following sections, we review the key findings in each of the four occupational areas covered. Finally, in the Discussion section, we answer the three research questions addressed in light of the findings reviewed.
Materials and Methods
We conducted a systematic literature search using the Web of Science (WoS) database with the search terms “cognitive biases AND decision making.” The search criteria included research articles, review articles, or book chapters with no restriction regarding the time period. We focused on the WoS database as the “Web of Science Categories” filter would offer a practical mean to select the applied areas covered. Admittedly, the results of our review might have been different had we covered more databases; however, as our strategy was to review the key publications and representative empirical studies in each of the areas selected, we reasoned that virtually every database would have led to these records.
The PRISMA flowchart in Figure 1 illustrates the process of article search and selection in this study. The WoS search led to a total of 3,169 records. Before screening, we used the “Web of Science Categories” filter to identify and select the four applied areas with the highest number of publications. Those areas were management ( n = 436), which merged the categories “Management” ( n = 260) and “Business” ( n = 176); medicine ( n = 517), which merged the categories “Psychiatry” ( n = 261), “Health Care Sciences Services” ( n = 112), “Medicine General Internal” ( n = 94), “Radiology Nuclear Medicine Medical Imaging” ( n = 22), “Critical Care Medicine” ( n = 14), and “Emergency Medicine” ( n = 14); and law ( n = 110) and finance ( n = 70). Noteworthy, while the category “Psychology Applied” was associated with a significant number of publications ( n = 146), a closer examination revealed that the majority of them was related to other applied areas (e.g., management, medicine, law, and ergonomics). Accordingly, this category was not included in the review. The abstracts selected were reviewed according to two inclusion criteria: (1) the article had a clear focus on cognitive biases and decision-making (e.g., not on implicit biases); (2) the article reported a review (narrative or systematic) on the topic or a representative empirical study. This screening led to a selection of 79 eligible articles, which were all included in the review.
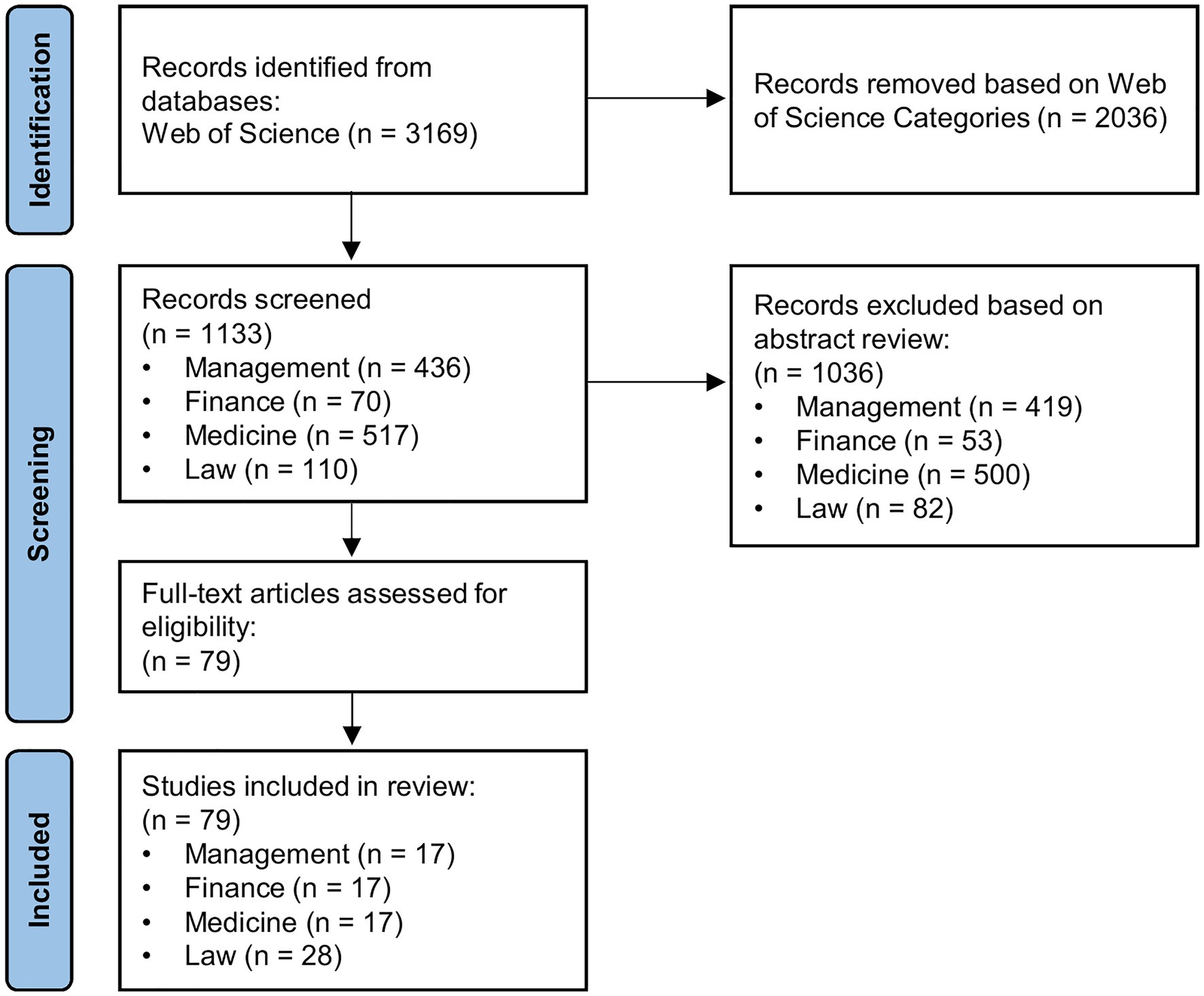
Figure 1 . PRISMA flowchart of article search and collection.
The life of any organization is made of crucial decisions. According to Eisenhardt and Zbaracki (1992 , p. 17), strategic decisions are “those infrequent decisions made by the top leaders of an organization that critically affect organizational health and survival.” For instance, when Disney decided to locate Euro Disney in Paris or when Quaker decided to acquire Snapple, these companies took strategic decisions.
A defining feature of strategic decisions is their lack of structure. While other areas of management deal with recurring, routinized, and operationally specific decisions, strategic issues and problems tend to be relatively ambiguous, complex, and surrounded by risk and uncertainty ( Hodgkinson, 2001 ). How do managers actually deal with such decisions? Much of early research on strategic decision-making was based on a neoclassical framework with the idea that strategists in organizations are rational actors. However, the seminal work of Kahneman and Tversky in the 1970s questioned this assumption ( Hardman and Harries, 2002 ). In fact, the very notion of “bounded rationality” emerged in the study of organizations ( March and Simon, 1958 ). One might argue that the issue of individual biases in strategic decision-making is of limited relevance as strategic decisions are the product of organizations rather than individuals within the context of a wider sociopolitical arena ( Mintzberg, 1983 ; Johnson, 1987 ). However, individual (micro) factors might help explain organizational (macro) phenomena, an idea promoted by behavioral strategy ( Powell et al., 2011 ).
The “heuristics and biases” program revived the interest for bounded rationality in management with the idea that decision-makers may use heuristics to cope with complex and uncertain environments, which in turn may result in inappropriate or suboptimal decisions (e.g., Barnes, 1984 ; Bazerman, 1998 ). Indeed, it is relatively easy to see how biases, such as availability, hindsight, or overconfidence, might play out in the strategic decision-making process. For instance, it may seem difficult in hindsight to understand why IBM and Kodak failed to see the potential that Haloid saw (which led to the Xerox company). The hindsight bias can actually lead managers to distort their evaluations of initial decisions and their predictions ( Bukszar and Connolly, 1988 ). Likewise, practicing auditors of major accounting firms are sensitive to anchoring effects ( Joyce and Biddle, 1981 ) and prospective entrepreneurs tend to neglect base rates for business failures ( Moore et al., 2007 ).
To our knowledge, no systematic review of empirical research on the impact of heuristics and CB on strategic decision-making has been published to date. Whereas the idea that CB could affect strategic decisions is widely recognized, the corresponding empirical evidence is quite weak. Most research on this topic consists in narrative papers relying upon documentary sources and anecdotal evidence (e.g., Duhaime and Schwenk, 1985 ; Lyles and Thomas, 1988 ; Huff and Schwenk, 1990 ; Zajac and Bazerman, 1991 ; Bazerman and Moore, 2008 ). In fact, the typical paper describes a few CB and provides for each one examples of how a particular bias can lead to poor strategic decisions (see Barnes, 1984 , for a representative example). While the examples provided are often compelling, such research faces severe methodological limitations.
The work of Schwenk (1984 , 1985) is representative of that type of research. This author identified three different stages of the strategic decision process (goal formulation and problem identification, strategic alternatives generation, evaluation of alternatives, and selection of the best one) and a set of heuristics and biases that might affect decisions at each stage. Schwenk also provided for each bias an illustrative example of how the bias may impede the overall quality of strategic decisions. For example, the representativeness heuristics may affect the stage of evaluation and selection of the alternatives. To illustrate this, Schwenk mentioned the head of an American retail organization (Montgomery Ward) who held a strong belief that there would be a depression at the end of the Second World War as was the case after World War I. Based on this belief, this executive decided not to allow his company to expand to meet competition from his rival (Sears), which led to a permanent loss of market share to Sears. Schwenk (1988) listed ten heuristics and biases of potential key significance in the context of strategic decision-making (availability, selective perception, illusory correlation, conservatism, law of small numbers, regression bias, wishful thinking, illusion of control, logical reconstruction, and hindsight bias).
In a similar vein, Das and Teng (1999) proposed a framework to explore the presence of four basic types of CB (prior hypotheses and focusing on limited targets, exposure to limited alternatives, insensitivity to outcome probabilities, and illusion of manageability) under five different modes of decision-making (rational, avoidance, logical incrementalist, political, and garbage can). They proposed that not all basic types of biases are robust across all kinds of decision processes; rather, their selective presence is contingent upon the specific processes that decision makers engage in. For instance, the garbage can mode ( Cohen et al., 1972 ) depicts decision-making processes as organized anarchies, in which a decision is largely dependent on chance and timing. In this kind of process, decision makers do not know their objectives ex ante , but merely look around for decisions to make. Das and Teng (1999) hypothesized that managers under the garbage can mode will be exposed to limited alternatives and insensitive to outcome probabilities. On the contrary, managers under the rational mode would be exposed to prior hypotheses and illusion of manageability. This framework, however, is not supported by rigorous empirical evidence.
It is not difficult to list examples of poor strategic decisions that can be readily interpreted – in hindsight – as the result of heuristics and biases. However, the claim that CB influence strategic decisions requires to be tested more directly through laboratory research and experimental studies ( Maule and Hodgkinson, 2002 ). It is worth noting that such research is scarce, probably because of its lack of ecological validity, an issue of primary importance in the field of management research ( Schwenk, 1982 ). Still, two CB in particular have been studied quantitatively, the framing effect and CEO overconfidence.
Hodgkinson et al. (1999) used an experimental setting to investigate the effect of framing on strategic decisions. Following the “Asian Disease” problem ( Tversky and Kahneman, 1981 ), they presented subjects (undergraduate management students) with a 500-word case vignette giving a brief history of a company that manufactured and distributed fast paint-drying systems. A positive and a negative frame were used and participants were asked to adopt the role of a board member facing a major strategic decision and to indicate which of two alternative options they would choose. The positive frame emphasized gains from a reference point of no profit, whereas the negative frame highlighted losses from a reference point where the target profit is achieved (£3 million). In addition, participants were either asked to choose between the presented options directly or to represent the ways in which they thought about the problem in the form of a causal map prior to making their choice. It turned out that when participants made their decisions directly, a massive framing effect was found (45.5% of participants chose the risk-averse option in the positive frame versus 9% in the negative frame). However, no framing effect was observed when participants were asked to draw a causal map before making their choice (36.4% of the participants opted for the risk-averse option in both versions). Interestingly, Hodgkinson et al. reported the same findings on experienced participants (senior managers in a banking organization).
Another CB that led to a large amount of empirical research in strategic management is CEO overconfidence. Overconfidence has various aspects: overprecision, overestimation, and overplacement ( Moore and Schatz, 2017 ). Regarding overprecision, Ben-David et al. (2013) investigated the accuracy of stock market predictions made by senior finance executives (the majority of them being CFOs). The data were collected in 40 quarterly surveys conducted between June 2001 and March 2011. Ben-David et al. asked participants to predict one- and 10-year market-wide stock returns and to provide an 80% confidence interval for their predictions (“Over the next year, I expect the annual SandP 500 return will be: There is a 1-in-10 chance the actual return will be less than ___%; I expect the return to be: ___%; There is a 1-in-10 chance the actual return will be greater than ___%.”). It turned out that the CFOs were severely miscalibrated as: the realized one-year SandP 500 returns fall within their 80% confidence intervals only 36.3% of the time. Even during the least volatile quarters in the sample, only 59% of realized returns fall within the 80% confidence intervals provided. The comparison of the size of the CFOs’ confidence intervals to the distribution of historical one-year returns revealed that their confidence intervals were too narrow. Indeed, CFOs provide an average confidence interval of 14.5%, whereas the difference between the 10th and 90th return percentiles from the realized distribution of the one-year SandP 500 returns is 42.2% (only 3.4% of CFOs provided confidence intervals wider than 42.2%).
Managers also overestimate their abilities, particularly with regard to the illusion of control. In their review on risk perception among managers, March and Shapira (1987) reported that most managers (1) consider that they take risks wisely and that they are less risk-averse than their colleagues, (2) perceive risk as largely controllable, and (3) attribute this controllability to skills and information.
Finally, executives also appear to be overconfident with regard to overplacement. Malmendier and Tate (2005) assessed CEO overconfidence through revealed preferences, examining how they exercised their options. A CEO persistently exercising options later than suggested by the benchmark reveals his belief in his ability to keep the company’s stock price rising and that he or she wants to profit from expected price increases by holding the options. Using panel data on personal portfolio and corporate investment decisions of Forbes 500 CEOs, Malmendier and Tate reported that most of CEO excessively hold company stock options, thereby failing to reduce their personal exposure to company-specific risk. CEO overconfidence is also believed to be involved in merger decisions. As overconfident CEOs overestimate their ability to generate returns, they are supposed to overpay for target companies and undertake value-destroying mergers. Using two measures of CEO overconfidence (CEOs’ personal over-investment in their company and their press portrayal), Malmendier and Tate (2008) provided support for that hypothesis: the odds of making an acquisition are 65% higher if the CEO is classified as overconfident.
The case of CB in finance is special. In the 1980s, CB were invoked to account for observations on markets in disagreement with the predictions of standard finance. This paradigm relies upon expected utility theory, assuming that investors make rational decisions under uncertainty (i.e., maximizing utility). Standard finance produced core theoretical concepts, such as arbitrage, portfolio theory, capital asset pricing theory, and efficient market hypothesis, all assuming rational investors. In the 1970s, some observations on financial markets relative to trading behavior, volatility, market returns, and portfolio selection turned out to be inconsistent with the framework of standard finance (“anomalies”). Psychological biases (micro level) were invoked as theoretical explanations of these market anomalies (macro level), launching the field of behavioral finance ( Shiller, 2003 ). In particular, behavioral finance capitalized on prospect theory ( Kahneman and Tversky, 1979 ), a more realistic view of decision-making under uncertainty that expected utility theory. A prime example is how (myopic) loss aversion – a key concept of prospect theory – can account for the equity premium puzzle (i.e., the excessively high difference between equity returns and the return of Treasury bills; Benartzi and Thaler, 1995 ).
Here, we focus on investment decision-making in individual investors ( Shefrin, 2000 ; Baker and Nofsinger, 2010 ; Baker and Ricciardi, 2014 ) and how CB may impede such decisions (see Baker and Nofsinger, 2002 , and Kumar and Goyal, 2015 , for reviews). 1 In fact, financial economists have distinguished between two types of investors in the market, arbitrageurs and noise traders. While the latter is assumed to be fully rational, noise traders are investors prone to CB ( De Long et al.,1990 ), which results in under-diversified portfolios. Various CB have been invoked to account for poor individual investment decisions, resulting in suboptimal portfolio management. For example, investors tend to favor stocks that performed well during the past 3–5 years (“winners”) over stocks that performed poorly (“losers”), neglecting that because of regression to the mean, the losers will tend to outperform the winners over the next years (actually by 30%; De Bondt and Thaler, 1985 ). Investors may exhibit a home bias (an instance of familiarity bias), a tendency to invest the majority of their portfolio in domestic equities rather than diversifying it into foreign equities ( Coval and Moskowitz, 1999 ). Investors may also fall prey to herding, a tendency to follow blindly what other investors do ( Grinblatt et al., 1995 ).
Two CB have been particularly studied in investment decision-making: overconfidence and disposition effect (see the systematic review of Kumar and Goyal, 2015 ). On the one hand, investors are usually overconfident with regard to the precision of their forecasts. When asked to predict the future return or price of a stock, investors report confidence intervals that are too narrow compared to the actual variability of prices (e.g., De Bondt, 1998 ). Investors also overestimate their ability to beat the market. Baker and Nofsinger (2002) reported a finding of a Gallup survey in 2001 revealing that on average, investors estimated that the stock market return during the next 12 months would be 10.3% while estimating that their portfolio return would be 11.7%. Barber and Odean (2001) reported evidence that overconfidence in investors is related to gender. Based on a sample of 35,000 individual accounts over a six-year period, their findings showed that males exhibit more overconfidence regarding their investing abilities and also trade more often than females. Overconfidence in investors makes them more prone to take high risks ( Chuang and Lee, 2006 ) and trade too much ( Odean, 1999 ; Statman et al., 2006 ; Glaser and Weber, 2007 ), which results in poor financial performance (consequent transaction costs and losses). For instance, trading turnover and portfolio returns are negatively correlated: of 66,465 households with accounts at a large discount broker during 1991–1996, households that trade most had an annual return of 11.4% while the average annual return was 16.4% ( Barber and Odean, 2000 ).
On the other hand, the disposition effect is the tendency by which investors tend to sell winning stocks too early while holding on to losing positions for too long ( Shefrin and Statman, 1985 ). Based on trading records for 10,000 accounts at a large discount brokerage house, Odean (1998) reported that on average, winning investments are 50% more likely to be sold than losing investment (similar results were obtained in other countries, such as France; Boolell-Gunesh et al., 2009 ). The disposition effect originates in loss aversion described by prospect theory ( Kahneman and Tversky, 1979 ).
The idea that cognitive failures are a primary source of medical errors has become prevalent in the medical literature (e.g., Detmer et al., 1978 ; Dawson and Arkes, 1987 ; Schmitt and Elstein, 1988 ; Elstein, 1999 ; Croskerry, 2003 ; Klein, 2005 ). In fact, emergency medicine has been described as a “natural laboratory of error” ( Bogner, 1994 ). Among medical errors, diagnostic errors have received particular attention ( Graber, 2013 ). Indeed, there is increasing evidence that mental shortcuts during information processing contribute to diagnostic errors (e.g., Schnapp et al., 2018 ).
It is not difficult to see how CB may impact medical decisions. Blumenthal-Barby and Krieger (2015) provided the following examples. A parent might refuse to vaccinate her child after she sees a media report of a child who developed autism after being vaccinated (availability bias). A patient with atrial fibrillation might refuse to take warfarin because she is concerned about causing a hemorrhagic stroke despite greater risk of having an ischemic stroke if she does not take warfarin (omission bias). Indeed, early papers on this topic were primarily narrative reviews suggesting a possible impact of CB on medical decision-making. These papers follow the same logic: they first provide a general description of a couple of CB and then describe how these shortcuts can lead physicians to make poor decisions, such as wrong diagnoses (e.g., Dawson and Arkes, 1987 ; Elstein, 1999 ; Redelmeier, 2005 ). But narrative reviews provide limited evidence. As Zwaan et al. (2017 , p.105) outlined, “While these papers make a formidable argument that the biases described in the literature might cause a diagnostic error, empirical evidence that any of these biases actually causes diagnostic errors is sparse.”
On the other hand, studies that investigated the actual impact of CB on medical decisions are mainly experimental studies using written cases (hypothetical vignettes) designed to elicit a particular bias. A typical example of vignette study is that of Mamede et al. (2010) on the effect of availability bias on diagnostic accuracy. In a first phase, participants (first-year and second-year internal medicine residents) were provided with 6 different cases and they were asked to rate the likelihood that the indicated diagnosis was correct (all cases were based on real patients with a confirmed diagnosis). Then, participants were asked to diagnose 8 new cases as quickly as possible, that is, relying on non-analytical reasoning. Half of those new cases were similar to the cases encountered in phase 1, so that the availability bias was expected to reduce diagnostic accuracy for those four cases. Second-year residents had actually lower diagnostic accuracy on cases similar to those encountered in phase 1 as compared to other cases, as they provided the phase 1 diagnosis more frequently for phase 2 cases they had previously encountered than for those they had not.
While vignette-based studies are the most frequent, researchers in this area have used diverse strategies ( Blumenthal-Barby and Krieger, 2015 ). For instance, Crowley et al. (2013) developed a computer-based method to detect heuristics and biases in diagnostic reasoning as pathologists examine virtual slide cases. Each heuristic or bias is defined as a particular sequence of hypothesis, findings, and diagnosis formulation in the diagnostic reasoning interface (e.g., availability bias is considered to occur if in a sequence of three cases where the third case has a different diagnosis than the two previous ones, the participant makes an incorrect diagnosis in the third case such that the diagnosis is identical to the correct diagnosis in the two immediately preceding cases). Such a procedure allows for examining the relationships between heuristics and biases, and diagnostic errors.
Another methodology consists in reviewing instances where errors occurred, to which CB presumably contributed (e.g., Graber et al., 2005 ). However, studies following this methodology are vulnerable to hindsight bias: since reviewers are aware that an error was committed, they are prone to identify biases ex post ( Wears and Nemeth, 2007 ). The fact that bias can be in the eye of the beholder has been supported by Zwaan et al. (2017) who asked 37 physicians to read eight cases and list which CB were present from a list provided. In half the cases, the outcome implied a correct diagnosis; in the other half, it implied an incorrect diagnosis. Physicians identified more biases when the case outcome implied an incorrect diagnosis (3.45 on average) than when it implied a correct one (1.75 on average).
To date, two systematic reviews have been published on the impact of CB on medical decision-making. Reviewing a total of 213 studies, Blumenthal-Barby and Krieger (2015) reported the following findings: (1) 77% of the studies ( N = 164) were based on hypothetical vignettes; (2) 34% of studies ( N = 73) investigated medical personnel; (3) 82% of the studies ( N = 175) were conducted with representative populations; (4) 68% of the studies ( N = 145 studies) confirmed a bias or heuristic in the study population; (5) the most studied CB are loss/gain framing bias (72 studies, 24.08%), omission bias (18 studies, 6.02%), relative risk bias (29 studies, 9.70%), and availability bias (22 studies, 7.36%); (6) the results regarding loss/gain framing bias are mixed with 39% of studies ( N = 28) confirming an effect, 39% ( N = 28) confirming an effect only in a subpopulation, and 22% ( N = 16) disconfirming any effect; (7) 25 of 29 studies (86%) supported the impact of relative risk bias on medical decisions; and (8) 14 of 18 studies (78%) supported the impact of omission bias on medical decisions.
Saposnik et al. (2016) conducted a similar review but including only 20 studies. These authors reported that as: (1) 60% of the studies ( N = 12) targeted CB in diagnostic tasks; (2) framing effect ( N = 5) and overconfidence ( N = 5) were the most common CB while tolerance to risk or ambiguity was the most commonly studied personality trait ( N = 5); and (3) given that the large majority of the studies (85%) targeted only one or two biases, the true prevalence of CB influencing medical decisions remains unknown. Moreover, there was a wide variability in the reported prevalence of CB. For example, when analyzing the three most comprehensive studies that accounted for several CB ( Ogdie et al., 2012 ; Stiegler and Ruskin, 2012 ; Crowley et al., 2013 ), it turned out that the availability bias ranged from 7.8 to 75.6% and anchoring bias from 5.9 to 87.8%; (4) the presence of CB was associated with diagnostic inaccuracies in 36.5 to 77% of case-scenarios. Physicians’ overconfidence, anchoring effect, and information or availability bias may be associated with diagnostic inaccuracies; (5) only seven studies (35%) provided information to evaluate the association between physicians’ CB and therapeutic or management errors. Five of these studies (71.4%) showed an association between CB (anchoring, information bias, overconfidence, premature closure, representativeness, and confirmation bias) and therapeutic or management errors.
Based on the legal realism’ premise that “judges are human,” the recent years have seen a growing interest for judicial decision-making (e.g., Klein and Mitchell, 2010 ; Dhami and Belton, 2017 ; Rachlinski, 2018 ). This topic covers issues, such as cognitive models of judicial decision-making (e.g., the story model), the impact of extralegal factors on decisions, prejudice (e.g., gender bias and racial bias), moral judgments, group decision-making, or the comparison of lay and professional judges. It is worth noting that most research on judicial decision-making has focused on how jurors decide cases, relying on jury simulations ( MacCoun, 1989 ). Here, we focus on how professional judges might be prone to CB. One might easily consider how CB could hamper judicial decisions. In a narrative fashion, Peer and Gamliel (2013) reviewed how such biases could intervene during the hearing process (confirmation bias and hindsight bias), ruling (inability to ignore inadmissible evidence), and sentencing (anchoring effects). In fact, research suggests that judges, prosecutors, and other professionals in the legal field might rely on heuristics to produce their decisions, which leaves room for CB (e.g., Guthrie et al., 2007 ; Helm et al., 2016 ; Rachlinski and Wistrich, 2017 ). 2
Researchers investigating judges’ decision-making have mainly relied upon archival studies (document analyses of court records) and experimental studies in which judges are asked to decide on hypothetical cases. In archival studies, researchers examine if judges’ decisions in actual cases exhibit features of irrationality. For instance, Ebbesen and Konecni (1975) investigated which information felony court judges considered when deciding the amount of bail to set. When presented with fictitious cases, the judges’ decisions were influenced by relevant information, such as prior criminal record, but their actual bail decisions relied almost exclusively on prosecutorial recommendations. That is, judges seem to be (too) heavily affected by prosecutors’ recommendations. Another example of archival study is the infamous research of Danziger et al. (2011) who highlighted a cycle in repeated judicial rulings: judges are initially lenient, then progressively rule more in favor of the status quo over time, and become lenient again after a food break. This would suggest that psychological factors, such as mental fatigue, could influence legal decisions (but see Weinshall-Margel and Shapard, 2011 ). Archival studies, however, are limited by the difficulty to control for unobserved variables.
On the other hand, vignette studies consist in presenting judges with hypothetical scenarios simulating real legal cases. As in the medical field, researchers have primarily relied on such studies. A representative study is that of Guthrie et al. (2001) who administered a survey to 167 federal magistrate judges in order to assess the impact of five CB (anchoring, framing, hindsight bias, inverse fallacy, and egocentric bias) on their decisions regarding litigation problems (see Guthrie et al., 2002 , for a summary of the research). Using materials adapting classic cognitive problems into legal ones, Guthrie et al. (2001) reported that judges fell prey to these biases but to various extent. For instance, in order to assess whether judges were susceptible to hindsight bias, Guthrie et al. (2001) presented them with a hypothetical case in which the plaintiff appealed the district court’s decision and asked them to indicate which of three possible outcomes of the appeal was most likely to have occurred. Crucially, they also provided them with the actual outcome of the court of appeals. The outcome significantly influenced judges’ assessments: those informed of a particular outcome were more likely to have identified that outcome as the most likely to have occurred.
In particular, numerous studies have investigated the impact of anchoring effects on judicial decisions (see Bystranowski et al., 2021 , for a recent meta-analysis). Judges and jurors are often required to translate qualitative judgments into quantitative decisions ( Hans and Reyna, 2011 ; Rachlinski et al., 2015 ). While their qualitative judgments on matters, such as the severity of the plaintiff’s injury or the appropriate severity of punishment, show a high degree of consistence and predictability ( Wissler et al., 1999 ), a great amount of variability appears (especially for non-economic and punitive damages) when these qualitative judgments are translated into numbers (e.g., civil damage awards and criminal sentences; Hart et al., 1997 ; Diamond et al., 1998 ). This might be explained by the fact that numerical assessments can be prone to anchoring. Facing uncertainty about the amount to determine, judges and especially juries (due to their lack of experience and information about standard practice) tend to rely on any numerical point of reference and make their judgment through adjustments from that number. As these adjustments are often insufficient, the judgments are biased toward the anchor (see Kahneman et al., 1998 , for a model describing how individual jurors set punitive damages and the role of anchoring in that process).
Accordingly, numerical values, such as a damage cap (e.g., Hinsz and Indahl, 1995 ; Robbennolt and Studebaker, 1999 ), the amount of damages claimed by the plaintiff ( Chapman and Bornstein, 1996 ), the amount of economic damage ( Eisenberg et al., 1997 , 2006 ), the sentence imposed in the preceding case, a sentence urged by the prosecutor, or a sentence recommended by a probation officer, might act as anchors in the courtroom, moving the judges’ decisions toward them. Guthrie et al. (2001) reported that in a personal injury suit, an irrelevant factor, such as a number in a pre-trial motion (used to determine whether the damages met the minimum limit for federal court), could act as an anchor. They presented judges with a description of a serious personal injury suit in which only damages were at issue and asked them to estimate how much they would award the plaintiff in compensatory damages. Prior to this estimation, half of the judges were asked to rule on a pre-trial motion filed by the defendant to have the case dismissed for failing to meet the jurisdictional minimum in a diversity suit ($75,000). It turned out that the judges who were asked only to determine the damage award provided an average estimate of $1,249,000 while the judges who first ruled on the motion provided an average estimate of $882,000.
Enough and Mussweiler (2001) conducted a series of research on how recommendations anchor judicial decisions, even when they are misleading. In their 2001 paper, they showed that sentencing decisions tend to follow the sentence demanded by the prosecutor. When told that the prosecutor recommended a sentence of 34 months, criminal trial judges recommended on average 8 months longer in prison ( M = 24.41 months) than when told that the sentence should be 12 months ( M = 17.64) for the same crime. This anchoring effect was independent of the perceived relevance of the sentencing demand, and judges’ experience. Englich et al. (2006) reported that anchoring even occurs when the sentencing demand is determined randomly (the result of a dice throw). Interestingly, Englich et al. (2005) found that the defense’s sentencing recommendation is actually anchored on the prosecutor’s demand, so that the former mediates the impact of the latter on the judge’s decision. Therefore, while it is supposed to be at their advantage, the fact that defense attorneys present their sentencing recommendation after the prosecution might be a hidden disadvantage for the defense.
Along with anchoring, the impact of hindsight bias in the courtroom has been also well documented, mainly in liability cases ( Harley, 2007 ; Oeberst and Goeckenjan, 2016 ). When determining liability or negligence, judges and juries must assess whether the defendant is liable for a negative outcome (damage or injury). The difficulty is that jurors accomplish this task in retrospect: having knowledge of the outcome, jurors tend to perceive it as foreseeable and accordingly rate highly the negligence or liability of the defendant ( Rachlinski et al., 2011 ). To avoid this bias, the law requires jurors to ignore the outcome information while evaluating the extent to which it should have been foreseen by the defendant. However, research suggests that jurors tend to fall prey to hindsight bias as much as lay persons. When evaluating the precautions took by a municipality to protect a riparian property owner from flood damage, participants assessing the situation in foresight concluded that a flood was too unlikely to justify further precautions. However, participants assessing the situation in hindsight considered that such a decision was negligent and also gave higher estimates for the probability of the disaster occurring ( Kamin and Rachlinski, 1995 ).
Outcome information has been shown to affect jurors’ decisions about punitive damage awards ( Hastie et al., 1999 ) and their decisions about the legality of a search ( Casper et al., 1989 ). In addition, more severe outcomes tend to produce a larger hindsight bias, a result particularly stressed in medical malpractice litigation ( LaBine and LaBine, 1996 ). While the assessment of negligence of the accused physician should be based on his course of action regardless of the outcome, jurors are highly influenced by the severity of a negative medical outcome when determining negligence in medical malpractice cases ( Berlin and Hendrix, 1998 ). Cheney et al. (1989) reviewed 1,004 cases of anesthesia-related negligence and reported that the court had imposed liability on the defendant in over 40 percent of the cases, even though the physician acted appropriately.
There is also significant evidence that confirmation bias ( Nickerson, 1998 ) may impact professional judges’ decisions. In the legal field, confirmation bias has been primarily studied with regard to criminal investigations ( Findley and Scott, 2006 ). Once they become convinced that the suspect is guilty, professionals involved in criminal proceedings (e.g., police officers and judges) may engage in guilt-confirming investigation endeavors (or tunnel vision) by which they undermine alternative scenarios in which the suspect is actually innocent. Several studies reported evidence of confirmation bias in criminal cases. For instance, O’Brien (2009) found that participants (College students) who articulated a hypothesis regarding the suspect early in their review of a mock police file showed bias in seeking and interpreting evidence to favor that hypothesis, thereby demonstrating a case-building mentality against a chosen suspect. Similarly, Lidén et al. (2019) showed that judges’ detentions of suspects trigger a confirmation bias that influences their assessment of guilt and that this bias is affected by who decided about detention. In fact, judges perceived the detained defendants’ statements as less trustworthy and were also more likely to convict when they themselves had previously detained the suspect as compared to when a colleague had decided to detain. 3
Table 1 provides a summary of the main CB in the four occupational areas reviewed and the corresponding evidence.
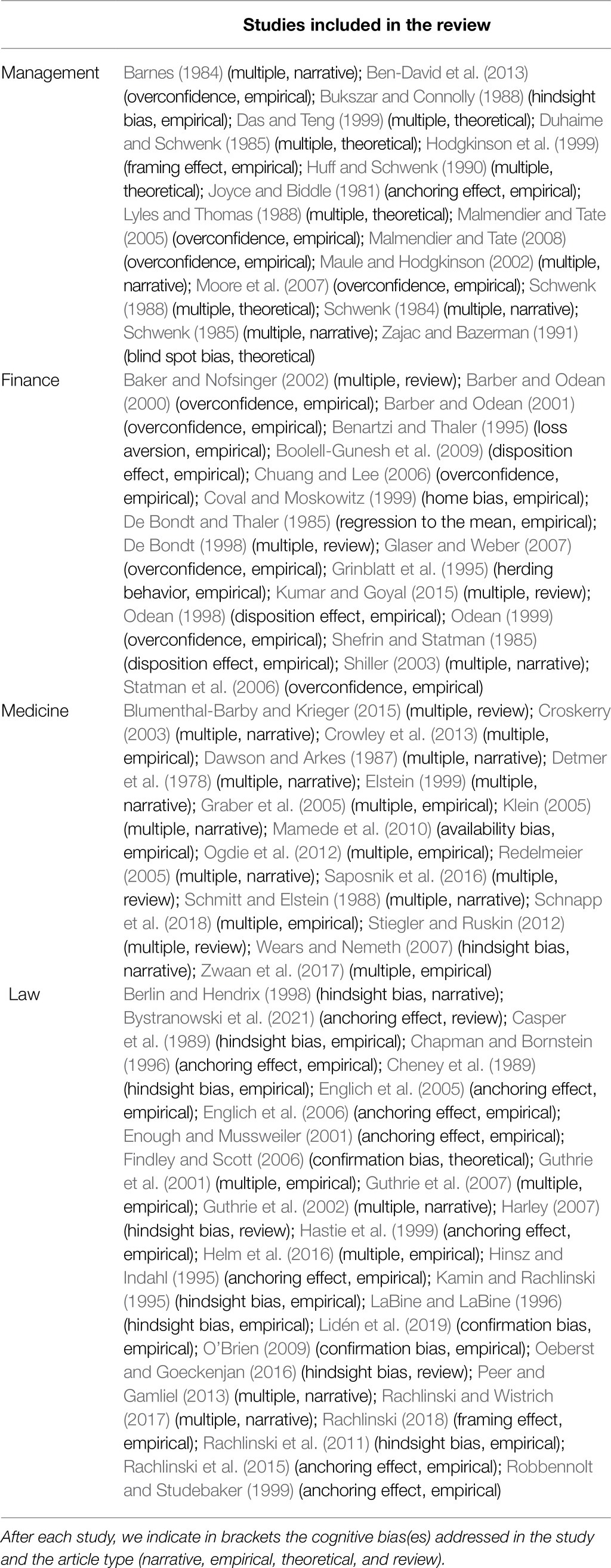
Table 1 . Summary of the main cognitive biases studied in the fields of management, finance, medicine, and law, and corresponding evidence.
The goal of the present paper was to provide an overview of the impact of CB on professional decision-making in various occupational areas (management, finance, medicine, and law). In all of them, there has been tremendous interest in that issue as revealed by a vast amount of research. Our review provided significant answers to the three research questions addressed.
First, the literature reviewed shows that, overall, professionals in the four areas covered are prone to CB. In management, there is evidence that risky-choice (loss/gain) framing effects and overconfidence (among CEOs) impact decision-making. In finance, there is strong evidence that overconfidence and the disposition effect (a consequence of loss aversion) impact individual investors’ decision-making. Regarding medical decision-making, the systematic review of Blumenthal-Barby and Krieger (2015) revealed that (1) 90% of the 213 studies reviewed confirmed a bias or heuristic in the study population or in a subpopulation of the study; (2) there is strong evidence that omission bias, relative risk bias, and availability bias have an impact on medical decisions, and mixed evidence for the risky-choice framing effect. On the other hand, the systematic review of Saposnik et al. (2016) – based on 20 studies only – reported that physicians’ overconfidence, anchoring, and availability bias were associated with diagnostic errors. Finally, the effects of anchoring, hindsight bias, and confirmation bias on judicial decision-making are well documented. Overall, overconfidence appears as the most recurrent CB over the four areas covered.
Second, the level of evidence supporting the claim that CB impact professionals’ decision-making differs across the four areas covered. In medicine and law, this issue has been primarily evidenced in vignettes studies. Such primary data provide a relevant assessment of CB in decision-making but they face the issue of ecological validity (see below). Accordingly, a mid-level of evidence can be assigned to these findings. On the other hand, following the method of revealed preference by which the preferences of individuals are uncovered through the analysis of their choices in real-life settings, the impact of CB on financial decision-making has been evidenced through secondary data (e.g., trading records), indicating a higher level of evidence. In management, both levels of evidence are found (framing effects were demonstrated in vignette studies while CEO overconfidence was evidenced through secondary data).
A practical implication of these findings is the need for professionals to consider concrete, practical ways of mitigating the impact of CB on decision-making. In finance, this issue has been tackled with programs that aimed to improve financial literacy ( Lusardi and Mitchell, 2014 ). In medicine, debiasing has been considered as a way to reduce the effects of CB ( Graber et al., 2002 , 2012 ; Croskerry, 2003 ; Croskerry et al., 2013 ). In fact, recent research has reported evidence that the debiasing of decisions can be effective ( Morewedge et al., 2015 ; Sellier et al., 2019 ). However, a preliminary step to considering practical means of mitigating the impact of CB is to acknowledge this diagnosis. In fact, professionals are reluctant to accept the idea that their decisions may be biased (e.g., Kukucka et al., 2017 ). Judges, for instance, tend to dismiss the evidence showing the impact of CB on judicial decisions, arguing that most studies did not investigate decisions on real cases ( Dhami and Belton, 2017 ).
Thirdly, our review highlights two major research gaps. The first one is a potential lack of ecological validity of the findings from vignette studies, which are numerous ( Blumenthal-Barby and Krieger, 2015 ). Consider for instance a study designed to test whether sentencing decisions could be anchored by certain information, such as the sentence demanded by the prosecutor ( Enough and Mussweiler, 2001 ). A typical study consists in presenting judges with a vignette describing a hypothetical criminal case and asking them to sentence the defendant (e.g., Rachlinski et al., 2015 ). If a statistically significant difference is observed between the different anchor conditions, it is concluded that anchoring impacts judges’ sentencing decisions. Does such a finding mean that judges’ sentencing decisions in real cases are affected by anchoring too? Likewise, it has been reported that 90% of judges solve the Wason task incorrectly ( Rachlinski et al., 2013 ) but this does not imply per se that confirmation bias impedes judges’ decisions in their regular work. Addressing that issue requires to use more ecological settings, such as mock trials in the case of judicial decision-making ( Diamond, 1997 ).
The second research gap is the neglect of individual differences in CB. This limit was found in the four areas covered. Individual differences have been neglected in decision-making research in general ( Stanovich et al., 2011 ; Mohammed and Schwall, 2012 ). Indeed, most of the current knowledge about the impact of CB on decision-making relies upon experimental research and group comparisons ( Gilovich et al., 2002 ). For instance, based on the experimental result described above, one might wrongly infer that all judges are susceptible to anchoring, to the same extent. That is why Guthrie et al. (2007 , p. 28) clarified that “the fact that we generally observed statistically significant differences between the control group judges and experimental group judges does not mean that every judge made intuitive decisions. […] Our results only show that, as a group, the judges were heavily influenced by their intuition – they do not tell us which judges were influenced and by how much.” In fact, there is clear evidence for individual differences in susceptibility to CB (e.g., Bruine de Bruin et al., 2007 ).
The issue of individual differences is of primary importance when considering CB in decision-making, especially among professionals. In finance for example, the measurement of the disposition effect at the individual level revealed significant individual differences, 20% of investors showing no disposition effect or a reverse effect ( Talpsepp, 2011 ). Taking full account of individual differences is crucial when considering public interventions aiming to mitigate individual biases: any single intervention might work on individuals highly susceptible to the bias addressed while having no or even harmful effects on individuals moderately susceptible to it ( Rachlinski, 2006 ).
Addressing the issue of individual differences in bias susceptibility requires having standardized, reliable measures ( Berthet, 2021 ). While reliable measures of a dozen CB are currently available, measures of key biases are still lacking (e.g., confirmation bias and availability bias). Most importantly, these measures are generic, using non-contextualized items. Such measures are relevant for research with the purpose of describing general aspects of decision-making ( Parker and Fischhoff, 2005 ; Bruine de Bruin et al., 2007 ). However, research on individual differences in professional decision-making requires specific measures which items are adapted to the context in which a particular decision is made (e.g., diagnostic decision and sentencing decision). An example is the inventory of cognitive biases in medicine ( Hershberger et al., 1994 ) which aims to measure 10 CB in doctors (e.g., insensitivity to prior probability and insensitivity to sample size) through 22 medical scenarios. The development of such instruments in the context of management, finance, and law is an important avenue for future research on professional decision-making.
Author Contributions
The author confirms being the sole contributor of this work and has approved it for publication.
Conflict of Interest
The author declares that the research was conducted in the absence of any commercial or financial relationships that could be construed as a potential conflict of interest.
Publisher’s Note
All claims expressed in this article are solely those of the authors and do not necessarily represent those of their affiliated organizations, or those of the publisher, the editors and the reviewers. Any product that may be evaluated in this article, or claim that may be made by its manufacturer, is not guaranteed or endorsed by the publisher.
1. ^ It should be noted that most research in behavioral finance has focused on individual rather than professional investors (e.g., mutual funds, hedge funds, pension funds, and investment advisors). Findings suggest that institutional investors are prone to various CB but to a lesser extent than individual investors (e.g., Kaustia et al., 2008 ).
2. ^ Interestingly, the notion of cognitive bias might also shed light on certain rules of law. For example, Guthrie et al. (2001) presented judges with a problem based on the classic English case Byrne v. Boadle (1863) asked them to assess the likelihood that a warehouse was negligent for an accident involving a barrel that injured a bystander. The materials indicated that when the warehouse is careful, accidents occur one time in 1,000, but that when the warehouse is negligent, accidents occur 90% of the time. The materials also indicated that the defendant is negligent only 1% of the time. Judges overestimated the probability that the defendant was negligent, failing to consider the base rate of negligence. Interestingly, this logical fallacy is implemented in the doctrine (res ipsa loquitur), which instructs judges to take no account of the base rates ( Kaye, 1979 ).
3. ^ Note that other CB, such as framing and omission bias, might also shed light on judicial decision-making ( Rachlinski, 2018 ). In fact, judges decide cases differently depending on whether the underlying facts are presented as gains or losses ( Rachlinski and Wistrich, 2018 ). Moreover, viewing the acceptance of a claim as the path of action and its dismissal as the path of inaction, omission bias might explain why the acceptance threshold of judges of a plaintiff’s claim is particularly high ( Zamir and Ritov, 2012 ). However, those biases have been much less studied than anchoring and hindsight bias.
Baker, K. H., and Nofsinger, J. R. (2002). Psychological biases of investors. Financ. Ser. Rev. 11, 97–116.
Google Scholar
Baker, H. K., and Nofsinger, J. R. (Eds.). (2010). Behavioral Finance: Investors, Corporations, and Markets. Vol. 6. New York: John Wiley & Sons.
Baker, H. K., and Ricciardi, V. (Eds.). (2014). Investor Behavior: The Psychology of Financial Planning and Investing. New York: John Wiley and Sons.
Barber, B. M., and Odean, T. (2000). Trading is hazardous to your wealth: The common stock investment performance of individual investors. J. Financ. 55, 773–806. doi: 10.1111/0022-1082.00226
CrossRef Full Text | Google Scholar
Barber, B., and Odean, T. (2001). Boys will be boys: gender, overconfidence, and common stock investment. Q. J. Econ. 116, 261–292. doi: 10.1162/003355301556400
Barnes, J. H. (1984). Cognitive biases and their impact on strategic planning. Strateg. Manag. J. 5, 129–137. doi: 10.1002/smj.4250050204
Baron, J. (2008). Thinking and Deciding. 4th Edn. Cambridge: Cambridge University Press.
Baron, J., and Hershey, J. C. (1988). Outcome bias in decision evaluation. J. Pers. Soc. Psychol. 54, 569–579. doi: 10.1037/0022-3514.54.4.569
Baron, J., and Ritov, I. (2004). Omission bias, individual differences, and normality. Organ. Behav. Hum. Decis. Process. 94, 74–85. doi: 10.1016/j.obhdp.2004.03.003
Bazerman, M. H. (1998). Judgment in Managerial Decision Making. New York: Wiley.
Bazerman, M. H., and Moore, D. (2008). Judgment in Managerial Decision Making. 7th Edn. Hoboken, NJ: Wiley.
Benartzi, S., and Thaler, R. H. (1995). Myopic loss aversion and the equity premium puzzle. Q. J. Econ. 110, 73–92. doi: 10.2307/2118511
Ben-David, I., Graham, J., and Harvey, C. (2013). Managerial miscalibration. Q. J. Econ. 128, 1547–1584. doi: 10.1093/qje/qjt023
Berlin, L., and Hendrix, R. W. (1998). Perceptual errors and negligence. AJR Am. J. Roentgenol. 170, 863–867. doi: 10.2214/ajr.170.4.9530024
PubMed Abstract | CrossRef Full Text | Google Scholar
Berthet, V. (2021). The measurement of individual differences in cognitive biases: A review and improvement. Front. Psychol. 12:630177. doi: 10.3389/fpsyg.2021.630177
Blumenthal-Barby, J. S., and Krieger, H. (2015). Cognitive biases and heuristics in medical decision making: A critical review using a systematic search strategy. Med. Decis. Mak. 35, 539–557. doi: 10.1177/0272989X14547740
Bogner, M. S. (Ed.) (1994). Human Error in Medicine. Hillsdale, NJ: Lawrence Erlbaum Associates, Inc.
Boolell-Gunesh, S., Broihanne, M., and Merli, M. (2009). Disposition effect, investor sophistication and taxes: Some French specificities. Finance 30, 51–78. doi: 10.3917/fina.301.0051
Brenner, L. A., Koehler, D. J., Liberman, V., and Tversky, A. (1996). Overconfidence in probability and frequency judgments: A critical examination. Organ. Behav. Hum. Decis. Process. 65, 212–219. doi: 10.1006/obhd.1996.0021
Bruine de Bruin, W., Parker, A. M., and Fischhoff, B. (2007). Individual differences in adult decision-making competence. J. Pers. Soc. Psychol. 92, 938–956. doi: 10.1037/0022-3514.92.5.938
Bukszar, E., and Connolly, T. (1988). Hindsight bias and strategic choice: Some problems in learning from experience. Acad. Manag. J. 31, 628–641.
Bystranowski, P., Janik, B., Próchnicki, M., and Skórska, P. (2021). Anchoring effect in legal decision-making: A meta-analysis. Law Hum. Behav. 45, 1–23. doi: 10.1037/lhb0000438
Casper, J. D., Benedict, K., and Perry, J. L. (1989). Juror decision making, attitudes, and the hindsight bias. Law Hum. Behav. 13, 291–310. doi: 10.1007/BF01067031
Chapman, G. B., and Bornstein, B. H. (1996). The more you ask for, the more you get: anchoring in personal injury verdicts. Appl. Cogn. Psychol. 10, 519–540. doi: 10.1002/(SICI)1099-0720(199612)10:6<519::AID-ACP417>3.0.CO;2-5
Cheney, F. W., Posner, K., Caplan, R. A., and Ward, R. J. (1989). Standard of care and anesthesia liability. JAMA 261, 1599–1603. doi: 10.1001/jama.1989.03420110075027
Chuang, W.-I., and Lee, B.-S. (2006). An empirical evaluation of the overconfidence hypothesis. J. Bank. Financ. 30, 2489–2515. doi: 10.1016/j.jbankfin.2005.08.007
Cohen, M. D., March, J. G., and Olsen, J. P. (1972). A garbage can model of organizational choice. Adm. Sci. Q. 17, 1–25. doi: 10.2307/2392088
Coval, J. D., and Moskowitz, T. J. (1999). Home bias at home: local equity preference in domestic portfolios. J. Financ. 54, 2045–2073. doi: 10.1111/0022-1082.00181
Croskerry, P. (2003). The importance of cognitive errors in diagnosis and strategies to minimize them. Acad. Med. 78, 775–780. doi: 10.1097/00001888-200308000-00003
Croskerry, P., Singhal, G., and Mamede, S. (2013). Cognitive debiasing 1: origins of bias and theory of debiasing. BMJ Qual. Saf. , 22(Suppl 2), 58–64. doi: 10.1136/bmjqs-2012-001712
Crowley, R. S., Legowski, E., Medvedeva, O., Reitmeyer, K., Tseytlin, E., Castine, M., et al. (2013). Automated detection of heuristics and biases among pathologists in a computer-based system. Adv. Health Sci. Educ. Theory Pract. 18, 343–363. doi: 10.1007/s10459-012-9374-z
Danziger, S., Levav, J., and Avnaim-Pesso, L. (2011). Extraneous factors in judicial decisions. Proc. Natl. Acad. Sci. 108, 6889–6892. doi: 10.1073/pnas.1018033108
Das, T. K., and Teng, B. (1999). Cognitive biases and strategic decision processes: An integrative perspective. J. Manag. Stud. 36, 757–778. doi: 10.1111/1467-6486.00157
Dawson, N. V., and Arkes, H. R. (1987). Systematic errors in medical decision making. J. Gen. Intern. Med. 2, 183–187. doi: 10.1007/BF02596149
De Bondt, W. F. (1998). A portrait of the individual investor. Eur. Econ. Rev. 42, 831–844. doi: 10.1016/S0014-2921(98)00009-9
De Bondt, W. F. M., and Thaler, R. (1985). Does the stock market overreact? J. Financ. 40, 793–805. doi: 10.1111/j.1540-6261.1985.tb05004.x
De Long, J. B., Shleifer, A., Summers, L. H., and Waldmann, R. J. (1990). Noise Trader Risk in Financial Markets. J. Polit. Econ. 98, 703–738.
PubMed Abstract | Google Scholar
Detmer, D. E., Fryback, D. G., and Gassner, K. (1978). Heuristics and biases in medical decision-making. J. Med. Educ. 53, 682–683.
Dhami, M. K., and Belton, I. K. (2017). On getting inside the judge’s mind. Trans. Issues Psychol. Sci. 3, 214–226. doi: 10.1037/tps0000115
Diamond, S. S. (1997). Illuminations and shadows from jury simulations. Law Hum. Behav. 21, 561–571. doi: 10.1023/A:1024831908377
Diamond, S. S., Saks, M. J., and Landsman, S. (1998). Jurors judgments about liability and damages: sources of variability and ways to increase consistency. DePaul Law Rev. 48, 301–325.
Duhaime, I. M., and Schwenk, C. R. (1985). Conjectures on cognitive simplification in acquisition and divestment decision making. Acad. Manag. Rev. 10, 287–295. doi: 10.5465/amr.1985.4278207
Ebbesen, E. B., and Konecni, V. J. (1975). Decision making and information integration in the courts: The setting of bail. J. Pers. Soc. Psychol. 32, 805–821. doi: 10.1037/0022-3514.32.5.805
Eisenberg, T., Goerdt, J., Ostrom, B., Rottman, D., and Wells, M. T. (1997). The predictability of punitive damages. J. Leg. Stud. 26, 623–661. doi: 10.1086/468010
Eisenberg, T., Hannaford-Agor, P. L., Heise, M., LaFountain, N., Munsterman, G. T., Ostrom, B., et al. (2006). Juries, judges, and punitive damages: empirical analyses using the civil justice survey of state courts 1992, 1996, and 2001 data. J. Empir. Leg. Stud. 3, 263–295. doi: 10.1111/j.1740-1461.2006.00070.x
Eisenhardt, K. M., and Zbaracki, M. J. (1992). Strategic decision making. Strateg. Manag. J. 13, 17–37. doi: 10.1002/smj.4250130904
Elstein, A. S. (1999). Heuristics and biases: selected errors in clinical reasoning. Acad. Med. 74, 791–794. doi: 10.1097/00001888-199907000-00012
Englich, B., Mussweiler, T., and Strack, F. (2005). The last word in court--A hidden disadvantage for the defense. Law Hum. Behav. 29, 705–722. doi: 10.1007/s10979-005-8380-7
Englich, B., Mussweiler, T., and Strack, F. (2006). Playing dice with criminal sentences: the influence of irrelevant anchors on experts’ judicial decision making. Personal. Soc. Psychol. Bull. 32, 188–200. doi: 10.1177/0146167205282152
Enough, B., and Mussweiler, T. (2001). Sentencing Under uncertainty: anchoring effects in the courtroom. J. Appl. Soc. Psychol. 31, 1535–1551. doi: 10.1111/j.1559-1816.2001.tb02687.x
Findley, K. A., and Scott, M. S. (2006). The multiple dimensions of tunnel vision in criminal cases. Wis. Law Rev. 2, 291–398.
Fischhoff, B. (1975). Hindsight is not equal to foresight: The effect of outcome knowledge on judgment under uncertainty. J. Exp. Psychol. Hum. Percept. Perform. 1, 288–299.
Forrow, L., Taylor, W. C., and Arnold, R. M. (1992). Absolutely relative: how research results are summarized can affect treatment decisions. Am. J. Med. 92, 121–124. doi: 10.1016/0002-9343(92)90100-P
Gigerenzer, G. (1991). “How to make cognitive illusions disappear: Beyond “heuristics and biases,”” in European Review of Social Psychology. Vol. 2 W. Stroebe and M. Hewstone (Eds.) (Chichester: Wiley), 83–115.
Gigerenzer, G. (1996). On narrow norms and vague heuristics: A reply to Kahneman and Tversky. Psychol. Rev. 103, 592–596. doi: 10.1037/0033-295X.103.3.592
Gigerenzer, G., Hertwig, R., Hoffrage, U., and Sedlmeier, P. (2008). “Cognitive illusions reconsidered,” in Handbook of Experimental Economics Results. eds. C. R. Plott and V. L. Smith (Amsterdam: Elsevier), 1018–1034. doi: 10.1016/S1574-0722(07)00109-6
Gilovich, T., Griffin, D., and Kahneman, D. (Eds.) (2002). Heuristics and Biases: The Psychology of Intuitive Judgment. Cambridge: Cambridge University Press.
Glaser, M., and Weber, M. (2007). Overconfidence and trading volume. Geneva Risk Insur. Rev. 32, 1–36. doi: 10.1007/s10713-007-0003-3
Graber, M. L. (2013). The incidence of diagnostic error in medicine. BMJ Qual. Saf. , 22(Suppl 2), 21–27. doi: 10.1136/bmjqs-2012-001615
Graber, M. L., Franklin, N., and Gordon, R. (2005). Diagnostic error in internal medicine. Arch. Intern. Med. 165, 1493–1499. doi: 10.1001/archinte.165.13.1493
Graber, M., Gordon, R., and Franklin, N. (2002). Reducing diagnostic errors in medicine: what’s the goal? Acad. Med. 77, 981–992. doi: 10.1097/00001888-200210000-00009
Graber, M. L., Kissam, S., Payne, V. L., Meyer, A. N., Sorensen, A., Lenfestey, N., et al. (2012). Cognitive interventions to reduce diagnostic error: a narrative review. BMJ Qual. Saf. 21, 535–557. doi: 10.1136/bmjqs-2011-000149
Grinblatt, M., Titman, S., and Wermers, R. (1995). Momentum investment strategies, portfolio performance, and herding: A study of mutual fund behavior. Am. Econ. Rev. 85, 1088–1105.
Guthrie, C., Rachlinski, J. J., and Wistrich, A. J. (2001). Inside the judicial mind. Cornell Law Rev. 86, 777–830. doi: 10.2139/ssrn.257634
Guthrie, C., Rachlinski, J. J., and Wistrich, A. J. (2002). Judging by heuristic: cognitive illusions in judicial decision making. Judicature 86, 44–50.
Guthrie, C., Rachlinski, J., and Wistrich, A. J. (2007). Blinking on the bench: how judges decide cases. Cornell Law Rev. 93, 1–43.
Hans, V. P., and Reyna, V. F. (2011). To dollars from sense: qualitative to quantitative translation in jury damage awards. J. Empir. Leg. Stud. 8, 120–147. doi: 10.1111/j.1740-1461.2011.01233.x
Hardman, D., and Harries, C. (2002). How rational are we? Psychologist 15, 76–79.
Harley, E. M. (2007). Hindsight bias in legal decision making. Soc. Cogn. 25, 48–63. doi: 10.1521/soco.2007.25.1.48
Hart, A. J., Evans, D. L., Wissler, R. L., Feehan, J. W., and Saks, M. J. (1997). Injuries, prior beliefs, and damage awards. Behav. Sci. Law 15, 63–82. doi: 10.1002/(SICI)1099-0798(199724)15:1<63::AID-BSL254>3.0.CO;2-9
Hastie, R., Schkade, D. A., and Payne, J. W. (1999). Juror judgments in civil cases: effects of plaintiff’s requests and plaintiff’s identity on punitive damage awards. Law Hum. Behav. 23, 445–470. doi: 10.1023/A:1022312115561
Helm, R. K., Wistrich, A. J., and Rachlinski, J. J. (2016). Are arbitrators human? J. Empir. Leg. Stud. 13, 666–692. doi: 10.1111/jels.12129
Hershberger, P. J., Part, H. M., Markert, R. J., Cohen, S. M., and Finger, W. W. (1994). Development of a test of cognitive bias in medical decision making. Acad. Med. 69, 839–842. doi: 10.1097/00001888-199410000-00014
Hinsz, V. B., and Indahl, K. E. (1995). Assimilation to anchors for damage awards in a mock civil trial. J. Appl. Soc. Psychol. 25, 991–1026. doi: 10.1111/j.1559-1816.1995.tb02386.x
Hodgkinson, G. (2001). “Cognitive processes in strategic management: some emerging trends and future directions,” in Handbook of Industrial, Work and Organizational Psychology Organizational Psychology. Vol. 2. eds. N. Anderson, D. S. Ones, and H. K. Sinangil (London: SAGE Publications Ltd.), 416–440.
Hodgkinson, G. P., Bown, N. J., Maule, A. J., Glaister, K. W., and Pearman, A. D. (1999). Breaking the frame: an analysis of strategic cognition and decision making under uncertainty. Strateg. Manag. J. 20, 977–985. doi: 10.1002/(SICI)1097-0266(199910)20:10<977::AID-SMJ58>3.0.CO;2-X
Huff, A. S., and Schwenk, C. (1990). “Bias and sensemaking in good times and bad,” in Mapping Strategic Thought. ed. A. S. Huff (Ed.) (Chichester, England: Wiley), 89–108.
Johnson, G. (1987). Strategic Change and the Management Process. Oxford: Basil Blackwell.
Joyce, E., and Biddle, G. (1981). Anchoring and adjustment in probabilistic inference in auditing. J. Account. Res. 19, 120–145. doi: 10.2307/2490965
Kahneman, D. (2011). Thinking, Fast and Slow. New York: Farrar, Straus and Giroux.
Kahneman, D., and Frederick, S. (2002). “Representativeness revisited: attribute substitution in intuitive judgment,” in Heuristics and Biases: The Psychology of Intuitive Judgment. T. Gilovich, D. Griffin, and D. Kahneman (Eds.) (Cambridge: Cambridge University Press), 103–119.
Kahneman, D., Schkade, D., and Sunstein, C. (1998). Shared outrage and erratic awards: The psychology of punitive damages. J. Risk Uncertain. 16, 49–86. doi: 10.1023/A:1007710408413
Kahneman, D., Slovic, P., and Tversky, A. (Eds.) (1982). Judgment Under Uncertainty: Heuristics and Biases. New York: Cambridge University Press.
Kahneman, D., and Tversky, A. (1979). Prospect theory: An analysis of decision under risk. Econometrica 47, 263–291. doi: 10.2307/1914185
Kamin, K. A., and Rachlinski, J. J. (1995). Ex post ≠ ex ante: determining liability in hindsight. Law Hum. Behav. 19, 89–104. doi: 10.1007/BF01499075
Kaustia, M., Alho, E., and Puttonen, V. (2008). How much does expertise reduce behavioral biases? The case of anchoring effects in stock return estimates. Financ. Manag. 37, 391–412. doi: 10.1111/j.1755-053X.2008.00018.x
Kaye, D. (1979). Probability theory meets res Ipsa loquitur. Mich. Law Rev. 77, 1456–1484. doi: 10.2307/1288109
Klein, J. G. (2005). Five pitfalls in decisions about diagnosis and prescribing. BMJ 330, 781–783. doi: 10.1136/bmj.330.7494.781
Klein, D. E., and Mitchell, G. (Eds.) (2010). The Psychology of Judicial Decision Making. New York, NY: Oxford University Press.
Kukucka, J., Kassin, S. M., Zapf, P. A., and Dror, I. E. (2017). Cognitive bias and blindness: A global survey of forensic science examiners. J. Appl. Res. Mem. Cogn. 6, 452–459. doi: 10.1016/j.jarmac.2017.09.001
Kumar, S., and Goyal, N. (2015). Behavioural biases in investment decision making – A systematic literature review. Qual. Res. Financ. Markets 7, 88–108. doi: 10.1108/QRFM-07-2014-0022
LaBine, S. J., and LaBine, G. (1996). Determinations of negligence and the hindsight bias. Law Hum. Behav. 20, 501–516. doi: 10.1007/BF01499038
Lidén, M., Gräns, M., and Juslin, P. (2019). ‘Guilty, no doubt’: detention provoking confirmation bias in judges’ guilt assessments and debiasing techniques. Psychol. Crime Law 25, 219–247. doi: 10.1080/1068316X.2018.1511790
Lusardi, A., and Mitchell, O. S. (2014). The economic importance of financial literacy: theory and evidence. J. Econ. Lit. 52, 5–44.
Lyles, M. A., and Thomas, H. (1988). Strategic problem formulation: biases and assumptions embedded in alternative decision-making models. J. Manag. Stud. 25, 131–145. doi: 10.1111/j.1467-6486.1988.tb00028.x
MacCoun, R. J. (1989). Experimental research on jury decision-making. Science 244, 1046–1050. doi: 10.1126/science.244.4908.1046
Malmendier, U., and Tate, G. (2005). CEO overconfidence and corporate investment. J. Financ. 60, 2661–2700. doi: 10.1111/j.1540-6261.2005.00813.x
Malmendier, U., and Tate, G. (2008). Who makes acquisitions? CEO overconfidence and the market’s reaction. J. Financ. Econ. 89, 20–43. doi: 10.1016/j.jfineco.2007.07.002
Mamede, S., van Gog, T., van den Berge, K., Rikers, R. M., van Saase, J. L., van Guldener, C., et al. (2010). Effect of availability bias and reflective reasoning on diagnostic accuracy among internal medicine residents. JAMA 304, 1198–1203. doi: 10.1001/jama.2010.1276
March, J. G., and Shapira, Z. (1987). Managerial perspectives on risk and risk taking. Manag. Sci. 33, 1404–1418. doi: 10.1287/mnsc.33.11.1404
March, J. G., and Simon, H. A. (1958). Organizations. New York: Wiley.
Maule, A. J., and Hodgkinson, G. P. (2002). Heuristics, biases and strategic decision making. Psychologist 15, 68–71.
Mintzberg, H. (1983). Power In and Around Organizations. Englewood Cliffs, N.J: Prentice-Hall.
Mohammed, S., and Schwall, A. (2012). Individual differences and decision making: what we know and where we go from here. Int. Rev. Ind. Organ. Psychol. 24, 249–312. doi: 10.1002/9780470745267.ch8
Moore, D. A., Oesch, J. M., and Zietsma, C. (2007). What competition? Myopic self-focus in market-entry decisions. Organ. Sci. 18, 440–454. doi: 10.1287/orsc.1060.0243
Moore, D. A., and Schatz, D. (2017). The three faces of overconfidence. Soc. Personal. Psychol. Compass 11:e122331. doi: 10.1111/spc3.12331
Morewedge, C. K., Yoon, H., Scopelliti, I., Symborski, C., Korris, J., and Kassam, K. S. (2015). Debiasing decisions: improved decision making with a single training intervention. Policy Insights Behav. Brain Sci. 2, 129–140. doi: 10.1177/2372732215600886
Nickerson, R. S. (1998). Confirmation bias: A ubiquitous phenomenon in many guises. Rev. Gen. Psychol. 2, 175–220. doi: 10.1037/1089-2680.2.2.175
O’Brien, B. (2009). Prime suspect: An examination of factors that aggravate and counteract confirmation bias in criminal investigations. Psychol. Public Policy Law 15, 315–334. doi: 10.1037/a0017881
Odean, T. (1998). Are investors reluctant to realize their losses? J. Financ. 53, 1775–1798. doi: 10.1111/0022-1082.00072
Odean, T. (1999). Do Investors trade too much? Am. Econ. Rev. 89, 1279–1298. doi: 10.1257/aer.89.5.1279
Oeberst, A., and Goeckenjan, I. (2016). When being wise after the event results in injustice: evidence for hindsight bias in judges’ negligence assessments. Psychol. Public Policy Law 22, 271–279. doi: 10.1037/law0000091
Ogdie, A. R., Reilly, J. B., Pang, W. G., Keddem, S., Barg, F. K., Von Feldt, J. M., et al. (2012). Seen through their eyes: residents’ reflections on the cognitive and contextual components of diagnostic errors in medicine. Acad. Med. 87, 1361–1367. doi: 10.1097/ACM.0b013e31826742c9
Parker, A. M., and Fischhoff, B. (2005). Decision-making competence: external validation through an individual-differences approach. J. Behav. Decis. Mak. 18, 1–27. doi: 10.1002/bdm.481
Peer, E., and Gamliel, E. (2013). Heuristics and biases in judicial decisions. Court Rev. 49, 114–118.
Perneger, T. V., and Agoritsas, T. (2011). Doctors and patients’ susceptibility to framing bias: A randomized trial. J. Gen. Intern. Med. 26, 1411–1417. doi: 10.1007/s11606-011-1810-x
Pohl, R. F. (2017). “Cognitive illusions,” in Cognitive Illusions: Intriguing Phenomena in Thinking, Judgment and Memory (London; New York, NY: Routledge/Taylor&Francis Group), 3–21.
Powell, T. C., Lovallo, D., and Fox, C. (2011). Behavioral strategy. Strateg. Manag. J. 32, 1369–1386. doi: 10.1002/smj.968
Rachlinski, J. J. (2006). Cognitive errors, individual differences, and paternalism. Univ. Chicago Law Rev. 73, 207–229. doi: 10.1093/acprof:oso/9780199211395.003.0008
Rachlinski, J. J. (2018). “Judicial decision-making,” in Behavioral Law and Economics. E. Zamir and D. Teichman (Eds.) (New York, NY: Oxford University Press), 525–565.
Rachlinski, J. J., Guthrie, C., and Wistrich, A. J. (2011). Probable cause, probability, and hindsight. J. Empir. Leg. Stud. 8, 72–98. doi: 10.1111/j.1740-1461.2011.01230.x
Rachlinski, J. J., Guthrie, C., and Wistrich, A. J. (2013). How lawyers’ intuitions prolong litigation. South. Calif. Law Rev. 86, 571–636.
Rachlinski, J. J., and Wistrich, A. J. (2017). Judging the judiciary by the numbers: empirical research on judges. Ann. Rev. Law Soc. Sci. 13, 203–229. doi: 10.1146/annurev-lawsocsci-110615-085032
Rachlinski, J. J., and Wistrich, A. J. (2018). Gains, losses, and judges: framing and the judiciary. Notre Dame Law Rev. 94, 521–582.
Rachlinski, J., Wistrich, A., and Guthrie, C. (2015). Can judges make reliable numeric judgments? Distorted damages and skewed sentences. Indiana Law J. 90, 695–739.
Redelmeier, D. A. (2005). The cognitive psychology of missed diagnoses. Ann. Intern. Med. 142, 115–120. doi: 10.7326/0003-4819-142-2-200501180-00010
Robbennolt, J. K., and Studebaker, C. A. (1999). Anchoring in the courtroom: The effects of caps on punitive damages. Law Hum. Behav. 23, 353–373. doi: 10.1023/A:1022312716354
Saposnik, G., Redelmeier, D., Ruff, C. C., and Tobler, P. N. (2016). Cognitive biases associated with medical decisions: a systematic review. BMC Med. Inform. Decis. Mak. 6:138. doi: 10.1186/s12911-016-0377-1
Schmitt, B. P., and Elstein, A. S. (1988). Patient management problems: heuristics and biases. Med. Decs. Making 8, 224–225.
Schnapp, B. H., Sun, J. E., Kim, J. L., Strayer, R. J., and Shah, K. H. (2018). Cognitive error in an academic emergency department. Diagnosis 5, 135–142. doi: 10.1515/dx-2018-0011
Schwenk, C. R. (1982). Dialectical inquiry in strategic decision-making: A comment on the continuing debate. Strateg. Manag. J. 3, 371–373. doi: 10.1002/smj.4250030408
Schwenk, C. R. (1984). Cognitive simplification processes in strategic decision-making. Strateg. Manag. J. 5, 111–128. doi: 10.1002/smj.4250050203
Schwenk, C. R. (1985). Management illusions and biases: their impact on strategic decisions. Long Range Plan. 18, 74–80. doi: 10.1016/0024-6301(85)90204-3
Schwenk, C. R. (1988). The cognitive perspective on strategic decision making. J. Manag. Stud. 25, 41–55. doi: 10.1111/j.1467-6486.1988.tb00021.x
Sellier, A. L., Scopelliti, I., and Morewedge, C. K. (2019). Debiasing training improves decision making in the field. Psychol. Sci. 30, 1371–1379. doi: 10.1177/0956797619861429
Shefrin, H. (2000). Beyond Greed and Fear: Understanding Behavioral Finance and the Psychology of Investing. Boston: Harvard Business School Press.
Shefrin, H., and Statman, M. (1985). The disposition to sell winners too early and ride losers too long: theory and evidence. J. Financ. 40, 777–790. doi: 10.1111/j.1540-6261.1985.tb05002.x
Shiller, R. J. (2003). From efficient markets theory to behavioral finance. J. Econ. Perspect. 17, 83–104. doi: 10.1257/089533003321164967
Stanovich, K. E., Toplak, M. E., and West, R. F. (2008). The development of rational thought: a taxonomy of heuristics and biases. Adv. Child Dev. Behav. 36, 251–285. doi: 10.1016/S0065-2407(08)00006-2
Stanovich, K. E., West, R. F., and Toplak, M. E. (2011). “Individual differences as essential components of heuristics and biases research,” in The Science of Reason: A Festschrift for Jonathan St B. T. Evans. K. Manktelow, D. Over, and S. Elqayam (Eds.) (New York: Psychology Press), 355–396.
Statman, M., Thorley, S., and Vorkink, K. (2006). Investor overconfidence and trading volume. Rev. Financ. Stud. 19, 1531–1565. doi: 10.1093/rfs/hhj032
Stiegler, M. P., and Ruskin, K. J. (2012). Decision-making and safety in anesthesiology. Curr. Opin. Anaesthesiol. 25, 724–729. doi: 10.1097/ACO.0b013e328359307a
Talpsepp, T. (2011). Reverse disposition effect of foreign investors. J. Behav. Financ. 12, 183–200. doi: 10.1080/15427560.2011.606387
Tversky, A., and Kahneman, D. (1973). Availability: A heuristic for judging frequency and probability. Cogn. Psychol. 5, 207–232. doi: 10.1016/0010-0285(73)90033-9
Tversky, A., and Kahneman, D. (1974). Judgment under uncertainty: heuristics and biases. Science 185, 1124–1131. doi: 10.1126/science.185.4157.1124
Tversky, A., and Kahneman, D. (1981). The framing of decisions and the psychology of choice. Science 211, 453–458. doi: 10.1126/science.7455683
Vranas, P. B. M. (2000). Gigerenzer’s normative critique of Kahneman and Tversky. Cognition 76, 179–193. doi: 10.1016/S0010-0277(99)00084-0
Wears, R. L., and Nemeth, C. P. (2007). Replacing hindsight with insight: toward better understanding of diagnostic failures. Ann. Emerg. Med. 49, 206–209. doi: 10.1016/j.annemergmed.2006.08.027
Weinshall-Margel, K., and Shapard, J. (2011). Overlooked factors in the analysis of parole decisions. Proc. Natl. Acad. Sci. 108:E833. doi: 10.1073/pnas.1110910108
Wissler, R. L., Hart, A. J., and Saks, M. J. (1999). Decision-making about general damages: A comparison of jurors, judges, and lawyers. Mich. Law Rev. 98, 751–826. doi: 10.2307/1290315
Zajac, E. J., and Bazerman, M. H. (1991). Blind spots in industry and competitor analysis: implications of interfirm (mis)perceptions for strategic decisions. Acad. Manag. Rev. 16, 37–56. doi: 10.5465/amr.1991.4278990
Zamir, E., and Ritov, I. (2012). Loss aversion, omission bias, and the burden of proof in civil litigation. J. Leg. Stud. 41, 165–207. doi: 10.1086/664911
Zwaan, L., Monteiro, S., Sherbino, J., Ilgen, J., Howey, B., and Norman, G. (2017). Is bias in the eye of the beholder? A vignette study to assess recognition of cognitive biases in clinical case workups. BMJ Qual. Saf. 26, 104–110. doi: 10.1136/bmjqs-2015-005014
Keywords: decision-making, cognitive biases, heuristics, management, finance, medicine, law
Citation: Berthet V (2022) The Impact of Cognitive Biases on Professionals’ Decision-Making: A Review of Four Occupational Areas. Front. Psychol . 12:802439. doi: 10.3389/fpsyg.2021.802439
Received: 26 October 2021; Accepted: 03 December 2021; Published: 04 January 2022.
Reviewed by:
Copyright © 2022 Berthet. This is an open-access article distributed under the terms of the Creative Commons Attribution License (CC BY) . The use, distribution or reproduction in other forums is permitted, provided the original author(s) and the copyright owner(s) are credited and that the original publication in this journal is cited, in accordance with accepted academic practice. No use, distribution or reproduction is permitted which does not comply with these terms.
*Correspondence: Vincent Berthet, [email protected]
Disclaimer: All claims expressed in this article are solely those of the authors and do not necessarily represent those of their affiliated organizations, or those of the publisher, the editors and the reviewers. Any product that may be evaluated in this article or claim that may be made by its manufacturer is not guaranteed or endorsed by the publisher.
Thank you for visiting nature.com. You are using a browser version with limited support for CSS. To obtain the best experience, we recommend you use a more up to date browser (or turn off compatibility mode in Internet Explorer). In the meantime, to ensure continued support, we are displaying the site without styles and JavaScript.
- View all journals
Decision making articles from across Nature Portfolio
Latest research and reviews.
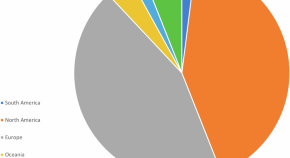
The rise of parent led climate movement from care to climate action
- Romina Rodela
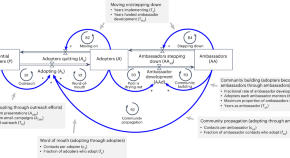
Community-based propagation to scale up educational innovations in sustainability
Innovative educational methods can be difficult to spread across universities and instructors. This study examines the best routes to rapidly scale new methods in sustainability education.
- Juliette N. Rooney-Varga
- Florian Kapmeier
- David N. Ford
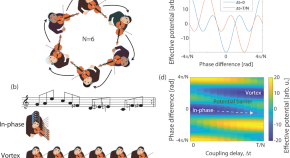
How synchronized human networks escape local minima
Human networks can demonstrate more rich dynamics and different mechanisms of self-organization compared to other types of networks. Considering an example of violin player ensemble, the authors show that by self-tuning tempo, amplitude, and coupling strength between players, their network achieves stability and synchronization.
- Elad Shniderman
- Yahav Avraham
- Moti Fridman

Prosocial preferences can escalate intergroup conflicts by countering selfish motivations to leave
When individuals meet hostile groups, they can choose whether to defend themselves or flee and leave others behind. Here, the authors show that pro-social preferences predict staying and defense, while leaving is predicted by concerns for personal costs and risk.
- Luuk L. Snijder
- Carsten K. W. De Dreu
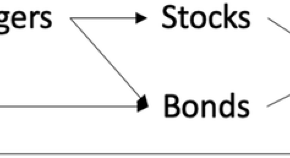
The evolving climate change investing strategies of asset owners
- Emil Moldovan
- Anthony Leiserowitz
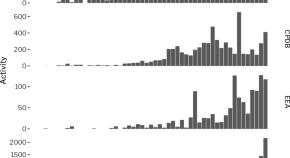
A review of national climate policies via existing databases
- Yves Steinebach
- Markus Hinterleitner
- Xavier Fernández-i-Marín
News and Comment
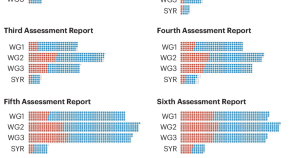
Diversity in IPCC author’s composition does not equate to inclusion
The IPCC holds the gold standard for climate change scientific knowledge and authority at the science–policy interface. Here we reflect on our experience of the IPCC Sixth Assessment Report and discuss how diversity in authorship and inclusion of different disciplinary backgrounds can be improved.
- Martina Angela Caretta
- Shobha Maharaj
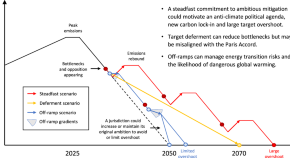
Temporary mitigation off-ramps could help manage decarbonization headwinds
Compressing global energy and industrial system decarbonization into less than three decades creates unique social, technical, financial and political risks. Here we introduce ‘off-ramps’ as one potential approach to manage these whilst still driving rapid emissions reductions.
- Chris Greig
Brazil’s coastline under attack
- Marcus V. Cianciaruso
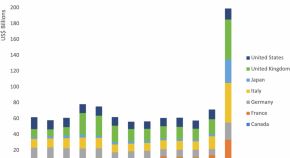
Revitalizing international fossil fuel subsidy phase-out commitments through roadmaps, closing loopholes, and support
Fossil fuel subsidies remain a persistent problem notwithstanding multiple international commitments to phase them out. A new approach is needed to ensure commitments account for, and help overcome, domestic barriers to fossil fuel subsidy reform. This new approach should comprise time-bound roadmaps, steps to close existing loopholes, and support for lower-income countries.
- Jakob Skovgaard
- Harro van Asselt
- Peter Wooders
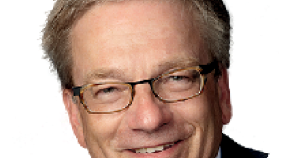
Never too local for science advice
No government is too small to promote data-informed decisions and innovation. This is especially true for cities, argues Rémi Quirion.
- Rémi Quirion
Reframing rational judgement
- Omid Ghasemi
Quick links
- Explore articles by subject
- Guide to authors
- Editorial policies

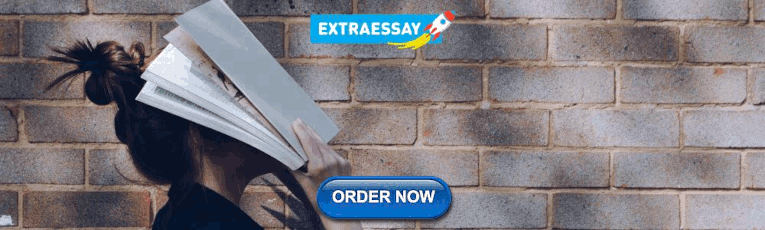
IMAGES
VIDEO
COMMENTS
This article defines the basis for decision-making: In research from Schoemaker & Russo [9], decisionmaking is processed by which individuals, groups, or organisations make decisions about future ...
Decision-making competence refers to the ability to make better decisions, as defined by decision-making principles posited by models of rational choice. Historically, psychological research on decision-making has examined how well people follow these principles under carefully manipulated experimental conditions.
A review of the literature regarding research over the past 35 years on the relationship between emotions and decision making is contained in the article by Lerner at al. [29]. The research reveals that emotions constitute potent, pervasive, predictable, sometimes harmful and sometimes beneficial drivers of decision making.
Early decision research emphasized the role of cognitive processes in decision-making (e.g., Newell & Simon 1972). But more recent work shows that emotions—not just strong emotions like anger and fear, but also “faint whispers of emotions” known as affect ( Slovic et al. 2004 , p. 312)—play an important role in decision-making.
Decision-making is a crucial skill that has a central role in everyday life and is necessary for adaptation to the environment and autonomy. It is the ability to choose between two or more options, and it has been studied through several theoretical approaches and by different disciplines. In this overview article, we contend a theoretical review regarding most theorizing and research on ...
This limit was found in the four areas covered. Individual differences have been neglected in decision-making research in general (Stanovich et al., 2011; Mohammed and Schwall, 2012). Indeed, most of the current knowledge about the impact of CB on decision-making relies upon experimental research and group comparisons (Gilovich et al., 2002 ...
JBDM seeks proposals of high-quality research that reflect contributions from world-wide sources. Special Issue proposals should be high-quality, psychological research on decision making. The journal encourages real-life contexts of decision making, and where possible, discusses the broader implications of the work.
As the US heads toward a presidential election, political polarization is influencing personal relationships, living choices, and even corporate decision-making. Research by Elisabeth Kempf reveals how partisan divides are shaping businesses, with significant implications for investment returns, credit ratings, and economic growth. 17 Sep 2024.
reviewed the results of four strategies that had been proposed as solutions for biased. decision making: (1) offering warnings about the possibility of bias; (2) describing the. direction of a bias; (3) providing a dose of feedback; and (4) offering an extended. program of training with feedback, coaching, and other interventions designed to ...
Compressing global energy and industrial system decarbonization into less than three decades creates unique social, technical, financial and political risks. Here we introduce ‘off-ramps’ as ...