Deep-Learning-Specialization
Coursera deep learning specialization, neural networks and deep learning.
In this course, you will learn the foundations of deep learning. When you finish this class, you will:
- Understand the major technology trends driving Deep Learning.
- Be able to build, train and apply fully connected deep neural networks.
- Know how to implement efficient (vectorized) neural networks.
- Understand the key parameters in a neural network’s architecture.
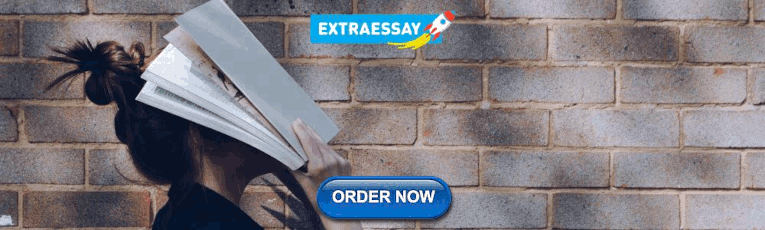
Week 1: Introduction to deep learning
Be able to explain the major trends driving the rise of deep learning, and understand where and how it is applied today.
- Quiz 1: Introduction to deep learning
Week 2: Neural Networks Basics
Learn to set up a machine learning problem with a neural network mindset. Learn to use vectorization to speed up your models.
- Quiz 2: Neural Network Basics
- Programming Assignment: Python Basics With Numpy
- Programming Assignment: Logistic Regression with a Neural Network mindset
Week 3: Shallow neural networks
Learn to build a neural network with one hidden layer, using forward propagation and backpropagation.
- Quiz 3: Shallow Neural Networks
- Programming Assignment: Planar Data Classification with Onehidden Layer
Week 4: Deep Neural Networks
Understand the key computations underlying deep learning, use them to build and train deep neural networks, and apply it to computer vision.
- Quiz 4: Key concepts on Deep Neural Networks
- Programming Assignment: Building your Deep Neural Network Step by Step
- Programming Assignment: Deep Neural Network Application
Course Certificate
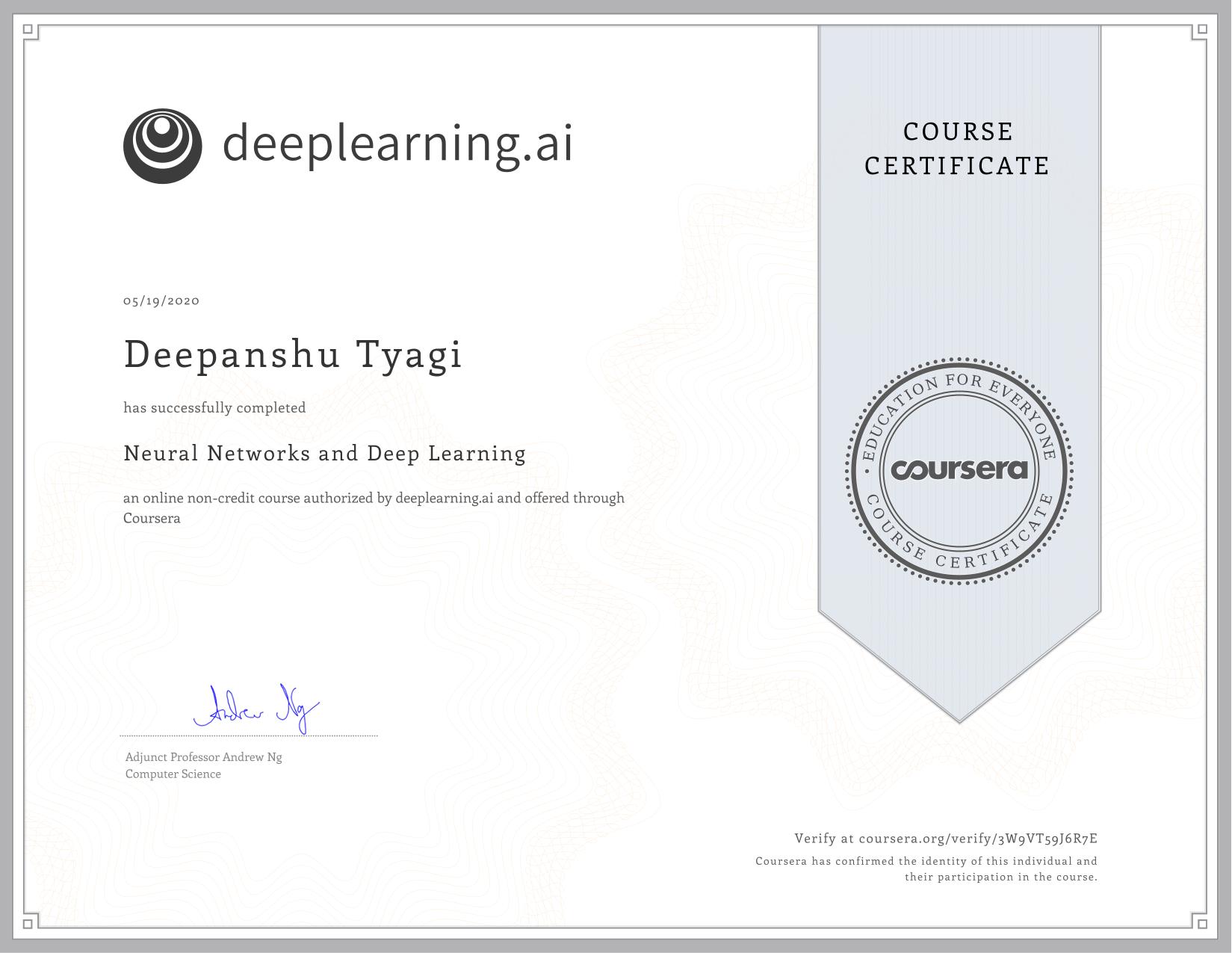
- 🌐 All Sites
- _APDaga DumpBox
- _APDaga Tech
- _APDaga Invest
- _APDaga Videos
- 🗃️ Categories
- _Free Tutorials
- __Python (A to Z)
- __Internet of Things
- __Coursera (ML/DL)
- __HackerRank (SQL)
- __Interview Q&A
- _Artificial Intelligence
- __Machine Learning
- __Deep Learning
- _Internet of Things
- __Raspberry Pi
- __Coursera MCQs
- __Linkedin MCQs
- __Celonis MCQs
- _Handwriting Analysis
- __Graphology
- _Investment Ideas
- _Open Diary
- _Troubleshoots
- _Freescale/NXP
- 📣 Mega Menu
- _Logo Maker
- _Youtube Tumbnail Downloader
- 🕸️ Sitemap
Coursera: Neural Networks and Deep Learning (Week 4A) [Assignment Solution] - deeplearning.ai
▸ building your deep neural network: step by step. i have recently completed the neural networks and deep learning course from coursera by deeplearning.ai while doing the course we have to go through various quiz and assignments in python. here, i am sharing my solutions for the weekly assignments throughout the course. these solutions are for reference only. > it is recommended that you should solve the assignments by yourself honestly then only it makes sense to complete the course. > but, in case you stuck in between, feel free to refer to the solutions provided by me., don't just copy paste the code for the sake of completion. even if you copy the code, make sure you understand the code first. click here : coursera: neural networks & deep learning (week 3) click here: coursera: neural networks & deep learning (week 4b) scroll down for coursera: neural networks & deep learning (week 4a) assignments . (adsbygoogle = window.adsbygoogle || []).push({}); recommended machine learning courses: coursera: machine learning coursera: deep learning specialization coursera: machine learning with python coursera: advanced machine learning specialization udemy: machine learning linkedin: machine learning eduonix: machine learning edx: machine learning fast.ai: introduction to machine learning for coders, building your deep neural network: step by step, in this notebook, you will implement all the functions required to build a deep neural network. in the next assignment, you will use these functions to build a deep neural network for image classification. after this assignment you will be able to: use non-linear units like relu to improve your model build a deeper neural network (with more than 1 hidden layer) implement an easy-to-use neural network class notation : superscript [ l ] denotes a quantity associated with the l t h layer. example: a [ l ] is the l t h layer activation. w [ l ] and b [ l ] are the l t h layer parameters. superscript ( i ) denotes a quantity associated with the i t h example. example: x ( i ) is the i t h training example. lowerscript i i denotes the i t h entry of a vector. example: a [ l ] i denotes the i t h entry of the l t h layer's activations). let's get started, 1 - packages, let's first import all the packages that you will need during this assignment. numpy is the main package for scientific computing with python. matplotlib is a library to plot graphs in python. dnn_utils provides some necessary functions for this notebook. testcases provides some test cases to assess the correctness of your functions np.random.seed(1) is used to keep all the random function calls consistent. it will help us grade your work. please don't change the seed., 2 - outline of the assignment.
- Initialize the parameters for a two-layer network and for an L -layer neural network.
- Complete the LINEAR part of a layer's forward propagation step (resulting in Z [ l ] ).
- We give you the ACTIVATION function (relu/sigmoid).
- Combine the previous two steps into a new [LINEAR->ACTIVATION] forward function.
- Stack the [LINEAR->RELU] forward function L-1 time (for layers 1 through L-1) and add a [LINEAR->SIGMOID] at the end (for the final layer L ). This gives you a new L_model_forward function.
- Compute the loss.
- Complete the LINEAR part of a layer's backward propagation step.
- We give you the gradient of the ACTIVATE function (relu_backward/sigmoid_backward)
- Combine the previous two steps into a new [LINEAR->ACTIVATION] backward function.
- Stack [LINEAR->RELU] backward L-1 times and add [LINEAR->SIGMOID] backward in a new L_model_backward function
- Finally update the parameters.

Check-out our free tutorials on IOT (Internet of Things):
3 - Initialization
3.1 - 2-layer neural network.
- The model's structure is: LINEAR -> RELU -> LINEAR -> SIGMOID .
- Use random initialization for the weight matrices. Use np.random.randn(shape)*0.01 with the correct shape.
- Use zero initialization for the biases. Use np.zeros(shape) .
3.2 - L-layer Neural Network

- The model's structure is [LINEAR -> RELU] × × (L-1) -> LINEAR -> SIGMOID . I.e., it has L − 1 L − 1 layers using a ReLU activation function followed by an output layer with a sigmoid activation function.
- Use random initialization for the weight matrices. Use np.random.randn(shape) * 0.01 .
- Use zeros initialization for the biases. Use np.zeros(shape) .
- We will store n [ l ] n [ l ] , the number of units in different layers, in a variable layer_dims . For example, the layer_dims for the "Planar Data classification model" from last week would have been [2,4,1]: There were two inputs, one hidden layer with 4 hidden units, and an output layer with 1 output unit. Thus means W1 's shape was (4,2), b1 was (4,1), W2 was (1,4) and b2 was (1,1). Now you will generalize this to L L layers!
- Here is the implementation for L = 1 L = 1 (one layer neural network). It should inspire you to implement the general case (L-layer neural network).
4 - Forward propagation module
4.1 - linear forward.
- LINEAR -> ACTIVATION where ACTIVATION will be either ReLU or Sigmoid.
- [LINEAR -> RELU] × × (L-1) -> LINEAR -> SIGMOID (whole model)
[[ 3.26295337 -1.23429987]] |
4.2 - Linear-Activation Forward
- Sigmoid : σ ( Z ) = σ ( W A + b ) = 1 1 + e − ( W A + b ) . We have provided you with the sigmoid function. This function returns two items: the activation value " a " and a " cache " that contains " Z " (it's what we will feed in to the corresponding backward function). To use it you could just call: A , activation_cache = sigmoid ( Z )
- ReLU : The mathematical formula for ReLu is A = R E L U ( Z ) = m a x ( 0 , Z ) A = R E L U ( Z ) = m a x ( 0 , Z ) . We have provided you with the relu function. This function returns two items: the activation value " A " and a " cache " that contains " Z " (it's what we will feed in to the corresponding backward function). To use it you could just call: A , activation_cache = relu ( Z )
[[ 0.96890023 0.11013289]] | |
[[ 3.43896131 0. ]] |
d) L-Layer Model

- Use the functions you had previously written
- Use a for loop to replicate [LINEAR->RELU] (L-1) times
- Don't forget to keep track of the caches in the "caches" list. To add a new value c to a list , you can use list.append(c) .
[[ 0.03921668 0.70498921 0.19734387 0.04728177]] | |
3 |
5 - Cost function
0.41493159961539694 |
6 - Backward propagation module
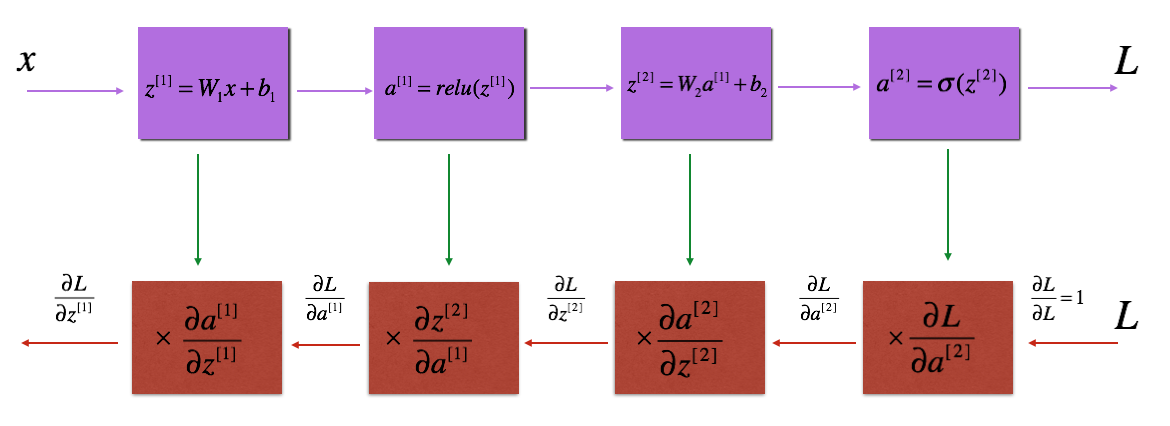
- LINEAR backward
- LINEAR -> ACTIVATION backward where ACTIVATION computes the derivative of either the ReLU or sigmoid activation
- [LINEAR -> RELU] × × (L-1) -> LINEAR -> SIGMOID backward (whole model)
6.1 - Linear backward
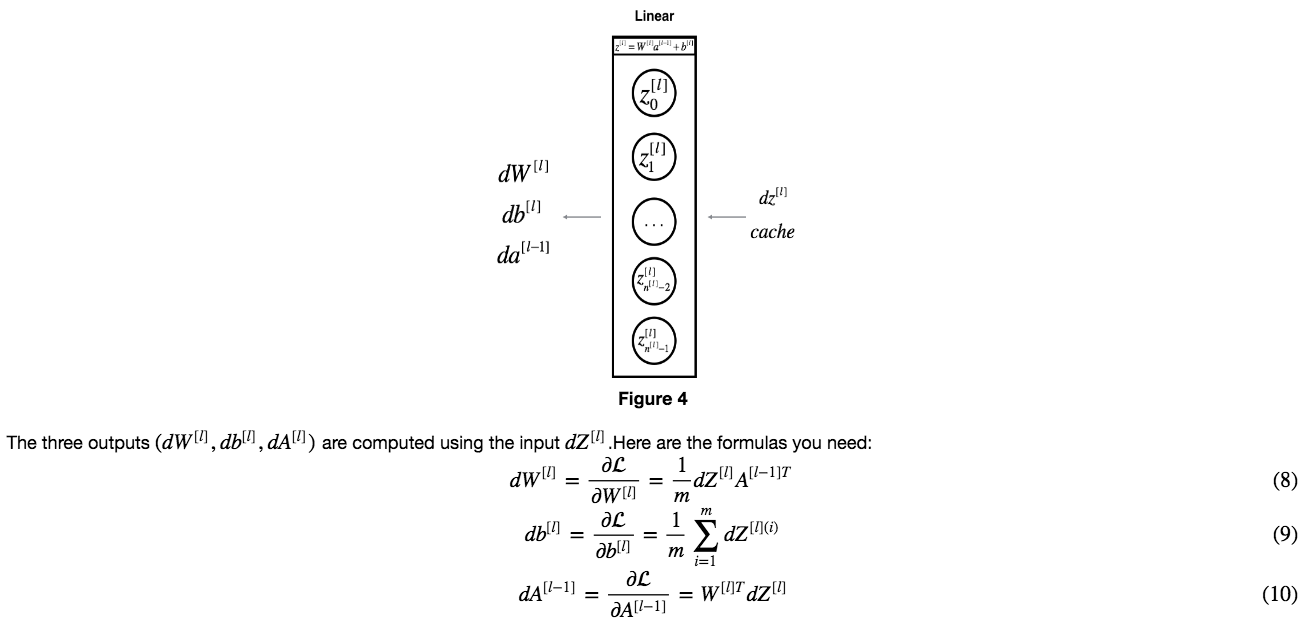
6.2 - Linear-Activation backward
- sigmoid_backward : Implements the backward propagation for SIGMOID unit. You can call it as follows:
- relu_backward : Implements the backward propagation for RELU unit. You can call it as follows:
6.3 - L-Model Backward

dW1 | [[ 0.41010002 0.07807203 0.13798444 0.10502167] [ 0. 0. 0. 0. ] [ 0.05283652 0.01005865 0.01777766 0.0135308 ]] |
db1 | [[-0.22007063] [ 0. ] [-0.02835349]] |
dA1 | [[ 0.12913162 -0.44014127] [-0.14175655 0.48317296] [ 0.01663708 -0.05670698]] |
6.4 - Update Parameters
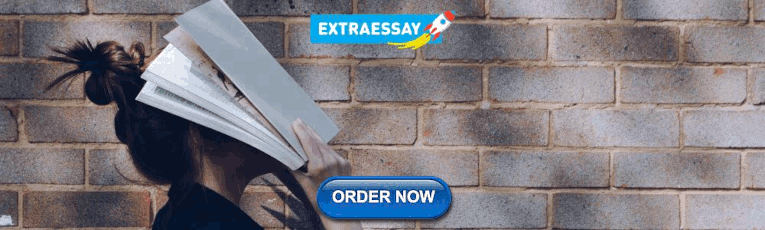
7 - Conclusion
- A two-layer neural network
- An L-layer neural network

hi bro...i was working on the week 4 assignment .i am getting an assertion error on cost_compute function.help me with this..but the same function is working for the l layer model AssertionError Traceback (most recent call last) in () ----> 1 parameters = two_layer_model(train_x, train_y, layers_dims = (n_x, n_h, n_y), num_iterations = 2500, print_cost= True) in two_layer_model(X, Y, layers_dims, learning_rate, num_iterations, print_cost) 46 # Compute cost 47 ### START CODE HERE ### (≈ 1 line of code) ---> 48 cost = compute_cost(A2, Y) 49 ### END CODE HERE ### 50 /home/jovyan/work/Week 4/Deep Neural Network Application: Image Classification/dnn_app_utils_v3.py in compute_cost(AL, Y) 265 266 cost = np.squeeze(cost) # To make sure your cost's shape is what we expect (e.g. this turns [[17]] into 17). --> 267 assert(cost.shape == ()) 268 269 return cost AssertionError:
Hey,I am facing problem in linear activation forward function of week 4 assignment Building Deep Neural Network. I think I have implemented it correctly and the output matches with the expected one. I also cross check it with your solution and both were same. But the grader marks it, and all the functions in which this function is called as incorrect. I am unable to find any error in its coding as it was straightforward in which I used built in functions of SIGMOID and RELU. Please guide.
hi bro iam always getting the grading error although iam getting the crrt o/p for all
Our website uses cookies to improve your experience. Learn more
Contact form
Get the Reddit app
A subreddit dedicated to learning machine learning
Where can I get the assignment solutions for Coursera: Neural Networks and Deep Learning Course by deeplearning.ai?
I think Coursera is the best place to start learning “Machine Learning” by Andrew NG (Stanford University) followed by Neural Networks and Deep Learning by same tutor. This course is full of theory required with practical assignments in MATLAB & Python. It recommended to solve the assignments honestly by yourself for full understanding.
I have done the same. In case you stuck in between, You can refer my solutions just for understanding. Don’t just copy paste it.
(These solution might be helpful for you to understand the deep learning in better way…)
I have recently completed that and these are the solutions for the Coursera: Neural Networks and Deep learning course by Home - deeplearning.ai Assignment Solutions in Python.
I have tried to provide optimized solutions :
Logistic Regression with a Neural Network mindset: Coursera: Neural Networks and Deep Learning (Week 2) [Assignment Solution] - deeplearning.ai
Planar data classification with one hidden layer: Coursera: Neural Networks and Deep Learning (Week 3) [Assignment Solution] - deeplearning.ai
Building your Deep Neural Network: Step by Step: Coursera: Neural Networks and Deep Learning (Week 4A) [Assignment Solution] - deeplearning.ai
Deep Neural Network for Image Classification: Application: Coursera: Neural Networks and Deep Learning (Week 4B) [Assignment Solution] - deeplearning.ai
Thanks, - Akshay P Daga
By continuing, you agree to our User Agreement and acknowledge that you understand the Privacy Policy .
Enter the 6-digit code from your authenticator app
You’ve set up two-factor authentication for this account.
Enter a 6-digit backup code
Create your username and password.
Reddit is anonymous, so your username is what you’ll go by here. Choose wisely—because once you get a name, you can’t change it.
Reset your password
Enter your email address or username and we’ll send you a link to reset your password
Check your inbox
An email with a link to reset your password was sent to the email address associated with your account
Choose a Reddit account to continue
Navigation Menu
Search code, repositories, users, issues, pull requests..., provide feedback.
We read every piece of feedback, and take your input very seriously.
Saved searches
Use saved searches to filter your results more quickly.
To see all available qualifiers, see our documentation .
- Notifications You must be signed in to change notification settings
Solutions to all quiz and all the programming assignments!!!
HeroKillerEver/coursera-deep-learning
Folders and files.
Name | Name | |||
---|---|---|---|---|
27 Commits | ||||
Repository files navigation

A series of online courses offered by deeplearning.ai . I would like to say thanks to Prof. Andrew Ng and his colleagues for spreading knowledge to normal people and great courses sincerely.
The reason I would like to create this repository is purely for academic use (in case for my future use). I am really glad if you can use it as a reference and happy to discuss with you about issues related with the course even for further deep learning techniques.
Please only use it as a reference . The quiz and assignments are relatively easy to answer, hope you can have fun with the courses.
1. Neural Network and Deep Learning
- Logistic Regression as a Neural Network
- Building your Deep Neural Network - Step by Step
- Deep Neural Network Application-Image Classification
2. Improving Deep Neural Networks-Hyperparameter tuning, Regularization and Optimization
- Initialization
- Regularization
- Gradient Checking
- Optimization
3. Structuring Machine Learning Projects
4. convolutional neural network.
- Convolutional Model- step by step
- Car detection for Autonomous Driving
- Neural Style Transfer
- Face Recognition
5. Sequence Models
- Building a Recurrent Neural Network - Step by Step
- Dinosaur Island -- Character-level language model
- Jazz improvisation with LSTM
- Word Vector Representation
- Machine Translation
- Trigger Word Detection
Haibin Yu/ @HeroKillerEver
Contributors 6
- Jupyter Notebook 98.9%
- Python 1.1%
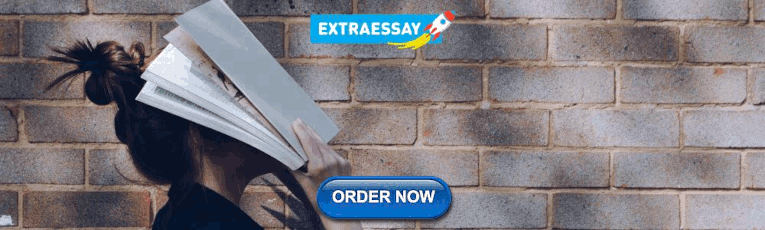
IMAGES
VIDEO
COMMENTS
amanchadha/coursera-deep-learning-specialization ...
Solutions of Deep Learning Specialization by Andrew Ng on Coursera - muhac/coursera-deep-learning-solutions. ... Programming Assignments Course A Course B Course C Course D Course E; Practice Questions: Course A: Course B: Course C: Course D: Course E: Programming Assignments. Course A - Neural Networks and Deep Learning. Week 2 - Neural ...
A repository of code and notes for the Deep Learning Specialization 2023 by Andrew Ng on Coursera. Includes quizzes, assignments, projects, and certificates for five courses on neural networks, optimization, convolutional networks, sequence models, and transformers.
This GitHub repository contains the course materials for the Coursera Deep Learning Specialization, which covers the foundations of deep learning. You can find the course syllabus, quizzes, programming assignments, and course certificate here.
I have recently completed the Neural Networks and Deep Learning course from Coursera by deeplearning.ai. While doing the course we have to go through various quiz and assignments in Python. Here, I am sharing my solutions for the weekly assignments throughout the course. These solutions are for reference only.
Links for the Solutions are here: 👇🏻Coursera: Neural Networks and Deep Learning Assignment Solution for reference - Andrew NG | deeplearning.ai | APDaga | ...
Learn how to build a deep neural network with Python and numpy. This notebook provides solutions for the week 4A assignment of the course, including initialization, forward and backward propagation, and update parameters.
Andrew Ng's Main Reasons Deep Learning is Taking Off. In this order: 1)Bigger data sets, 2) Can compute larger models, 3) Algorithmic advancements. Andrew Ng's Indirect Benefits of Speed Improvemnts. Shorter developer iterations for testing out ideas. Binary Classification.
Learn the foundations of deep learning and neural networks with this course, part of the Deep Learning Specialization on Coursera. You will study the concepts, trends, applications, and techniques of deep learning, and earn a career certificate.
This repo contains the updated version of all the assignments/labs of Deep Learning Specialization on Coursera by Andrew Ng. It includes building various deep learning models from scratch and implementing them for object detection, facial recognition, autonomous driving, neural machine translation, etc.
If you want to break into cutting-edge AI, this course will help you do so. Deep learning engineers are highly sought after, and mastering deep learning will...
In the first course of the Deep Learning Specialization, you will study the foundational concept of neural networks and deep learning. By the end, you will be familiar with the significant technological trends driving the rise of deep learning; build, train, and apply fully connected deep neural networks; implement efficient (vectorized) neural networks; identify key parameters in a neural ...
Learn the fundamentals of deep learning and neural networks with Keras, a high-level Python library. This course is part of the IBM AI Engineering Professional Certificate and covers topics such as gradient descent, backpropagation, convolutional networks, and autoencoders.
Week 1 Quiz - Introduction to deep learning.md
I think Coursera is the best place to start learning "Machine Learning" by Andrew NG (Stanford University) followed by Neural Networks and Deep Learning by same tutor. This course is full of theory required with practical assignments in MATLAB & Python. It recommended to solve the assignments honestly by yourself for full understanding.
Learn how to develop deep learning models using PyTorch, a Python library for automatic differentiation and tensor computation. This course is part of the IBM AI Engineering Professional Certificate and covers topics such as linear regression, logistic regression, feedforward networks, convolutional networks, and more.
This specialisation has five courses. Courses: Course 1: Neural Networks and Deep Learning. Learning Objectives : Understand the major technology trends driving Deep Learning. Be able to build, train and apply fully connected deep neural networks. Know how to implement efficient (vectorized) neural networks.
Learn how to build and apply convolutional neural networks (CNNs) to various computer vision tasks, such as image classification, object detection, segmentation, and style transfer. This course is part of the Deep Learning Specialization and covers the foundations, case studies, and applications of CNNs.
HeroKillerEver/coursera-deep-learning
Learn the basics of deep learning, including neural networks, optimization methods, and applications. This course is part of a specialization that covers theory and hands-on practice with Python.