A large language model from Google Research, designed for the medical domain.
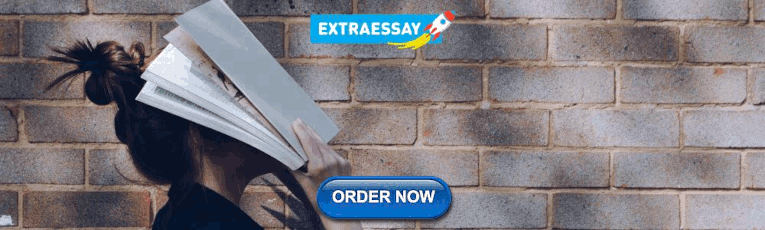
Introduction
Med-PaLM is a large language model (LLM) designed to provide high quality answers to medical questions. Our second version, Med-PaLM 2, is one of the research models that powers MedLM – a family of foundation models fine-tuned for the healthcare industry. MedLM is now available to Google Cloud customers who have been exploring a range of applications, from basic tasks to complex workflows.
Med-PaLM harnesses the power of Google’s large language models, which we have aligned to the medical domain and evaluated using medical exams, medical research, and consumer queries. Our first version of Med-PaLM, preprinted in late 2022 and published in Nature in July 2023 , was the first AI system to surpass the pass mark (>60%) in the U.S. Medical Licensing Examination (USMLE) style questions. Med-PaLM also generates accurate, helpful long-form answers to consumer health questions, as judged by panels of physicians and users.
We introduced Med-PaLM 2 at Google Health’s annual event, The Check Up, in March 2023. Med-PaLM 2 was the first to reach human expert level on answering USMLE-style questions. According to physicians, the model's long-form answers to consumer medical questions improved substantially.
Med-PaLM 2 reached 86.5% accuracy on the MedQA medical exam benchmark in research
Since medicine is inherently multimodal, we have also introduced research on a multimodal version of Med-PaLM, called Med-PaLM M . We are also exploring a wide range of other techniques to build medical AI systems that can bring information together from a wide range of data modalities.
Medical question–answering: a grand challenge for AI
Progress in AI over the last decade has enabled it to play an increasingly important role in healthcare and medicine . Breakthroughs such as the Transformer have enabled LLMs – such as PaLM – and other large models to scale to billions of parametersletting generative AI move beyond the limited pattern-spotting of earlier AIs and into the creation of novel expressions of content, from speech to scientific modeling.
Developing AI that can answer medical questions accurately has been a long-standing challenge with several research advances over the past few decades. While the topic is broad, answering USMLE-style questions has recently emerged as a popular benchmark for evaluating medical question answering performance.
Above is an example USMLE-style question. You are presented with a vignette containing a description of the patient, symptoms, and medications.
Answering the question accurately requires the reader to understand symptoms, examine findings from a patient’s tests, perform complex reasoning about the likely diagnosis, and ultimately, pick the right answer for what disease, test, or treatment is most appropriate. In short, a combination of medical comprehension, knowledge retrieval, and reasoning is necessary to do well. It takes years of training for clinicians to be able to accurately and consistently answer these questions.
The generation capabilities of large language models also enable them to produce long-form answers to consumer medical questions. However, ensuring model responses are accurate, safe, and helpful has been a crucial research challenge, especially in this safety-critical domain.
In a pairwise study, Med-PaLM 2 answers were preferred to physician answers across eight of nine axes considered.
Evaluating answer quality
We assessed Med-PaLM and Med-PaLM 2 against a benchmark we call ‘MultiMedQA’, which combines seven question answering datasets spanning professional medical exams, medical research, and consumer queries. Med-PaLM was the first AI system to obtain a passing score on USMLE-style questions from the MedQA dataset, with an accuracy of 67.6%. Med-PaLM 2 improves on this further with state of the art performance of 86.5%.
Importantly, in this work we go beyond multiple-choice accuracy to measure and improve model capabilities in medical question answering. Our model’s long-form answers were tested against several criteria — including scientific factuality, precision, medical consensus, reasoning, bias, and likelihood of possible harm — which were evaluated by clinicians and non-clinicians from a range of backgrounds and countries. Both Med-PaLM and Med-PaLM 2 performed encouragingly across three datasets of consumer medical questions. In a pairwise study, Med-PaLM 2 answers were preferred to physician answers across eight of nine axes considered.
Check out how Med-PaLM 2 answers medical questions
*Examples only. Med-PaLM 2 is currently being evaluated to ensure safe and responsible use.
Extending Med-PaLM 2 Beyond Language
The practice of medicine is inherently multi-modal and incorporates information from images, electronic health records, sensors, wearables, genomics and more. We believe AI systems that leverage these data at scale using self-supervised learning with careful consideration of privacy, safety and health equity will be the foundation of the next generation of medical AI systems that scale world-class healthcare to everyone.
Building on the “PaLM-E” vision-language model, we designed a multimodal version of Med-PaLM, called Med-PaLM M . This system can synthesize and communicate information from images like chest X-rays, mammograms, and more to help doctors provide better patient care. Within scope are several modalities alongside language: dermatology, retina, radiology (3D and 2D), pathology, health records and genomics. We’re excited to explore how this technology can benefit clinicians in the future.
*Example only. This image reflects early exploration of Med-PaLM M's future capabilities.
Limitations
While Med-PaLM 2 reached state-of-the-art performance on several multiple-choice medical question answering benchmarks, and our human evaluation shows answers compare favorably to physician answers across several clinically important axes, we know that more work needs to be done to ensure these models are safely and effectively deployed.
Careful consideration will need to be given to the ethical deployment of this technology including rigorous quality assessment in different clinical settings with guardrails to mitigate against risks. For example, the potential harms of using a LLM for diagnosing or treating an illness are much greater than using a LLM for information about a disease or medication. Additional research will be needed to assess LLMs used in healthcare for homogenization and amplification of biases and security vulnerabilities inherited from base models.
We dive into many important areas for further research in our Med-PaLM and Med-PaLM 2 papers.
In the press
Scientific American: AI Chatbots Can Diagnose Medical Conditions at Home. How Good Are They?
CNBC: Google’s working on an updated version of its medical A.I. that can answer health questions
Med Page Today: Google AI Performs at 'Expert' Level on U.S. Medical Licensing Exam
New Scientist: Google's AI is best yet at answering medical and health questions
The Economist: A bioethicist and a professor of medicine on regulating AI in healthcare
Advisory Board: Are AI doctors on the horizon?
STAT: Google will let health care customers test its generative AI model, ramping up rivalry with GPT-4
MobiHealthNews: Google to offer limited access to medical LLM
Forbes: How Tech Leaders Compete In The Battle Of Healthcare AI
Google Cloud Blog: MedLM
Acknowledgements
Med-PaLM research:
Karan Singhal*, Shekoofeh Azizi*, Tao Tu*, S. Sara Mahdavi, Jason Wei, Hyung Won Chung, Nathan Scales, Ajay Tanwani, Heather Cole-Lewis, Stephen Pfohl, Perry Payne, Martin Seneviratne, Paul Gamble, Chris Kelly, Abubakr Babiker, Yu-Han Liu, Nathanael Schärli, Aakanksha Chowdhery, Philip Mansfield, Dina Demner-Fushman, Blaise Agüera y Arcas, Dale Webster, Greg S. Corrado, Yossi Matias, Katherine Chou, Juraj Gottweis, Nenad Tomasev, Yun Liu, Alvin Rajkomar, Joelle Barral, Christopher Semturs, Alan Karthikesalingam**, and Vivek Natarajan**
Med-PaLM 2 research:
Karan Singhal*, Tao Tu*, Juraj Gottweis*, Rory Sayres*, Ellery Wulczyn, Le Hou, Kevin Clark, Stephen Pfohl, Heather Cole-Lewis, Darlene Neal, Mike Schaekermann, Amy Wang, Mohamed Amin, Sami Lachgar, Philip Mansfield, Sushant Prakash, Bradley Green, Ewa Dominowska, Blaise Aguera y Arcas, Nenad Tomasev, Yun Liu, Renee Wong, Christopher Semturs, S. Sara Mahdavi, Joelle Barral, Dale Webster, Greg S. Corrado, Yossi Matias, Shekoofeh Azizi**, Alan Karthikesalingam**, Vivek Natarajan**
Med-PaLM M research:
Tao Tu*, Shekoofeh Azizi*, Danny Driess, Mike Schaekermann, Mohamed Amin, Pi-Chuan Chang, Andrew Carroll, Chuck Lau, Ryutaro Tanno, Ira Ktena, Basil Mustafa, Aakanksha Chowdhery, Yun Liu, Simon Kornblith, David Fleet, Philip Mansfield, Sushant Prakash, Renee Wong, Sunny Virmani, Christopher Semturs, S Sara Mahdavi, Bradley Green, Ewa Dominowska, Blaise Aguera y Arcas, Joelle Barral, Dale Webster, Greg S. Corrado, Yossi Matias, Karan Singhal, Pete Florence, Alan Karthikesalingam** and Vivek Natarajan**
* - equal contributions
** - equal leadership
Additional contributors:
Preeti Singh, Kavita Kulkarni, Jonas Kemp, Anna Iurchenko, Lauren Winer, Will Vaughan, Le Hou, Jimmy Hu, Yuan Liu, Jonathan Krause, John Guilyard, Divya Pandya.
We thank Michael Howell, Boris Babenko, Naama Hammel, Cameron Chen, Basil Mustafa, David Fleet, Douglas Eck, Simon Kornblith, Fayruz Kibria, Gordon Turner, Lisa Lehmann, Ivor Horn, Maggie Shiels, Shravya Shetty, Jukka Zitting, Evan Rappaport, Lucy Marples, Viknesh Sounderajah, Ali Connell, Jan Freyberg, Dave Steiner, Cian Hughes, Brett Hatfield, SiWai Man, Gary Parakkal, Sudhanshu Sharma, Megan Jones-Bell, Susan Thomas, Martin Ho, Sushant Prakash, Bradley Green, Ewa Dominowska, Frederick Liu, Kate Weber, Annisah Um’rani, Laura Culp, and Xuezhi Wang for their assistance, insights, and feedback during our research.
We are also grateful to Yossi Matias, Karen DeSalvo, Zoubin Ghahramani, James Manyika, and Jeff Dean for their support throughout this project.
Discover More
A responsible path to generative AI in healthcare
Our latest health AI research updates
The next generation of AI for developers and Google Workspace
Google Research, 2022 & beyond: Health
Google Health + blog

An official website of the United States government
The .gov means it’s official. Federal government websites often end in .gov or .mil. Before sharing sensitive information, make sure you’re on a federal government site.
The site is secure. The https:// ensures that you are connecting to the official website and that any information you provide is encrypted and transmitted securely.
- Publications
- Account settings
- My Bibliography
- Collections
- Citation manager
Save citation to file
Email citation, add to collections.
- Create a new collection
- Add to an existing collection
Add to My Bibliography
Your saved search, create a file for external citation management software, your rss feed.
- Search in PubMed
- Search in NLM Catalog
- Add to Search
Medical literature searches: a comparison of PubMed and Google Scholar
Affiliation.
- 1 Department of Internal Medicine, Texas Tech University Health Sciences Center, Lubbock, TX, USA.
- PMID: 22925384
- DOI: 10.1111/j.1471-1842.2012.00992.x
Background: Medical literature searches provide critical information for clinicians. However, the best strategy for identifying relevant high-quality literature is unknown.
Objectives: We compared search results using PubMed and Google Scholar on four clinical questions and analysed these results with respect to article relevance and quality.
Methods: Abstracts from the first 20 citations for each search were classified into three relevance categories. We used the weighted kappa statistic to analyse reviewer agreement and nonparametric rank tests to compare the number of citations for each article and the corresponding journals' impact factors.
Results: Reviewers ranked 67.6% of PubMed articles and 80% of Google Scholar articles as at least possibly relevant (P = 0.116) with high agreement (all kappa P-values < 0.01). Google Scholar articles had a higher median number of citations (34 vs. 1.5, P < 0.0001) and came from higher impact factor journals (5.17 vs. 3.55, P = 0.036).
Conclusions: PubMed searches and Google Scholar searches often identify different articles. In this study, Google Scholar articles were more likely to be classified as relevant, had higher numbers of citations and were published in higher impact factor journals. The identification of frequently cited articles using Google Scholar for searches probably has value for initial literature searches.
© 2012 The authors. Health Information and Libraries Journal © 2012 Health Libraries Group.
PubMed Disclaimer
Similar articles
- Google Scholar versus PubMed in locating primary literature to answer drug-related questions. Freeman MK, Lauderdale SA, Kendrach MG, Woolley TW. Freeman MK, et al. Ann Pharmacother. 2009 Mar;43(3):478-84. doi: 10.1345/aph.1L223. Epub 2009 Mar 3. Ann Pharmacother. 2009. PMID: 19261965
- Comparison of PubMed and Google Scholar literature searches. Anders ME, Evans DP. Anders ME, et al. Respir Care. 2010 May;55(5):578-83. Respir Care. 2010. PMID: 20420728
- Variation in number of hits for complex searches in Google Scholar. Bramer WM. Bramer WM. J Med Libr Assoc. 2016 Apr;104(2):143-5. doi: 10.3163/1536-5050.104.2.009. J Med Libr Assoc. 2016. PMID: 27076802 Free PMC article.
- [Breast pathology: evaluation of the Portuguese scientific activity based on bibliometric indicators]. Donato HM, De Oliveira CF. Donato HM, et al. Acta Med Port. 2006 May-Jun;19(3):225-34. Epub 2006 Sep 7. Acta Med Port. 2006. PMID: 17234084 Review. Portuguese.
- Psychiatry and the Hirsch h-index: The relationship between journal impact factors and accrued citations. Hunt GE, Cleary M, Walter G. Hunt GE, et al. Harv Rev Psychiatry. 2010 Jul-Aug;18(4):207-19. doi: 10.3109/10673229.2010.493742. Harv Rev Psychiatry. 2010. PMID: 20597591 Review.
- Supporting the Frontlines: A Scoping Review Addressing the Health Challenges of Military Personnel and Veterans. Alruwaili A, Khorram-Manesh A, Ratnayake A, Robinson Y, Goniewicz K. Alruwaili A, et al. Healthcare (Basel). 2023 Oct 31;11(21):2870. doi: 10.3390/healthcare11212870. Healthcare (Basel). 2023. PMID: 37958012 Free PMC article. Review.
- Google Scholar as a Sole Literature Source for Meta-analyses in Otolaryngology Head and Neck Surgery. Ungar OJ, Muhanna N, Chaushu H. Ungar OJ, et al. Indian J Otolaryngol Head Neck Surg. 2023 Jun;75(2):864-870. doi: 10.1007/s12070-023-03532-8. Epub 2023 Feb 10. Indian J Otolaryngol Head Neck Surg. 2023. PMID: 37275023 Free PMC article.
- Impact of digital technologies on self-efficacy in people with Parkinson's: a scoping review protocol. Hall AM, Aroori S, Carroll CB, Meinert E, Allgar V. Hall AM, et al. BMJ Open. 2023 Mar 23;13(3):e069929. doi: 10.1136/bmjopen-2022-069929. BMJ Open. 2023. PMID: 36958772 Free PMC article.
- Palliative and End-of-Life Care Service Models: To What Extent Are Consumer Perspectives Considered? Rumbold B, Aoun SM. Rumbold B, et al. Healthcare (Basel). 2021 Sep 28;9(10):1286. doi: 10.3390/healthcare9101286. Healthcare (Basel). 2021. PMID: 34682966 Free PMC article. Review.
- The Impact of COVID-19 School Closure on Child and Adolescent Health: A Rapid Systematic Review. Chaabane S, Doraiswamy S, Chaabna K, Mamtani R, Cheema S. Chaabane S, et al. Children (Basel). 2021 May 19;8(5):415. doi: 10.3390/children8050415. Children (Basel). 2021. PMID: 34069468 Free PMC article. Review.
Publication types
- Search in MeSH
LinkOut - more resources
Full text sources.
- Ovid Technologies, Inc.
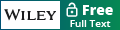
- Citation Manager
NCBI Literature Resources
MeSH PMC Bookshelf Disclaimer
The PubMed wordmark and PubMed logo are registered trademarks of the U.S. Department of Health and Human Services (HHS). Unauthorized use of these marks is strictly prohibited.
Skip to Content
AI-enabled imaging and diagnostics previously thought impossible
In partnership with healthcare organizations globally, we’re researching robust new AI-enabled tools focused on diagnostics to assist clinicians. Drawing from diverse datasets, high-quality labels, and state-of-the-art deep learning techniques, we are making models that we hope will eventually support medical specialists in diagnosing disease. We’re excited to further develop this research towards new frontiers—and to demonstrate that AI has the ability to enable novel, transformative diagnostics.
Improving access to skin disease information
This product has been CE marked as a Class I medical device in the EU. It is not available in the United States. This research is not related to the DermAssist tool, which is no longer in development.
Through computer vision AI and image search capabilities, we are developing a tool to help individuals better research & identify their skin, hair, and nail conditions. The tool supports hundreds of conditions, including more than 80% of the conditions seen in clinics and more than 90% of the most commonly searched conditions. The work was highlighted in both Nature Medicine and JAMA Network Open .
Using AI to help doctors address eye disease
Helping doctors prevent blindness.
Automated Retinal Disease Assessment (ARDA) is being used to help clinicians detect diabetic retinopathy, a leading cause of blindness, in India and across the world. With widespread adoption, perhaps millions of patients with diabetes could keep their vision in part to ARDA assisting doctors. Our research was published in JAMA and Ophthalmology . Additional research, published in Lancet Digital Health , showed that we can predict whether patients will develop diabetic retinopathy in the future, which can help doctors customize both treatment and eye screening frequencies for their patients. The solution is currently being evaluated in clinical studies in the United States as well as in Thailand. Learn more .
Using AI to improve lung cancer detection
A promising step forward for predicting lung cancer.
Lung cancer leads to over 1.8 million deaths per year world wide, accounting for almost one in five cancer deaths, and is the largest cause of cancer mortality. Our research, published in Nature Medicine , shows that deep learning may eventually help physicians more accurately screen for lung cancer and identify the disease even in incidental lung cancer detection workflows. Read the post
Novel biomarkers for non-eye related conditions
Anemia detection, computer vision, detecting hidden signs of anemia from the eye.
The human eye can reveal signs of underlying diseases like anemia, a condition that affects 1.6 billion people worldwide causing tiredness, weakness, dizziness and drowsiness. In research published in Nature Biomedical Engineering , we were able to use deep learning to quantify hemoglobin levels and detect anemia with de-identified photographs of the back of the eye. This result means it’s possible that someday providers may be able to detect the disease with a simple non-invasive screening tool. Read the post
Using computer vision to assess cardiovascular risk
Assessing the risk of cardiovascular diseases is the first and most critical step toward reducing the likelihood that a patient suffers a cardiovascular event in the future. By applying deep learning techniques to retinal images, we’ve been able to reveal factors associated with the risk of a major cardiovascular event like a heart attack or stroke, as published in Nature Biomedical Engineering . This research could help scientists generate more targeted hypotheses and drive a wide range of future research. Read the post
Using AI to improve breast cancer detection
Clinical practice, deep learning, studying how ai can help breast cancer screening in clinical practice.
Breast cancer screening helps detect cancer earlier, but diagnosing breast cancer accurately and consistently remains a challenge, with half of all women experiencing a false-positive over a 10-year period. In Nature , we demonstrated the potential of our AI model to analyze de-identified retrospectively collected screening mammograms with similar or better accuracy than clinicians. Now, we’re collaborating on an investigative device research study to understand how the model can help in clinical practice to reduce the time from screening mammography to diagnosis, narrowing the assessment gap and improving the patient experience. Read the post
Applying deep learning to metastatic breast cancer detection
In our pathology research published in the Archives of Pathology & Laboratory Medicine as well as The American Journal of Surgical Pathology , we showed how a proof-of-concept assistance tool (LYNA) could use deep learning to increase the accuracy of metastatic breast cancer detection. Read the post
Detecting signs of disease from external images of the eye
Exploring how external eye photos can reduce the need for specialized equipment.
We’re doing research and building AI models that can not only decipher important information from retinal images, but rather from external eye images. In our research published in The Lancet Digital Health , we show that a deep learning model can predict the presence of diabetic retinal disease and other biomarkers such as HbA1c or eGFR from external eye images alone. This could reduce the need for specialized equipment and expand access to care for the growing population of patients with diabetes or other chronic diseases. Read the post
Further research in imaging and diagnostics
We continue to advance AI-enabled imaging research in other domains, expanding this technology to facilitate transformative diagnostics.
AI Advances
Ai learning, ai diagnosis, exploring ai advancements in radiotherapy planning to improve efficiency.
Building off of work done with the University College London Hospitals and published in JMIR Publications , we are collaborating with Mayo Clinic to study the use of AI to help clinicians plan radiotherapy treatment for cancer. We’ve joined forces to research, train and validate an algorithm to assist physicians with segmenting healthy tissue and organs from tumors to reduce treatment planning time and improve the efficiency of radiotherapy, hopefully allowing clinicians to spend less time planning and more time with their patients. Read the post
Using machine learning to detect deficient coverage in colonoscopy screenings
Colorectal cancer (CRC) is a global health problem and the second deadliest cancer in the United States, resulting in an estimated 900K deaths per year. By alerting physicians to missed regions of the colon wall, our algorithm has the potential to lead to the discovery of more adenomas, thereby increasing the adenoma detection rate and decreasing the rate of interval colorectal cancer, as published in IEEE Transactions on Medical Imaging . Read the post
Using AI to identify the aggressiveness of prostate cancer
To diagnose the severity of prostate cancer, biopsies are analyzed and given a Gleason grade, which is scored on comparisons to healthy cells. In work published in JAMA Oncology and JAMA Network Open , we explored whether an AI system could accurately Gleason grade prostate biopsies, and our results indicated that the deep learning system has the potential to support expert-level diagnoses. Read the post
- Study Protocol
- Open access
- Published: 26 August 2024
Learning effect of online versus onsite education in health and medical scholarship – protocol for a cluster randomized trial
- Rie Raffing 1 ,
- Lars Konge 2 &
- Hanne Tønnesen 1
BMC Medical Education volume 24 , Article number: 927 ( 2024 ) Cite this article
Metrics details
The disruption of health and medical education by the COVID-19 pandemic made educators question the effect of online setting on students’ learning, motivation, self-efficacy and preference. In light of the health care staff shortage online scalable education seemed relevant. Reviews on the effect of online medical education called for high quality RCTs, which are increasingly relevant with rapid technological development and widespread adaption of online learning in universities. The objective of this trial is to compare standardized and feasible outcomes of an online and an onsite setting of a research course regarding the efficacy for PhD students within health and medical sciences: Primarily on learning of research methodology and secondly on preference, motivation, self-efficacy on short term and academic achievements on long term. Based on the authors experience with conducting courses during the pandemic, the hypothesis is that student preferred onsite setting is different to online setting.
Cluster randomized trial with two parallel groups. Two PhD research training courses at the University of Copenhagen are randomized to online (Zoom) or onsite (The Parker Institute, Denmark) setting. Enrolled students are invited to participate in the study. Primary outcome is short term learning. Secondary outcomes are short term preference, motivation, self-efficacy, and long-term academic achievements. Standardized, reproducible and feasible outcomes will be measured by tailor made multiple choice questionnaires, evaluation survey, frequently used Intrinsic Motivation Inventory, Single Item Self-Efficacy Question, and Google Scholar publication data. Sample size is calculated to 20 clusters and courses are randomized by a computer random number generator. Statistical analyses will be performed blinded by an external statistical expert.
Primary outcome and secondary significant outcomes will be compared and contrasted with relevant literature. Limitations include geographical setting; bias include lack of blinding and strengths are robust assessment methods in a well-established conceptual framework. Generalizability to PhD education in other disciplines is high. Results of this study will both have implications for students and educators involved in research training courses in health and medical education and for the patients who ultimately benefits from this training.
Trial registration
Retrospectively registered at ClinicalTrials.gov: NCT05736627. SPIRIT guidelines are followed.
Peer Review reports
Medical education was utterly disrupted for two years by the COVID-19 pandemic. In the midst of rearranging courses and adapting to online platforms we, with lecturers and course managers around the globe, wondered what the conversion to online setting did to students’ learning, motivation and self-efficacy [ 1 , 2 , 3 ]. What the long-term consequences would be [ 4 ] and if scalable online medical education should play a greater role in the future [ 5 ] seemed relevant and appealing questions in a time when health care professionals are in demand. Our experience of performing research training during the pandemic was that although PhD students were grateful for courses being available, they found it difficult to concentrate related to the long screen hours. We sensed that most students preferred an onsite setting and perceived online courses a temporary and inferior necessity. The question is if this impacted their learning?
Since the common use of the internet in medical education, systematic reviews have sought to answer if there is a difference in learning effect when taught online compared to onsite. Although authors conclude that online learning may be equivalent to onsite in effect, they agree that studies are heterogeneous and small [ 6 , 7 ], with low quality of the evidence [ 8 , 9 ]. They therefore call for more robust and adequately powered high-quality RCTs to confirm their findings and suggest that students’ preferences in online learning should be investigated [ 7 , 8 , 9 ].
This uncovers two knowledge gaps: I) High-quality RCTs on online versus onsite learning in health and medical education and II) Studies on students’ preferences in online learning.
Recently solid RCTs have been performed on the topic of web-based theoretical learning of research methods among health professionals [ 10 , 11 ]. However, these studies are on asynchronous courses among medical or master students with short term outcomes.
This uncovers three additional knowledge gaps: III) Studies on synchronous online learning IV) among PhD students of health and medical education V) with long term measurement of outcomes.
The rapid technological development including artificial intelligence (AI) and widespread adaption as well as application of online learning forced by the pandemic, has made online learning well-established. It represents high resolution live synchronic settings which is available on a variety of platforms with integrated AI and options for interaction with and among students, chat and break out rooms, and exterior digital tools for teachers [ 12 , 13 , 14 ]. Thus, investigating online learning today may be quite different than before the pandemic. On one hand, it could seem plausible that this technological development would make a difference in favour of online learning which could not be found in previous reviews of the evidence. On the other hand, the personal face-to-face interaction during onsite learning may still be more beneficial for the learning process and combined with our experience of students finding it difficult to concentrate when online during the pandemic we hypothesize that outcomes of the onsite setting are different from the online setting.
To support a robust study, we design it as a cluster randomized trial. Moreover, we use the well-established and widely used Kirkpatrick’s conceptual framework for evaluating learning as a lens to assess our outcomes [ 15 ]. Thus, to fill the above-mentioned knowledge gaps, the objective of this trial is to compare a synchronous online and an in-person onsite setting of a research course regarding the efficacy for PhD students within the health and medical sciences:
Primarily on theoretical learning of research methodology and
Secondly on
◦ Preference, motivation, self-efficacy on short term
◦ Academic achievements on long term
Trial design
This study protocol covers synchronous online and in-person onsite setting of research courses testing the efficacy for PhD students. It is a two parallel arms cluster randomized trial (Fig. 1 ).
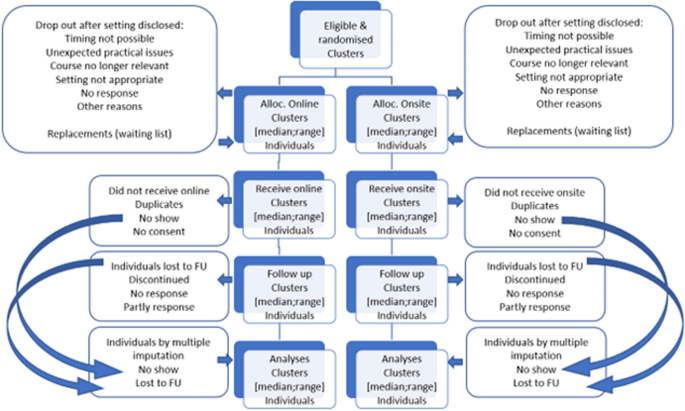
Consort flow diagram
The study measures baseline and post intervention. Baseline variables and knowledge scores are obtained at the first day of the course, post intervention measurement is obtained the last day of the course (short term) and monthly for 24 months (long term).
Randomization is stratified giving 1:1 allocation ratio of the courses. As the number of participants within each course might differ, the allocation ratio of participants in the study will not fully be equal and 1:1 balanced.
Study setting
The study site is The Parker Institute at Bispebjerg and Frederiksberg Hospital, University of Copenhagen, Denmark. From here the courses are organized and run online and onsite. The course programs and time schedules, the learning objective, the course management, the lecturers, and the delivery are identical in the two settings. The teachers use the same introductory presentations followed by training in break out groups, feed-back and discussions. For the online group, the setting is organized as meetings in the online collaboration tool Zoom® [ 16 ] using the basic available technicalities such as screen sharing, chat function for comments, and breakout rooms and other basics digital tools if preferred. The online version of the course is synchronous with live education and interaction. For the onsite group, the setting is the physical classroom at the learning facilities at the Parker Institute. Coffee and tea as well as simple sandwiches and bottles of water, which facilitate sociality, are available at the onsite setting. The participants in the online setting must get their food and drink by themselves, but online sociality is made possible by not closing down the online room during the breaks. The research methodology courses included in the study are “Practical Course in Systematic Review Technique in Clinical Research”, (see course programme in appendix 1) and “Getting started: Writing your first manuscript for publication” [ 17 ] (see course programme in appendix 2). The two courses both have 12 seats and last either three or three and a half days resulting in 2.2 and 2.6 ECTS credits, respectively. They are offered by the PhD School of the Faculty of Health and Medical Sciences, University of Copenhagen. Both courses are available and covered by the annual tuition fee for all PhD students enrolled at a Danish university.
Eligibility criteria
Inclusion criteria for participants: All PhD students enrolled on the PhD courses participate after informed consent: “Practical Course in Systematic Review Technique in Clinical Research” and “Getting started: Writing your first manuscript for publication” at the PhD School of the Faculty of Health and Medical Sciences, University of Copenhagen, Denmark.
Exclusion criteria for participants: Declining to participate and withdrawal of informed consent.
Informed consent
The PhD students at the PhD School at the Faculty of Health Sciences, University of Copenhagen participate after informed consent, taken by the daily project leader, allowing evaluation data from the course to be used after pseudo-anonymization in the project. They are informed in a welcome letter approximately three weeks prior to the course and again in the introduction the first course day. They register their consent on the first course day (Appendix 3). Declining to participate in the project does not influence their participation in the course.
Interventions
Online course settings will be compared to onsite course settings. We test if the onsite setting is different to online. Online learning is increasing but onsite learning is still the preferred educational setting in a medical context. In this case onsite learning represents “usual care”. The online course setting is meetings in Zoom using the technicalities available such as chat and breakout rooms. The onsite setting is the learning facilities, at the Parker Institute, Bispebjerg and Frederiksberg Hospital, The Capital Region, University of Copenhagen, Denmark.
The course settings are not expected to harm the participants, but should a request be made to discontinue the course or change setting this will be met, and the participant taken out of the study. Course participants are allowed to take part in relevant concomitant courses or other interventions during the trial.
Strategies to improve adherence to interventions
Course participants are motivated to complete the course irrespectively of the setting because it bears ECTS-points for their PhD education and adds to the mandatory number of ECTS-points. Thus, we expect adherence to be the same in both groups. However, we monitor their presence in the course and allocate time during class for testing the short-term outcomes ( motivation, self-efficacy, preference and learning). We encourage and, if necessary, repeatedly remind them to register with Google Scholar for our testing of the long-term outcome (academic achievement).
Outcomes are related to the Kirkpatrick model for evaluating learning (Fig. 2 ) which divides outcomes into four different levels; Reaction which includes for example motivation, self-efficacy and preferences, Learning which includes knowledge acquisition, Behaviour for practical application of skills when back at the job (not included in our outcomes), and Results for impact for end-users which includes for example academic achievements in the form of scientific articles [ 18 , 19 , 20 ].
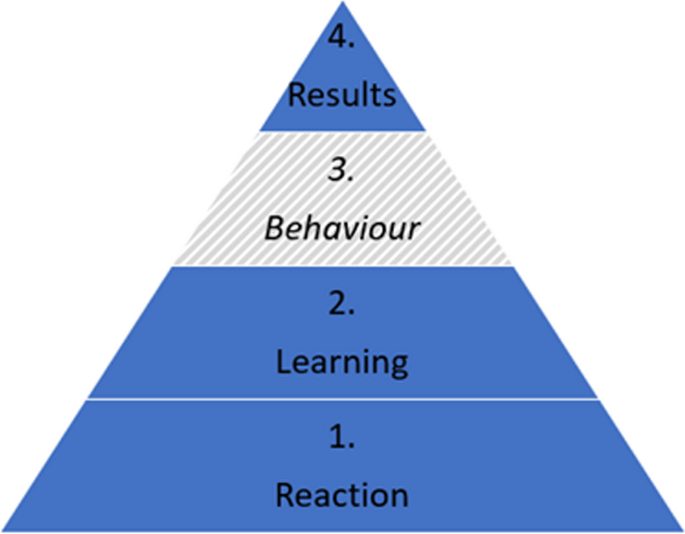
The Kirkpatrick model
Primary outcome
The primary outcome is short term learning (Kirkpatrick level 2).
Learning is assessed by a Multiple-Choice Questionnaire (MCQ) developed prior to the RCT specifically for this setting (Appendix 4). First the lecturers of the two courses were contacted and asked to provide five multiple choice questions presented as a stem with three answer options; one correct answer and two distractors. The questions should be related to core elements of their teaching under the heading of research training. The questions were set up to test the cognition of the students at the levels of "Knows" or "Knows how" according to Miller's Pyramid of Competence and not their behaviour [ 21 ]. Six of the course lecturers responded and out of this material all the questions which covered curriculum of both courses were selected. It was tested on 10 PhD students and within the lecturer group, revised after an item analysis and English language revised. The MCQ ended up containing 25 questions. The MCQ is filled in at baseline and repeated at the end of the course. The primary outcomes based on the MCQ is estimated as the score of learning calculated as number of correct answers out of 25 after the course. A decrease of points of the MCQ in the intervention groups denotes a deterioration of learning. In the MCQ the minimum score is 0 and 25 is maximum, where 19 indicates passing the course.
Furthermore, as secondary outcome, this outcome measurement will be categorized as binary outcome to determine passed/failed of the course defined by 75% (19/25) correct answers.
The learning score will be computed on group and individual level and compared regarding continued outcomes by the Mann–Whitney test comparing the learning score of the online and onsite groups. Regarding the binomial outcome of learning (passed/failed) data will be analysed by the Fisher’s exact test on an intention-to-treat basis between the online and onsite. The results will be presented as median and range and as mean and standard deviations, for possible future use in meta-analyses.
Secondary outcomes
Motivation assessment post course: Motivation level is measured by the Intrinsic Motivation Inventory (IMI) Scale [ 22 ] (Appendix 5). The IMI items were randomized by random.org on the 4th of August 2022. It contains 12 items to be assessed by the students on a 7-point Likert scale where 1 is “Not at all true”, 4 is “Somewhat true” and 7 is “Very true”. The motivation score will be computed on group and individual level and will then be tested by the Mann–Whitney of the online and onsite group.
Self-efficacy assessment post course: Self-efficacy level is measured by a single-item measure developed and validated by Williams and Smith [ 23 ] (Appendix 6). It is assessed by the students on a scale from 1–10 where 1 is “Strongly disagree” and 10 is “Strongly agree”. The self-efficacy score will be computed on group and individual level and tested by a Mann–Whitney test to compare the self-efficacy score of the online and onsite group.
Preference assessment post course: Preference is measured as part of the general course satisfaction evaluation with the question “If you had the option to choose, which form would you prefer this course to have?” with the options “onsite form” and “online form”.
Academic achievement assessment is based on 24 monthly measurements post course of number of publications, number of citations, h-index, i10-index. This data is collected through the Google Scholar Profiles [ 24 ] of the students as this database covers most scientific journals. Associations between onsite/online and long-term academic will be examined with Kaplan Meyer and log rank test with a significance level of 0.05.
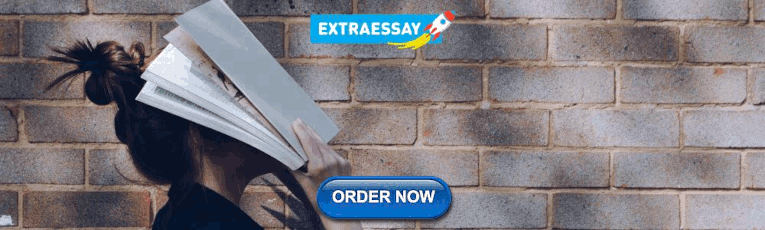
Participant timeline
Enrolment for the course at the Faculty of Health Sciences, University of Copenhagen, Denmark, becomes available when it is published in the course catalogue. In the course description the course location is “To be announced”. Approximately 3–4 weeks before the course begins, the participant list is finalized, and students receive a welcome letter containing course details, including their allocation to either the online or onsite setting. On the first day of the course, oral information is provided, and participants provide informed consent, baseline variables, and base line knowledge scores.
The last day of scheduled activities the following scores are collected, knowledge, motivation, self-efficacy, setting preference, and academic achievement. To track students' long term academic achievements, follow-ups are conducted monthly for a period of 24 months, with assessments occurring within one week of the last course day (Table 1 ).
Sample size
The power calculation is based on the main outcome, theoretical learning on short term. For the sample size determination, we considered 12 available seats for participants in each course. To achieve statistical power, we aimed for 8 clusters in both online and onsite arms (in total 16 clusters) to detect an increase in learning outcome of 20% (learning outcome increase of 5 points). We considered an intraclass correlation coefficient of 0.02, a standard deviation of 10, a power of 80%, and a two-sided alpha level of 5%. The Allocation Ratio was set at 1, implying an equal number of subjects in both online and onsite group.
Considering a dropout up to 2 students per course, equivalent to 17%, we determined that a total of 112 participants would be needed. This calculation factored in 10 clusters of 12 participants per study arm, which we deemed sufficient to assess any changes in learning outcome.
The sample size was estimated using the function n4means from the R package CRTSize [ 25 ].
Recruitment
Participants are PhD students enrolled in 10 courses of “Practical Course in Systematic Review Technique in Clinical Research” and 10 courses of “Getting started: Writing your first manuscript for publication” at the PhD School of the Faculty of Health Sciences, University of Copenhagen, Denmark.
Assignment of interventions: allocation
Randomization will be performed on course-level. The courses are randomized by a computer random number generator [ 26 ]. To get a balanced randomization per year, 2 sets with 2 unique random integers in each, taken from the 1–4 range is requested.
The setting is not included in the course catalogue of the PhD School and thus allocation to online or onsite is concealed until 3–4 weeks before course commencement when a welcome letter with course information including allocation to online or onsite setting is distributed to the students. The lecturers are also informed of the course setting at this time point. If students withdraw from the course after being informed of the setting, a letter is sent to them enquiring of the reason for withdrawal and reason is recorded (Appendix 7).
The allocation sequence is generated by a computer random number generator (random.org). The participants and the lecturers sign up for the course without knowing the course setting (online or onsite) until 3–4 weeks before the course.
Assignment of interventions: blinding
Due to the nature of the study, it is not possible to blind trial participants or lecturers. The outcomes are reported by the participants directly in an online form, thus being blinded for the outcome assessor, but not for the individual participant. The data collection for the long-term follow-up regarding academic achievements is conducted without blinding. However, the external researcher analysing the data will be blinded.
Data collection and management
Data will be collected by the project leader (Table 1 ). Baseline variables and post course knowledge, motivation, and self-efficacy are self-reported through questionnaires in SurveyXact® [ 27 ]. Academic achievements are collected through Google Scholar profiles of the participants.
Given that we are using participant assessments and evaluations for research purposes, all data collection – except for monthly follow-up of academic achievements after the course – takes place either in the immediate beginning or ending of the course and therefore we expect participant retention to be high.
Data will be downloaded from SurveyXact and stored in a locked and logged drive on a computer belonging to the Capital Region of Denmark. Only the project leader has access to the data.
This project conduct is following the Danish Data Protection Agency guidelines of the European GDPR throughout the trial. Following the end of the trial, data will be stored at the Danish National Data Archive which fulfil Danish and European guidelines for data protection and management.
Statistical methods
Data is anonymized and blinded before the analyses. Analyses are performed by a researcher not otherwise involved in the inclusion or randomization, data collection or handling. All statistical tests will be testing the null hypotheses assuming the two arms of the trial being equal based on corresponding estimates. Analysis of primary outcome on short-term learning will be started once all data has been collected for all individuals in the last included course. Analyses of long-term academic achievement will be started at end of follow-up.
Baseline characteristics including both course- and individual level information will be presented. Table 2 presents the available data on baseline.
We will use multivariate analysis for identification of the most important predictors (motivation, self-efficacy, sex, educational background, and knowledge) for best effect on short and long term. The results will be presented as risk ratio (RR) with 95% confidence interval (CI). The results will be considered significant if CI does not include the value one.
All data processing and analyses were conducted using R statistical software version 4.1.0, 2021–05-18 (R Foundation for Statistical Computing, Vienna, Austria).
If possible, all analysis will be performed for “Practical Course in Systematic Review Technique in Clinical Research” and for “Getting started: Writing your first manuscript for publication” separately.
Primary analyses will be handled with the intention-to-treat approach. The analyses will include all individuals with valid data regardless of they did attend the complete course. Missing data will be handled with multiple imputation [ 28 ] .
Upon reasonable request, public assess will be granted to protocol, datasets analysed during the current study, and statistical code Table 3 .
Oversight, monitoring, and adverse events
This project is coordinated in collaboration between the WHO CC (DEN-62) at the Parker Institute, CAMES, and the PhD School at the Faculty of Health and Medical Sciences, University of Copenhagen. The project leader runs the day-to-day support of the trial. The steering committee of the trial includes principal investigators from WHO CC (DEN-62) and CAMES and the project leader and meets approximately three times a year.
Data monitoring is done on a daily basis by the project leader and controlled by an external independent researcher.
An adverse event is “a harmful and negative outcome that happens when a patient has been provided with medical care” [ 29 ]. Since this trial does not involve patients in medical care, we do not expect adverse events. If participants decline taking part in the course after receiving the information of the course setting, information on reason for declining is sought obtained. If the reason is the setting this can be considered an unintended effect. Information of unintended effects of the online setting (the intervention) will be recorded. Participants are encouraged to contact the project leader with any response to the course in general both during and after the course.
The trial description has been sent to the Scientific Ethical Committee of the Capital Region of Denmark (VEK) (21041907), which assessed it as not necessary to notify and that it could proceed without permission from VEK according to the Danish law and regulation of scientific research. The trial is registered with the Danish Data Protection Agency (Privacy) (P-2022–158). Important protocol modification will be communicated to relevant parties as well as VEK, the Joint Regional Information Security and Clinicaltrials.gov within an as short timeframe as possible.
Dissemination plans
The results (positive, negative, or inconclusive) will be disseminated in educational, scientific, and clinical fora, in international scientific peer-reviewed journals, and clinicaltrials.gov will be updated upon completion of the trial. After scientific publication, the results will be disseminated to the public by the press, social media including the website of the hospital and other organizations – as well as internationally via WHO CC (DEN-62) at the Parker Institute and WHO Europe.
All authors will fulfil the ICMJE recommendations for authorship, and RR will be first author of the articles as a part of her PhD dissertation. Contributors who do not fulfil these recommendations will be offered acknowledgement in the article.
This cluster randomized trial investigates if an onsite setting of a research course for PhD students within the health and medical sciences is different from an online setting. The outcomes measured are learning of research methodology (primary), preference, motivation, and self-efficacy (secondary) on short term and academic achievements (secondary) on long term.
The results of this study will be discussed as follows:
Discussion of primary outcome
Primary outcome will be compared and contrasted with similar studies including recent RCTs and mixed-method studies on online and onsite research methodology courses within health and medical education [ 10 , 11 , 30 ] and for inspiration outside the field [ 31 , 32 ]: Tokalic finds similar outcomes for online and onsite, Martinic finds that the web-based educational intervention improves knowledge, Cheung concludes that the evidence is insufficient to say that the two modes have different learning outcomes, Kofoed finds online setting to have negative impact on learning and Rahimi-Ardabili presents positive self-reported student knowledge. These conflicting results will be discussed in the context of the result on the learning outcome of this study. The literature may change if more relevant studies are published.
Discussion of secondary outcomes
Secondary significant outcomes are compared and contrasted with similar studies.
Limitations, generalizability, bias and strengths
It is a limitation to this study, that an onsite curriculum for a full day is delivered identically online, as this may favour the onsite course due to screen fatigue [ 33 ]. At the same time, it is also a strength that the time schedules are similar in both settings. The offer of coffee, tea, water, and a plain sandwich in the onsite course may better facilitate the possibility for socializing. Another limitation is that the study is performed in Denmark within a specific educational culture, with institutional policies and resources which might affect the outcome and limit generalization to other geographical settings. However, international students are welcome in the class.
In educational interventions it is generally difficult to blind participants and this inherent limitation also applies to this trial [ 11 ]. Thus, the participants are not blinded to their assigned intervention, and neither are the lecturers in the courses. However, the external statistical expert will be blinded when doing the analyses.
We chose to compare in-person onsite setting with a synchronous online setting. Therefore, the online setting cannot be expected to generalize to asynchronous online setting. Asynchronous delivery has in some cases showed positive results and it might be because students could go back and forth through the modules in the interface without time limit [ 11 ].
We will report on all the outcomes defined prior to conducting the study to avoid selective reporting bias.
It is a strength of the study that it seeks to report outcomes within the 1, 2 and 4 levels of the Kirkpatrick conceptual framework, and not solely on level 1. It is also a strength that the study is cluster randomized which will reduce “infections” between the two settings and has an adequate power calculated sample size and looks for a relevant educational difference of 20% between the online and onsite setting.
Perspectives with implications for practice
The results of this study may have implications for the students for which educational setting they choose. Learning and preference results has implications for lecturers, course managers and curriculum developers which setting they should plan for the health and medical education. It may also be of inspiration for teaching and training in other disciplines. From a societal perspective it also has implications because we will know the effect and preferences of online learning in case of a future lock down.
Future research could investigate academic achievements in online and onsite research training on the long run (Kirkpatrick 4); the effect of blended learning versus online or onsite (Kirkpatrick 2); lecturers’ preferences for online and onsite setting within health and medical education (Kirkpatrick 1) and resource use in synchronous and asynchronous online learning (Kirkpatrick 5).
Trial status
This trial collected pilot data from August to September 2021 and opened for inclusion in January 2022. Completion of recruitment is expected in April 2024 and long-term follow-up in April 2026. Protocol version number 1 03.06.2022 with amendments 30.11.2023.
Availability of data and materials
The project leader will have access to the final trial dataset which will be available upon reasonable request. Exception to this is the qualitative raw data that might contain information leading to personal identification.
Abbreviations
Artificial Intelligence
Copenhagen academy for medical education and simulation
Confidence interval
Coronavirus disease
European credit transfer and accumulation system
International committee of medical journal editors
Intrinsic motivation inventory
Multiple choice questionnaire
Doctor of medicine
Masters of sciences
Randomized controlled trial
Scientific ethical committee of the Capital Region of Denmark
WHO Collaborating centre for evidence-based clinical health promotion
Samara M, Algdah A, Nassar Y, Zahra SA, Halim M, Barsom RMM. How did online learning impact the academic. J Technol Sci Educ. 2023;13(3):869–85.
Article Google Scholar
Nejadghaderi SA, Khoshgoftar Z, Fazlollahi A, Nasiri MJ. Medical education during the coronavirus disease 2019 pandemic: an umbrella review. Front Med (Lausanne). 2024;11:1358084. https://doi.org/10.3389/fmed.2024.1358084 .
Madi M, Hamzeh H, Abujaber S, Nawasreh ZH. Have we failed them? Online learning self-efficacy of physiotherapy students during COVID-19 pandemic. Physiother Res Int. 2023;5:e1992. https://doi.org/10.1002/pri.1992 .
Torda A. How COVID-19 has pushed us into a medical education revolution. Intern Med J. 2020;50(9):1150–3.
Alhat S. Virtual Classroom: A Future of Education Post-COVID-19. Shanlax Int J Educ. 2020;8(4):101–4.
Cook DA, Levinson AJ, Garside S, Dupras DM, Erwin PJ, Montori VM. Internet-based learning in the health professions: A meta-analysis. JAMA. 2008;300(10):1181–96. https://doi.org/10.1001/jama.300.10.1181 .
Pei L, Wu H. Does online learning work better than offline learning in undergraduate medical education? A systematic review and meta-analysis. Med Educ Online. 2019;24(1):1666538. https://doi.org/10.1080/10872981.2019.1666538 .
Richmond H, Copsey B, Hall AM, Davies D, Lamb SE. A systematic review and meta-analysis of online versus alternative methods for training licensed health care professionals to deliver clinical interventions. BMC Med Educ. 2017;17(1):227. https://doi.org/10.1186/s12909-017-1047-4 .
George PP, Zhabenko O, Kyaw BM, Antoniou P, Posadzki P, Saxena N, Semwal M, Tudor Car L, Zary N, Lockwood C, Car J. Online Digital Education for Postregistration Training of Medical Doctors: Systematic Review by the Digital Health Education Collaboration. J Med Internet Res. 2019;21(2):e13269. https://doi.org/10.2196/13269 .
Tokalić R, Poklepović Peričić T, Marušić A. Similar Outcomes of Web-Based and Face-to-Face Training of the GRADE Approach for the Certainty of Evidence: Randomized Controlled Trial. J Med Internet Res. 2023;25:e43928. https://doi.org/10.2196/43928 .
Krnic Martinic M, Čivljak M, Marušić A, Sapunar D, Poklepović Peričić T, Buljan I, et al. Web-Based Educational Intervention to Improve Knowledge of Systematic Reviews Among Health Science Professionals: Randomized Controlled Trial. J Med Internet Res. 2022;24(8): e37000.
https://www.mentimeter.com/ . Accessed 4 Dec 2023.
https://www.sendsteps.com/en/ . Accessed 4 Dec 2023.
https://da.padlet.com/ . Accessed 4 Dec 2023.
Zackoff MW, Real FJ, Abramson EL, Li STT, Klein MD, Gusic ME. Enhancing Educational Scholarship Through Conceptual Frameworks: A Challenge and Roadmap for Medical Educators. Acad Pediatr. 2019;19(2):135–41. https://doi.org/10.1016/j.acap.2018.08.003 .
https://zoom.us/ . Accessed 20 Aug 2024.
Raffing R, Larsen S, Konge L, Tønnesen H. From Targeted Needs Assessment to Course Ready for Implementation-A Model for Curriculum Development and the Course Results. Int J Environ Res Public Health. 2023;20(3):2529. https://doi.org/10.3390/ijerph20032529 .
https://www.kirkpatrickpartners.com/the-kirkpatrick-model/ . Accessed 12 Dec 2023.
Smidt A, Balandin S, Sigafoos J, Reed VA. The Kirkpatrick model: A useful tool for evaluating training outcomes. J Intellect Dev Disabil. 2009;34(3):266–74.
Campbell K, Taylor V, Douglas S. Effectiveness of online cancer education for nurses and allied health professionals; a systematic review using kirkpatrick evaluation framework. J Cancer Educ. 2019;34(2):339–56.
Miller GE. The assessment of clinical skills/competence/performance. Acad Med. 1990;65(9 Suppl):S63–7.
Ryan RM, Deci EL. Self-Determination Theory and the Facilitation of Intrinsic Motivation, Social Development, and Well-Being. Am Psychol. 2000;55(1):68–78. https://doi.org/10.1037//0003-066X.55.1.68 .
Williams GM, Smith AP. Using single-item measures to examine the relationships between work, personality, and well-being in the workplace. Psychology. 2016;07(06):753–67.
https://scholar.google.com/intl/en/scholar/citations.html . Accessed 4 Dec 2023.
Rotondi MA. CRTSize: sample size estimation functions for cluster randomized trials. R package version 1.0. 2015. Available from: https://cran.r-project.org/package=CRTSize .
Random.org. Available from: https://www.random.org/
https://rambollxact.dk/surveyxact . Accessed 4 Dec 2023.
Sterne JAC, White IR, Carlin JB, Spratt M, Royston P, Kenward MG, et al. Multiple imputation for missing data in epidemiological and clinical research: Potential and pitfalls. BMJ (Online). 2009;339:157–60.
Google Scholar
Skelly C, Cassagnol M, Munakomi S. Adverse Events. StatPearls Treasure Island: StatPearls Publishing. 2023. Available from: https://www.ncbi.nlm.nih.gov/books/NBK558963/ .
Rahimi-Ardabili H, Spooner C, Harris MF, Magin P, Tam CWM, Liaw ST, et al. Online training in evidence-based medicine and research methods for GP registrars: a mixed-methods evaluation of engagement and impact. BMC Med Educ. 2021;21(1):1–14. Available from: https://www.ncbi.nlm.nih.gov/pmc/articles/PMC8439372/pdf/12909_2021_Article_2916.pdf .
Cheung YYH, Lam KF, Zhang H, Kwan CW, Wat KP, Zhang Z, et al. A randomized controlled experiment for comparing face-to-face and online teaching during COVID-19 pandemic. Front Educ. 2023;8. https://doi.org/10.3389/feduc.2023.1160430 .
Kofoed M, Gebhart L, Gilmore D, Moschitto R. Zooming to Class?: Experimental Evidence on College Students' Online Learning During Covid-19. SSRN Electron J. 2021;IZA Discussion Paper No. 14356.
Mutlu Aİ, Yüksel M. Listening effort, fatigue, and streamed voice quality during online university courses. Logop Phoniatr Vocol :1–8. Available from: https://doi.org/10.1080/14015439.2024.2317789
Download references
Acknowledgements
We thank the students who make their evaluations available for this trial and MSc (Public Health) Mie Sylow Liljendahl for statistical support.
Open access funding provided by Copenhagen University The Parker Institute, which hosts the WHO CC (DEN-62), receives a core grant from the Oak Foundation (OCAY-18–774-OFIL). The Oak Foundation had no role in the design of the study or in the collection, analysis, and interpretation of the data or in writing the manuscript.
Author information
Authors and affiliations.
WHO Collaborating Centre (DEN-62), Clinical Health Promotion Centre, The Parker Institute, Bispebjerg & Frederiksberg Hospital, University of Copenhagen, Copenhagen, 2400, Denmark
Rie Raffing & Hanne Tønnesen
Copenhagen Academy for Medical Education and Simulation (CAMES), Centre for HR and Education, The Capital Region of Denmark, Copenhagen, 2100, Denmark
You can also search for this author in PubMed Google Scholar
Contributions
RR, LK and HT have made substantial contributions to the conception and design of the work; RR to the acquisition of data, and RR, LK and HT to the interpretation of data; RR has drafted the work and RR, LK, and HT have substantively revised it AND approved the submitted version AND agreed to be personally accountable for their own contributions as well as ensuring that any questions which relates to the accuracy or integrity of the work are adequately investigated, resolved and documented.
Corresponding author
Correspondence to Rie Raffing .
Ethics declarations
Ethics approval and consent to participate.
The Danish National Committee on Health Research Ethics has assessed the study Journal-nr.:21041907 (Date: 21–09-2021) without objections or comments. The study has been approved by The Danish Data Protection Agency Journal-nr.: P-2022–158 (Date: 04.05.2022).
All PhD students participate after informed consent. They can withdraw from the study at any time without explanations or consequences for their education. They will be offered information of the results at study completion. There are no risks for the course participants as the measurements in the course follow routine procedure and they are not affected by the follow up in Google Scholar. However, the 15 min of filling in the forms may be considered inconvenient.
The project will follow the GDPR and the Joint Regional Information Security Policy. Names and ID numbers are stored on a secure and logged server at the Capital Region Denmark to avoid risk of data leak. All outcomes are part of the routine evaluation at the courses, except the follow up for academic achievement by publications and related indexes. However, the publications are publicly available per se.
Competing interests
The authors declare no competing interests
Additional information
Publisher’s note.
Springer Nature remains neutral with regard to jurisdictional claims in published maps and institutional affiliations.
Supplementary Information
Supplementary material 1., supplementary material 2., supplementary material 3., supplementary material 4., supplementary material 5., supplementary material 6., supplementary material 7., rights and permissions.
Open Access This article is licensed under a Creative Commons Attribution 4.0 International License, which permits use, sharing, adaptation, distribution and reproduction in any medium or format, as long as you give appropriate credit to the original author(s) and the source, provide a link to the Creative Commons licence, and indicate if changes were made. The images or other third party material in this article are included in the article's Creative Commons licence, unless indicated otherwise in a credit line to the material. If material is not included in the article's Creative Commons licence and your intended use is not permitted by statutory regulation or exceeds the permitted use, you will need to obtain permission directly from the copyright holder. To view a copy of this licence, visit http://creativecommons.org/licenses/by/4.0/ . The Creative Commons Public Domain Dedication waiver ( http://creativecommons.org/publicdomain/zero/1.0/ ) applies to the data made available in this article, unless otherwise stated in a credit line to the data.
Reprints and permissions
About this article
Cite this article.
Raffing, R., Konge, L. & Tønnesen, H. Learning effect of online versus onsite education in health and medical scholarship – protocol for a cluster randomized trial. BMC Med Educ 24 , 927 (2024). https://doi.org/10.1186/s12909-024-05915-z
Download citation
Received : 25 March 2024
Accepted : 14 August 2024
Published : 26 August 2024
DOI : https://doi.org/10.1186/s12909-024-05915-z
Share this article
Anyone you share the following link with will be able to read this content:
Sorry, a shareable link is not currently available for this article.
Provided by the Springer Nature SharedIt content-sharing initiative
- Self-efficacy
- Achievements
- Health and Medical education
BMC Medical Education
ISSN: 1472-6920
- General enquiries: [email protected]

An official website of the United States government
The .gov means it’s official. Federal government websites often end in .gov or .mil. Before sharing sensitive information, make sure you’re on a federal government site.
The site is secure. The https:// ensures that you are connecting to the official website and that any information you provide is encrypted and transmitted securely.
- Publications
- Account settings
Preview improvements coming to the PMC website in October 2024. Learn More or Try it out now .
- Advanced Search
- Journal List
- Springer Nature - PMC COVID-19 Collection
- PMC10160711

The Emergence of Mpox: Epidemiology and Current Therapeutic Options
Samriddhi ranjan.
1 College of Public Health, George Mason University, 4400 University Drive Fairfax, Fairfax, VA 22030 USA
Kanupriya Vashishth
2 Advance Cardiac Centre Department of Cardiology, PGIMER, Chandigarh, 160012 India
3 NGO Praeventio, Tartu, Estonia
Hardeep Singh Tuli
4 Department of Biotechnology, Maharishi Markandeshwar Engineering College, Maharishi Markandeshwar (Deemed to Be University), Mullana-Ambala, Haryana, 133207 India
Associated Data
This document includes citations for all the data that were analysed throughout the literature review.
The world recently witnessed the emergence of new epidemic outbreaks like COVID-19 and mpox. The 2022 outbreak of mpox amid COVID-19 presents an intricate situation and requires strategies to combat the status quo. Some of the challenges to controlling an epidemic include present knowledge of the disease, available treatment options, appropriate health infrastructures facilities, current scientific methods, operations concepts, availability of technical staff, financial funds, and lastly international policies to control an epidemic state. These insufficiencies often hinder the control of disease spread and jeopardize the health of countless people. Also, disease outbreaks often put a huge burden on the developing economies. These countries are the worst affected and are immensely dependent on assistance provided from the larger economies to control such outbreaks. The first case of mpox was reported in the 1970s and several outbreaks were detected thereafter in the endemic areas eventually leading to the recent outbreak. Approximately, more than 80,000 individuals were infected, and 110 countries were affected by this outbreak. Yet, no definite vaccines and drugs are available to date. The lack of human clinical trials affected thousands of individuals in availing definite disease management. This paper focuses on the epidemiology of mpox, scientific concepts, and treatment options including future treatment modalities for mpox.
Introduction
Amidst the COVID-19 pandemic, another public health concern emerged as a potential threat to afflict people globally, i.e. an abrupt increase in the incidence of mpox (monkeypox) cases. Indeed, starting from mid-May 2022, cases of human mpox have significantly risen in several non-endemic countries worldwide, leading to the declaration of the ongoing outbreak of mpox as a Public Health Emergency of International Concern (PHEIC) by the World Health Organization (WHO) in July 2022 [ 1 , 2 ]. Mpox disease is caused by the mpox virus (MPXV), a double-stranded DNA virus from the Orthopoxvirus genus, belonging to the Poxviridae family [ 2 , 3 ]. The same genus includes the variola virus, a known causative agent of smallpox [ 2 ]. Genetically, MPXV is identified with two types of clads. Clad I, also known as Congo Basin clad, is mostly clustered in the Central-South Cameroon region till DRC. Infections from this clad are more severe with case fatality rates (CRF) > 10%. Clad II also referred to as West African clad, commonly distributed in western Cameroon to the Sierra Leon area, is further divided into sub-clad groups as IIa and IIb (also now referred to as clad III) having a CRF < 1% [ 4 , 5 ]. Overall reported human case fatality rates (CFRs) range between 3.6 and 10.6% in the endemic regions [ 2 ]. In the current 2022 outbreak, clad IIb was predominant [ 6 , 7 ]. To date, the exact animal reservoir for the mpox virus (MPXV) has remained unknown. However, few native African rodents (Gambian giant rats) and squirrels are suspected to be natural reservoirs of the virus. Common species which were frequently infected with MPXV are squirrels, Gambian giant rats, strip mice, dormice, and primates [ 8 ].
Emergence of Mpox
The first outbreak of mpox was reported in 1958 in a group of 10 captive monkeys at the Statens Seruminstitut, Copehengan, Denmark, and Centre d’Enseignement et de Recherches de Medecine aeronautique, Paris. No human infection was reported in individuals who were in close contact with infected monkeys. Subsequently, the mpox outbreak occurred for the first time in humans between 1970 to 1971 [ 9 ]. The first case was reported in a 9-month-old boy residing in a remote village of the Democratic Republic of Congo (DRC), admitted to a local hospital suspected of smallpox infection. Samples from infected individuals were sent to the WHO Smallpox Reference Center, Moscow, revealing mpox infections in virus isolates [ 10 ]. When inspected from family, monkeys were part of the diet, and their skins were also processed in this area. However, no other cases including secondary infections were reported in the community. Nonetheless, seven more cases were reported during this time period [ 9 ]. The World Health Organization in 1967 took the initiative to collaborate with laboratories to conduct cooperative studies. This was to conduct serological surveys, identify mpox outbreaks, and determine the natural foci of the virus. However, these surveys failed to state any major findings and concluded mpox is not a widespread disease and can exist only in the local environment [ 9 ]. Ever since, there has been a subsequent upsurge of mpox cases, mostly recorded in the DRC province. Approximately, 80% of the cases were reported in this region from the years 1970–1997 [ 11 ]. For the past five decades, DRC is the most affected country with mpox; no other country had reported an mpox outbreak to such an extent [ 12 ].
The initial mpox outbreak that was reported in DRC mostly affected children below 10 years of age. A slight male predominance was observed in the systemic review conducted by Beer and Rao [ 11 ]. Most of the initial outbreaks occurred among individuals living in small rural areas or residing close to humid evergreen tropical forests or individuals commonly involved with bushmeat hunting [ 11 ]. Geographically, the spread of infection from 1970 to 2003 concentrated in the Central and Western parts of Africa (Table 1). Countries which frequently reported infections were Cameroon, the Central African Republic, Gabon, Sierra Leone, Liberia, Nigeria, and Cote d’Ivoire, yet greater outbreaks were mostly detected in DRC [ 13 ]. An active surveillance programme was carried out by WHO between the years 1981 to 1986 reporting total confirmed cases of 338 and 33 deaths, an almost 20 times rise in the reported case after the surveillance [ 10 , 11 ]. A slight drop in the incidence of disease was observed between the period of 1993–1995. But soon after, DRC witnessed a major outbreak from 1996–1997 [ 13 ]. A total of 511 cases were recorded with a surge in secondary transmission rates of up to 78% and a fatality rate between 1 and 5% [ 10 , 13 ].
In 2003, the mpox outbreak occurred for the first time in the USA, outside the African continent. The index case was a 3-year-old girl, bitten by an infected prairie dog, imported from Ghana along with other African rodents to the USA [ 14 ]. A total of 71 cases were reported, including both suspected and laboratory-confirmed cases, as per the CDC report [ 15 ]. During the period of 2005, mpox was registered for the first time in the dry savannah region of Sudan. Overall, 40 cases both suspected and confirmed were recorded. In this outbreak, a change in the genomic structure of MPXV was observed as compared to the MPXV traditionally reported in DRC suggesting the adaptability of MPVX in dry regions from humid evergreen tropical forests [ 10 ]. In the year 2018, mpox travelled for the first time to the UK and was reported in the European continent. Only two cases were registered, in individuals, which had a travel history to Nigeria [ 16 ]. Nevertheless, with the advent of 2022, the world saw a major outbreak of mpox (Table (Table1 1 ).
Decade-wise spread of mpox across different countries between 1970 and 2020 9,10

Mpox Outbreak 2022
Mpox is endemic in Central and West Africa, where hundreds of cases were detected annually for many years, acquired mostly from wild animals and most rarely from infected humans [ 1 , 3 ], which results in a sporadic spillover of cases in humans as observed in the MPVX endemic regions [ 5 ]. However, in the 2022 outbreak of mpox, most of the cases were reported in non-endemic countries like N. America, S. America, and Europe (Fig. 1 ) [ 17 ]. Although the origin of the 2022 outbreak is still unknown, it is highly likely that the initial infection has been imported from an endemic country, allowing the circulation of the virus through close physical contacts among humans [ 1 , 18 ]. For the first time, mpox was documented with transmission chains in countries which had no immediate contact with Central or Western Africa [ 19 , 20 ]. This suggests a probability of undetected MPXV circulating in the local population in the outbreak-hit regions causing disease transmission in humans [ 17 ]. Being a DNA virus, mpox is more stable in nature and may have possibly evolved as a potent virus causing infections in humans in the due course of time [ 17 ]. Daniel et al. reported 6–12 times higher mutation rates in mpox as previously estimated [ 6 ]. Human to human transmissibility of mpox has also evolved in these decades [ 21 , 22 ]. Vertical infection of mpox has been also reported. Pregnant mothers infected with mpox had miscarriages during the first trimester of pregnancy [ 6 ]. Perinatally acquired mpox infection was registered in a 9-day year old neonate as well [ 23 ]. Transmissibility of infection within the family especially from parents to children have also been stated to increase [ 20 , 22 ]. The degree of transmissibility of the diseases, popularly known as R 0 , reported in the 1980 for mpox was 0.83. However, in the 2022 outbreak, the R 0 reported was 1.1–2.4 [ 21 , 24 ]. Pan et al. suggested the increase in the R 0 is due to decreased immunity of individuals due to the absence of smallpox immunization and high contact rates of infection in the MSM community [ 6 ].

Countries reporting mpox historically vs countries reporting an mpox outbreak as recorded in an early March 2023 report by CDC 19
As per the WHO, till 17 March 2023, the total confirmed cases for mpox were 86,601, with 1265 probable cases reported with 112 deaths. Globally, 110 countries were affected by mpox so far (Fig. 2 ) [ 20 ]. Approximately 34.7% of cases were reported in America, the worst affected country [ 20 ]. Majority of the infection occurred through household contacts (43%) and by sexual encounters (43%) [ 20 , 22 ]. Commonly affected individuals were young males who were not vaccinated against smallpox and have had sex with men. There was a slight male predisposition, with the median age reported as 34 years (IQR: 29–41). Around, 98% of individuals who were infected were either gay or bisexual, among which 41% of the people were HIV infected [ 4 ].

Mpox number of cases and deaths recorded in early March 2023 across the continent as per the report by CDC 19
On 23 July 2022, mpox was declared a public health emergency by the Public Health Emergency of International Concern (PHEIC), depicting a risk of international spread, along with significant international coordination to control the disease [ 25 ].
Clinical presentation of this disease includes three distinct phases, i.e. incubation, prodrome, and rash [ 2 ]. The incubation period can last for 3 to 20 days with the median being 7 days followed by the prodrome phase that is characterized by lethargy, myalgia, headache, fever, and lymphadenopathy which may last up to 5 days (Fig. 3 ) [ 2 , 4 , 18 ]. Lymphadenopathy is one of the critical features of the progression of the disease and often reported before the development of skin lesions [ 18 ]. Fever is usually followed by multiple papular, ulcerative, and vesiculopustular skin lesions [ 4 ], which progress from macules to papules, vesicles, pustules, crusts, and lastly scab, presenting for up to 4 weeks [ 2 , 18 ]. In 95% of the cases, skin lesions appears [ 4 ]. Common anatomical sites for skin lesions were anogenital with approximately 73% of cases followed by trunk, arms or legs, face, and eventually palms and soles, only accounting for 10% of the cases. Lesions developed contain infectious virus particles, through which the infection can be transmitted directly with human contacts [ 2 ]. Secondary complications include pneumonia, encephalitis, keratitis, gastroenteritis, sepsis, and secondary bacterial infections, affecting mostly patients with a previous diagnosis of HIV infection [ 2 , 4 , 5 ].

Common features reported in mpox infection 4
Current Treatment Modalities and Prevention
The strategy for the prevention and treatment of mpox is very similar to the treatment of Orthomyxovirus infection [ 26 ]. The 2022 outbreak revealed the urgency to control the spread of mpox as it has caused a potential threat in many countries [ 27 ]. Presently, there is no definitive cure for mpox infection, mild symptoms are manageable, and further complications can be avoided in patients with mpox with the help of supportive care [ 28 , 29 ]. Studies have depicted that patients with mild symptoms recover without any treatment [ 30 – 32 ]. Treatment options available for smallpox are also effective in the treatment of mpox, as the clinical presentation of mpox and smallpox is very similar. These include the vaccinia vaccine, vaccinia immune globulin (IVG), and antiviral agents such as cidofovir, tecovirimat, and brincidofovir [ 32 ]. Furthermore, CDC recommends the use of the potential treatment options should be done depending upon the severity of the cases and for serious emergency cases, as the current drugs pose severe adverse effects, and their therapeutic efficacy is still uncertain [ 33 ]. Antiviral drugs are a choice of treatment in immunocompromised patients, in patients with complicated lesions, in pregnant women infected with mpox, in breast-feeding women, and in the paediatric population [ 34 ]. Tecovirimat is the first line of action antiviral recommended for the treatment of smallpox; it works by inhibiting the viral envelope protein, thereby blocking the final steps of virus maturation and release from infected cells, inhibiting the spread. As per the CDC guidelines, emergency access use of tecovirimat is allowed for compassionate use, for the treatment of Orthopoxvirus infections, such as mpox [ 35 , 36 ]. Cidofovir and its oral analogue brincidofovir are commonly approved drugs for the treatment of smallpox; both act by inhibiting viral DNA polymerase. Different studies have evaluated the effect of brincidofovir against Orthopoxvirus infections [ 37 ]. Studies done by Lanier et al. and others on the effect of cidofovir and brincidofovir have been evaluated for mpox with some success [ 34 , 37 ]. As per the recommended guidelines by CDC, preexposure smallpox vaccination has been advised for veterinarians, monkeypox contacts, healthcare workers caring for mpox patients, researchers, and field investigators [ 38 ]. Prior immunization with the smallpox vaccine has demonstrated some proven protective effects against mpox due to the cross-protective immunity provided by the smallpox vaccine. Furthermore, the severity of clinical manifestations is also reduced [ 39 ]. Currently, three available smallpox vaccines with the US national stockpile, i.e. JYNNEOSTM, ACAM2000, have been licenced (2007) for smallpox, the most recent being Aventis Pasteur Smallpox Vaccine (APSV) which could be potentially used for mpox on a case-to-case basis, under an investigational new drug (IND) protocol. JYNNEOSTM, a third-generation and live viral vaccine, is produced from the modified vaccinia Ankara-Bavarian Nordic [ 40 – 42 ]. Licenced in 2019, JYNNEOSTM is an attenuated non-replicating orthopoxvirus. It is now indicated for both smallpox and mpox prevention for adults. Further, ACAM2000, a second-generation vaccine constituted of live vaccinia virus, under the emergency access ACAM2000 is allowed for mpox during the outbreak. Researchers have demonstrated that these vaccines can be used as pre- and post-treatment options, i.e. either in preventing the infection and the disease or in ameliorating the infection and disease [ 34 , 43 , 44 ]. Studies have demonstrated that pregnant women, children less than 8 years of age, and immunocompromised patients should be given antiviral treatment than vaccination. These vaccines, although approved, have shown some local and systematic side effects such as fever, muscle pain, vaccinia, abdominal and back pain, fatigue, headache, lymphadenopathy, etc. [ 42 – 44 ]. Researchers have also highlighted the need for maintaining appropriate social barriers such as avoiding close contact with affected individuals, avoiding contact with skin lesions of individuals infected with MPXV, etc. [ 44 – 46 ]. Vaccinia immune globulin intravenous (VIGIV) is a choice of treatment in case of severe infection with mpox, though there is a paucity of data about its effectiveness in treating mpox. VIGIV is also under SNS and can be administered under investigational new drugs held by CDC [ 29 , 30 , 47 – 49 ]. Therefore, the treatment options and the repurposing of vaccines need to be considered on a case-to-case basis depending on the severity of cases and the immune state of patients [ 50 ] (Fig. (Fig.4 4 ).

A Symptoms and B mechanism of action of mpox antiviral therapy: cidofovir, brincidofovir, vaccinia immune globulin, and tecovirimat [ 50 ]
Key Fundamental Findings of the Narrative Review
Some major key findings related to mpox are as follows: mpox was solely endemic to the region of DRC [ 11 ]. There has been a slow and steady increase in mpox cases which has adapted itself to develop into the current outbreak. Secondly, the 1996–1997 DRC outbreak highlighted the increase in secondary transmission rates of mpox, potentially getting adapted to spread in the human population [ 13 ]. Thirdly, the MPXV had adapted to thrive itself from the humid evergreen regions to the dry savannah region of Sudan, as observed in the 2005 outbreak, thus further demonstrating its environmental adaptability to flourish [ 10 ]. Lastly, international travel and commerce have given a wider chance for the disease to spread as reported in the 2003 and 2018 outbreaks of mpox in the USA and the UK [ 14 , 16 ]. All these above factors have led to the 2022 outbreak of mpox, affecting every continent across the globe (Fig. 5 ).

Global spread of mpox in 2023 outbreak 19
Most of the consistent outbreak guidelines available from WHO or CDC only account for people with a high risk of exposure; these guidelines are based on the best available evidence which is based upon risk–benefit analysis and other factors [ 51 ]. Available drugs for the treatment of choice are also limited and lack evidence-based studies in humans [ 29 , 30 , 48 ]. This depicts an extensive need to increase the sustainable funding option to enhance our understanding of the development of new drugs and vaccines to curtail the spread of mpox.
Implication in Future Research
The environmental, behavioural, and social reasons behind the 2022 mpox outbreak remain unknown to date [ 1 ]. A deeper understanding of mpox genetics and biochemistry is essential to control its outbreak. It is currently unclear how mpox is closely related and linked to the viral strain that is primarily found in western Africa, as well as the potential routes of rapid transmission. To further understand the immune defence mechanisms against MPXV, more research is needed on the human systemic and mucosal immune responses. As DNA viruses are more adept to correct mutations; therefore, it is unlikely that the mpox virus will suddenly change during human transmission [ 24 , 52 ]. It is yet unknown, whether vaccinations and earlier infections have given the population immunity. Additionally, exploratory studies are required to pinpoint the precise mpox virus reservoir, understand how the virus spreads naturally, and determine the causes of the present increase in cases across several nations. Currently, no potent drugs are available and limited evidence-based studies are being conducted for the treatment of mpox [ 29 ]. Most of the available choices of treatment are discussed in this paper (Fig. 6 ). Therefore, it becomes essential to investigate the domain of natural products with antiviral properties. This provides alternative treatment options, to prevent human to human spread of infection and restrict virus amplification in the host organisms. There is a recent increased interest among the scientific community to look into the numerous bioactivities of structurally unrelated natural compounds [ 53 , 54 ]. Plant-derived polyphenol resveratrol has beeb shown to significantly suppress replication of MPXV affecting probably the viral DNA synthesis and inducing a comparable effect to the well-characterized Orthopoxvirus inhibitor, i.e. cytosine-1-β- d -arabinofuranoside (AraC) [ 55 ]. Due to the pleiotropic action of natural compounds and lack of systemic toxicity, plant-derived agents may represent target compounds to be explored in future clinical trials to enrich the drug arsenal against Orthopoxvirus infections. Parallel to this, early detection of infected patients who are potentially capable of transmitting the infection is also crucial, pointing to the need for improved diagnosis (particularly in atypical clinical presentations and asymptomatic cases), and better availability of molecular tests. Besides, such continual efforts of preclinical scientists and pharmaceutical companies, availability of health infrastructures, and medical staff are of critical importance—a situation still aggravated by the ongoing COVID-19 pandemic. A high-risk patient population is possibly in danger of mpox nosocomial transmission and deserves more attention. Therefore, it is crucial to administer the proper supportive care [ 24 ]. Consequently, it is necessary to improve genomic sequencing capabilities to identify the mpox viral clade(s). The primary necessities are to combat the spread of mpox while dealing with the ongoing COVID-19 pandemic and to include suitable and timely information campaigns for people at risk. It is challenging to create an evidence-based classification of drug safety and effectiveness having a brief history of mpox. Further studies on various animal models, which may affect medication exposure, are also encouraged. The focus of larger research should be on identifying the patients who are most at risk for consequences from mpox infection as well as the best timing for initiating and completing antiviral therapy.

Different treatment modalities in mpox
The emergence of new diseases is one of the incessant threats which mankind can face. Persistent interference between the environment and humans creates an opportunity for new infections to evolve. Over 75% of the pathogens, which are newly emerging, are zoonotic in nature [ 56 ]. Several diseases like HIV/AIDS, Nipah, SARS, and Ebola including mpox have recently appeared. International travel and commerce and human behaviour often help disease to spread [ 56 ]. With the first emergence of mpox in 1958, little is still known about its reservoir host and vector of the disease. Despite repeated outbreaks of mpox over the past years, it has failed to gather scientific attention. There is a lack of understanding of mpox transmission dynamics and disease evolution. In the areas endemic to mpox, regular disease surveillance is lacking. This also includes the need to promote funding for capacity building required for surveillance of the disease, research activities, and testing facilities [ 17 , 57 ]. The role of central bodies like the World Health Organization plays a major role in controlling such outbreaks. However, non-compliance to guidelines and regulations by health agencies like WHO severely impacts the control measures [ 25 ]. Boosting vaccine development and effective drug development is essential to prevent future outbreaks. In addition, new plant-derived products could be further developed and can be promoted as they potentially have lesser side effects for mpox treatment.
Abbreviations
Mpox | Monkeypox |
MPXV | Mpox virus |
PHEIC | Public Health Emergency of International Concern |
DRC | Democratic Republic of Congo |
SNS | Strategic National Stockpile |
VIGIV | Vaccinia immune globulin intravenous |
Author Contribution
SR, KV, and KS: conceptualization and writing; HST: editing and proofreading. All authors reviewed the manuscript.
Data Availability
Compliance with ethical standards.
Not applicable.
All authors have their consent to publish.
The authors declare no competing interests.
This article does not contain any studies with human or animal subjects performed by any of the authors.
Publisher's Note
Springer Nature remains neutral with regard to jurisdictional claims in published maps and institutional affiliations.
Contributor Information
Samriddhi Ranjan, Email: ude.umg.evilnosam@najnars .
Kanupriya Vashishth, Email: [email protected] .
Katrin Sak, Email: [email protected] .
Hardeep Singh Tuli, Email: [email protected] .
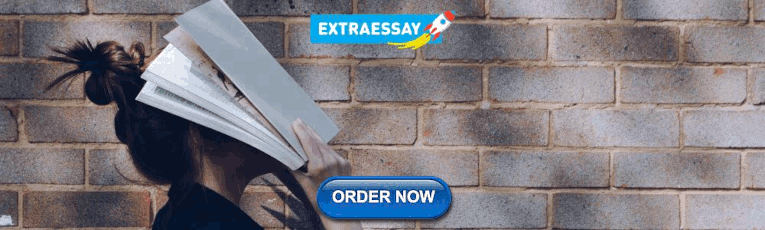
IMAGES
COMMENTS
Google Scholar provides a simple way to broadly search for scholarly literature. Search across a wide variety of disciplines and sources: articles, theses, books, abstracts and court opinions.
The 4 main ethical principles, that is beneficence, nonmaleficence, autonomy, and justice, are defined and explained. Informed consent, truth-telling, and confidentiality spring from the principle of autonomy, and each of them is discussed. In patient care situations, not infrequently, there are conflicts between ethical principles (especially ...
Prepare for medical visits with help from Google and AHRQ by Dave Greenwood. Google Keyword Blog | 2-Dec-2020. Blog Posts A Collaborative Approach to Shaping Successful UX Critique Practices by Anna Lurchenko. Google Design Blog | 29-Jul-2020 ... Google Research Blog | 2-Mar-2018. Publications
1. INTRODUCTION. Nursing is the largest profession in health care, with continued growth expected over the next several years (Grady & Hinshaw, 2017).Nursing science plays a critical role in addressing health challenges, generating new knowledge and translating evidence to practice to improve patient outcomes (Grady, 2017; Powell, 2015).Furthermore, nursing science integrates biobehavioural ...
Transformative healthcare technologies build upon translational research to realize long-term potential. Our Health AI team embraces a model of embedded research and development where scientists work in tight integration with clinical, product, and engineering teams, collaborating with academic and clinical partners globally to conduct research ...
Med-PaLM harnesses the power of Google's large language models, which we have aligned to the medical domain and evaluated using medical exams, medical research, and consumer queries. Our first version of Med-PaLM, preprinted in late 2022 and published in Nature in July 2023 , was the first AI system to surpass the pass mark (>60%) in the U.S ...
Abstract. Background: Medical literature searches provide critical information for clinicians. However, the best strategy for identifying relevant high-quality literature is unknown. Objectives: We compared search results using PubMed and Google Scholar on four clinical questions and analysed these results with respect to article relevance and ...
Research shows that 2% of hospitalized patients experience serious preventable medication-related incidents that can be life-threatening, cause permanent harm, or result in death. Published in Clinical Pharmacology and Therapeutics, our best-performing AI model was able to anticipate physician's actual prescribing decisions 75% of the time ...
This "Cited by" count includes citations to the following articles in Scholar. The ones marked * may be different from the article in the profile. Add co-authors Co-authors. Follow. New articles by this author. ... Journal of medical Internet research 20 (11), e11519, 2018. 22: 2018:
Through computer vision AI and image search capabilities, we are developing a tool to help individuals better research & identify their skin, hair, and nail conditions. The tool supports hundreds of conditions, including more than 80% of the conditions seen in clinics and more than 90% of the most commonly searched conditions.
The following articles are merged in Scholar. Their combined citations are counted only for the first article. Merged citations. ... Clinical Cancer Research 5 (7), 1619-1628, 1999. 158: 1999: Conservation of copy number profiles during engraftment and passaging of patient-derived cancer xenografts.
This systematic scoping review of the literature aims to determine the extent of research into the medical research sex and gender gap and to assess the extent of misogyny, if any, in modern medical research. Methods: Initial literature searches were conducted using PubMed, Science Direct, PsychINFO and Google Scholar. Articles published ...
Astrid Stephan. npj Digital Medicine (2023) Artificial intelligence (AI) driven by machine learning (ML) algorithms is a branch in computer science that is rapidly gaining popularity within the ...
The following articles are merged in Scholar. Their combined citations are counted only for the first article. Merged citations. ... Trends in medical research 2 (2), 108-112, 2007. 20: 2007: Influence of selected formulation variables on the preparation of peptide loaded lipospheres.
Google Scholar is the largest database in the world of its kind. ... COVID-19 research dominated new arrivals in the list, but we're also featuring a popular AI paper from 2016, and research ...
Academic rewards system. Academics' professional standing depends on demonstrating productivity through publication, 3 with disproportionate rewards offered to those who attain publication in 'luxury journals' with high impact factors. 4 Journal impact factors do little to capture the quality or value of individual research articles and can be manipulated. 5 The extraordinary ...
Volitional activation of remote place representations with a hippocampal brain-machine interface. C Lai, S Tanaka, TD Harris, AK Lee. Science 382 (6670), 566-573. , 2023. 15. 2023. Learning produces a hippocampal cognitive map in the form of an orthogonalized state machine.
The disruption of health and medical education by the COVID-19 pandemic made educators question the effect of online setting on students' learning, motivation, self-efficacy and preference. In light of the health care staff shortage online scalable education seemed relevant. Reviews on the effect of online medical education called for high quality RCTs, which are increasingly relevant with ...
[PMC free article] [Google Scholar] 18. World Medical Association. ... Ethical principles for medical research involving human subjects. JAMA. 2013; 310:2191. [Google Scholar] 19. Hall R, McKnight D, Cox R, Coonan T. Guidelines on the ethics of clinical research in anesthesia. Can J Anesth. 2011; 58:1115-24.
Journal of Medical Research and Innovation 1 (1), 21-29. , 2017. 20. 2017. Knowledge, attitude and practice of preventing the occurrence of work-related musculoskeletal disorders among doctors in University of Port-Harcourt Teaching Hospital. BC Ephraim-Emmanuel, R Ogbomade, BN Idumesaro, I Ugwoke.
The lack of human clinical trials affected thousands of individuals in availing definite disease management. This paper focuses on the epidemiology of mpox, scientific concepts, and treatment options including future treatment modalities for mpox. Keywords: Mpox, DNA virus infections, Orthopoxvirus, Public health emergency.
Search Help. Get the most out of Google Scholar with some helpful tips on searches, email alerts, citation export, and more. Your search results are normally sorted by relevance, not by date. To find newer articles, try the following options in the left sidebar: click the envelope icon to have new results periodically delivered by email.
2013. Visualization and interpretation of convolutional neural network predictions in detecting pneumonia in pediatric chest radiographs. S Rajaraman, S Candemir, I Kim, G Thoma, S Antani. Applied Sciences 8 (10), 1715. , 2018. 316. 2018. A review on lung boundary detection in chest X-rays. S Candemir, S Antani.
Taxonomy and distribution of narrow-mouth frogs of the genus Microhyla Tschudi, 1838 (Anura: Microhylidae) from Vietnam with descriptions of five new species. NA Poyarkov Jr, AB Vassilieva, NL Orlov, EA Galoyan, TTA Dao, DTT Le, ... NL Orlov, NA Poyarkov, AB Vassilieva, NB Ananjeva, TT Nguyen, ...