Wilcoxon rank sum test
Kruskal-Wallis test
Continuous | test or ANOVA | Pearson’s correlation coefficient | Spearman’s rank correlation coefficient |
ANOVA=analysis of variance.
Twenty-five preterm infants on mechanical ventilation received varying doses of hydrocortisone for 9 days in an attempt to achieve extubation. Baseline blood pressure was measured before hydrocortisone therapy and daily during therapy. Blood pressure was measured in 50 age-matched infants who did not receive hydrocortisone. Hypertension was defined as systolic blood pressure above 90th percentile for postmenstrual age . Based on Table 2 and assuming that blood pressure and hydrocortisone dose are normally distributed, the choice of statistical test is as follows:
- Classify patients into hydrocortisone treated and not treated and hypertensive and not hypertensive (two dichotomous categorical variables): χ 2 test
- Compare change in mean blood pressure from baseline and compare hydrocortisone versus no hydrocortisone: t test
- Compare cumulative dose of hydrocortisone (mg/kg) to change in systolic blood pressure from baseline (two continuous variables): correlation
2. Distribution of Data
Distribution of data includes measures of central tendency, dispersion, and distribution.
- The mean is the sum of all observations divided by the number of observations.
- The mean is the measure of central tendency for interval and ratio data and is the “average” value for the data.
- It is representative of all data points and is the most efficient estimator of the middle of a normal (Gaussian) distribution; however, it is inappropriate as a measure of central tendency if data are skewed.
- The mean is influenced by outlying values, particularly in small samples.
- Mean is commonly used for interval and ratio data.
- The median is that value such that half of the data points fall above it and half below it. It is the middle value when data are sequentially ordered from lowest to highest or highest to lowest.
- It is not influenced by outlying values and is more appropriate for data that are not normally distributed (skewed data).
- Median is also commonly used for ordinal data (eg, Apgar score).
- Mode is the most frequently occurring observation.
- It is particularly useful while describing data distributed in a bimodal pattern when mean and median are not appropriate. For example, nosocomial infections in the NICU have a bimodal gestational age at birth distribution. Extremely preterm infants with percutaneous lines and full-term infants with surgical procedures are at risk. The mean or median age of these infants with nosocomial infections may be 34 weeks and is not representative of the central tendency in this population.
- Mode is commonly used with nominal data (eg, commonest maternal blood group associated with neonatal hyperbilirubinemia).

A. Types of symmetric curves with different levels of kurtosis (peak or flatness). As the curves are normally distributed and symmetric, the mean, median, and mode values are the same. B. Skewed curves (D and E): Curve D has a positive skew or is skewed right and curve E has a negative skew or is skewed left. Note that the values for mode, median, and mean are different with a skewed distribution.
- Kurtosis refers to how flat or peaked the curve is. for example, in Fig 3A , curve B is a normal distribution (excess kurtosis ~0, also called mesokurtic); curve A is peaked (excess kurtosis >0, also known as leptokurtic) compared with curve B and curve C is “flatter” with a lower central peak and broader (excess kurtosis <0, also known as platykurtic). All the three curves are symmetric and have the same mean, median, and mode values.
- An appropriate statistical test would be a parametric test such as a t -test or an analysis of variance (ANOVA).
- Data are usually represented as mean (SD).
- In Fig 3B , curves D and E are skewed.
- The terminology for skewness can be confusing. Curve D is said to be skewed right or has a positive skew. Curve E is skewed left or has a negative skew. The direction of the skew refers to the direction of the tail, not to where the bulk of the data are located.
- An appropriate statistical test would be a non-parametric test, such as Wilcoxon test or Mann-Whitney test.
- Data are usually represented as median, inter-quartile range (IQR).
- RANGE is the difference between the highest and the lowest values. Range can change drastically when the study is repeated. It is also dependent on sample size (range widens if more subjects are added) and is influenced by extreme values.
- INTERQUARTILE RANGE (IQR) is the range between the 25th and 75th percentiles or the difference between the medians of the lower half and upper half of the data and comprises the middle 50% of the data. IQR is less influenced by extreme values and is represented in a box plot.
- VARIANCE is a measure of dispersion or average deviation from the mean. It is the sum of the square of the deviation from the central value.
- Mean ± 1 SD: 68.2% of the sample is included
- Mean ± 2 SDs: 95.4% of the sample is included
- Mean ± 3 SDs: 99.8% of the sample is included
- The SD reflects how close individual scores cluster around the sample mean, whereas the SEM shows how close mean scores from repeated random samples will be to the true population mean.
- With increasing size of a random sample, the mean of the sample comes closer to the population mean.
3. Hypothesis Testing
Let us review study 1 and Fig 1 . This study has the following elements:
- Sample: Preterm infants in the NICU
- Predictor variable: Feeding
- Outcome variable: NEC
A simple hypothesis has one predictor and one outcome variable. For example, preterm infants who are exclusively fed human milk have a lower incidence of NEC is a simple hypothesis.
A complex hypothesis has more than one predictor variable. Preterm infants with maternal chorioamnionitis and exposure to indomethacin for patent ductus arteriosus (PDA) and formula feeds have a higher incidence of NEC is a complex hypothesis.
- Null hypothesis refers to restating the research hypothesis to one that proposes no difference between groups being compared. The statement that “there is no difference in the incidence of NEC in preterm infants fed human milk compared with preterm infants fed formula” is a null hypothesis.
- An alternative hypothesis proposes an association. Preterm infants who are exclusively fed human milk have a lower incidence of NEC is an alternative hypothesis.
- Preterm infants fed human milk have a lower incidence of NEC compared with formula-fed preterm infants is an example of a one-sided hypothesis.
- Preterm infants fed human milk have a different incidence of NEC compared with formula-fed preterm infants (increased risk or decreased risk) is an example of a two-sided hypothesis.
- One-sided hypotheses should be used only in unusual circumstances, when only one direction of the association is clinically or biologically meaningful. This is rarely used. For example, the use of vancomycin for late-onset sepsis is associated with a higher risk of red-man syndrome than placebo. It is highly unlikely that placebo use will have a higher incidence of red-man syndrome than vancomycin.
- Switching from a two-sided to a one-sided alternative hypothesis to reduce the P value is not appropriate.
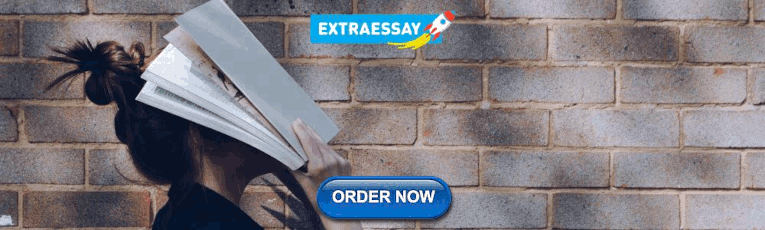
4. Statistical Tests
4a. parametric tests.
These tests assume the underlying population to be normally distributed and are based on means and SDs: the parameters of a normal distribution.
- Student’s t test is a simple, commonly used parametric test to compare two groups of continuous variables that are normally distributed.
- The t test compares the means of two groups and is based on the ratio of the difference between groups to the SE of the difference.

Paired and unpaired t test. Paired test compares the same patient/subject before and after an intervention (nutrition and weight). Each individual subject is compared with himself/herself. Two groups of patients are compared in an unpaired t test (weight gain in human milk–fed and formula-fed preterm infants).
- “Unpaired” t test: Two groups of patients/subjects are compared with each other. For example, Fig 4 comparing human milk–fed and formula-fed infants and comparing weight gain over the first 10 days after birth.
- One-tailed hypothesis: Only one direction of association will be tested. For example, assuming that weight at 10 days after birth will be greater than birthweight.
- Two-tailed hypothesis: Both directions of association will be tested. For example, weight may increase or decrease over the first 10 days after birth in preterm infants; for practical purposes, most t tests performed in neonatal research should be two-tailed, with rare exceptions.
- One-way ANOVA is an extension of the two-sample t test to three or more samples and deals with statistical test on more than two groups (eg, weight gain over the first 10 days after birth is compared among mother’s expressed milk–fed, donor milk–fed, and formula-fed preterm infants).
- The sum of squares representing the differences between individual group means and a second sum of squares representing variation within groups are analyzed.
- Planned comparisons are hypotheses specified before the analysis commences. Before the commencement of the study, it is hypothesized that weight gain over the first 10 days after birth is compared among small for gestational age, appropriate for gestational age, and large for gestational age will not be different and this analysis is planned.
- Post hoc comparisons are for further exploration of the data after a significant effect has been found. This analysis is occurring out of interest after the primary analysis has rejected the null hypothesis. For example, formula-fed infants gain more weight during the first 10 days after birth compared with mother’s or donor milk–fed infants. The investigator now wonders if increased weight gain with formula is observed only in infants who are small for gestational age and conducts a post hoc analysis.
- Factorial ANOVA performs complex analysis involving multiple independent factors. Additional information is derived from the interaction between factors.
- ANOVA repeated measures examines multiple (more than two) measures per subject that may be a result of more than one factor (eg, birth-weight is compared with weight at 10, 20, 30, and 40 days after birth and weight at discharge among breast-fed, donor milk–fed, and formula-fed preterm infants).
4B. Nonparametric Tests Make No Assumption About the Population Distribution
- (c) Wilcoxon rank test/Mann-Whitney U test are used to compare ordinal data. Human milk–fed and formula-fed preterm infants are compared based on the stage of NEC (Stage 0=no NEC, Stage I=nonspecific signs, Stage II=pneumatosis, and Stage III=intestinal perforation). These tests also can be used to analyze interval or ratio data that are not normally distributed.

Choosing the right statistical test. Some of the tests described in this flow diagram are not described in the text. The reader is referred to a textbook of biostatistics for a detailed description of these statistical tests.
Other Commonly Used Statistical Tests
Association Between Feeds and NEC
Disease → Exposure ↓ | Disease (NEC) Present | Disease (NEC) Not Present | Total |
---|
Exposure to formula present (formula feeds) | 5 (a) | 45 (b) | 50 (a + b) |
No exposure to formula (human milk feeds) | 1 (c) | 49 (d) | 50 (c + d) |
Total | 6 (a + c) | 94 (b + d) | 100 (a + b + c + d) |
A 2 × 2 contingency table. NEC=necrotizing enterocolitis.
- If the numbers are small (expected value is ≤5), an alternative test called Fisher’s exact test is used.
4C. Interpretation of P Value
Table 4 explains type I and type II errors during interpretation of a statistical test.
Type I and Type II Errors
True Condition (In the Population) |
---|
Trial study results | Therapies are different | Therapies are not different |
Therapies are different | True positive (correct decision) | False-positive (type I error) Probability = (same as value) |
Therapies are not different | False-negative (type II error) Probability = | True-negative (correct decision) |
Normally, α is 5% or 0.05 (setting a P value) and power is (1 − β ), often, 0.8 or 0.9 (80% or 90% power). P value and power are used in sample size calculations.
- TYPE I ERROR (false-positive, also known as a rejection error) is rejection of a null hypothesis that is actually true in the population. The investigator concludes that there is a significant difference between the groups when, in fact, there is no true difference. This risk can be reduced by setting a more stringent P value (eg, .01 instead of .05).
- TYPE II ERROR (false-negative, also known as an acceptance error) is failure to reject a null hypothesis that is actually false. The investigator concludes that there is no difference when a difference actually exists in the population. Increasing the sample size will reduce the risk of these errors.
- A lower P value (<.01) indicates a lower likelihood (1%) that the null hypothesis may be true due to chance alone.
- A lower P value does not infer a higher strength of association or clinical importance of an association.

Factors influencing P values. A large sample size, increased numeric difference between the means and less variability within the groups will reduce P value and increase statistical significance (A). A small sample size, small numeric difference between the means, and increased variability within the groups will increase P value and reduce statistical significance (B). This hypothetical example is comparing placebo (control group) and hydrocortisone (experimental group) for extubation of patients at risk for bronchopulmonary dysplasia and comparing diastolic blood pressure, as mentioned in study 2.
- Factors that tend to increase P value and decrease significance are small sample size, small difference between control and experimental means, and high variance/SD ( Fig 6B ).
- Interpreting P value when multiple comparisons have been made: When multiple comparisons are made, why not just do a bunch of t tests? If the probability of making a type I error on any one comparison is set at .05, it is important to recognize that a more stringent P value should be set if multiple comparisons are being performed (approximately, the P value is set at .05 ÷ the number of comparisons performed). This is called a Bonferroni correction .

Confidence interval. The true population mean (birthweight in this example) is shown by the black circle. The white circle represents the mean of a small sample (eg, your hospital deliveries). The 95% confidence interval is shown by the solid line (and is mean ± 1.96 × SEM). Increasing confidence from 95% to 99% will widen the range of the confidence interval to mean ± 2.58 × SEM). Increasing the sample size (eg, including all births in a county or state) will narrow the confidence interval.
- The values at either extreme of this range are called confidence limits .
- The probability of including the population mean within the confidence interval is the level of confidence, typically 95% confidence intervals are used in research. A higher level of confidence (99%) will widen the range of the confidence interval.
- 95% confidence interval is sample mean ± 1.96 SEM. As mentioned earlier, SEM = SD/√ n , where n is the sample size. A 99% confidence interval is sample mean ± 2.58 SEM (and hence a wider confidence interval compared with the 95% confidence interval).
- Based on this equation, larger sample size ( n ), and smaller SD will narrow the confidence interval.
- When expressing relative risk or odds ratio (OR), 95% or 99% confidence limits are mentioned. The interpretation of these confidence intervals is described in the next section.
5. Measures of Association
Table 3 demonstrates the association between formula and human milk feeds with NEC. The probability of developing NEC in formula-fed preterm infants is a/(a + b) or 5/50 = 0.1. The odds of developing NEC are a/b or 5/45 = 0.11. The probability of developing NEC among human milk–fed preterm infants is c/(c + d) or 1/50 = 0.02. The odds of developing NEC among human milk–fed infants is c/d or 1/49 = 0.0204. So, probability and odds approximate each other if the outcome is rare.
- The absolute risk of NEC following exposure to formula is a/(a + b) = 5/50 = 0.1
- The absolute risk of NEC following exposure to human milk is c/(c + d) = 1/50 = 0.02
- Absolute risk reduction = a/(a + b) − c/(c + d) = 0.1 − 0.02 = 0.08
- This measure of association is also called risk difference or attributable risk .
- Number needed to treat = 1 ÷ (a/[a + b] − c/[c + d]) = 1 ÷ (0.1 – 0.02) = 1/0.08 = 12.5 ( Table 3 )
- Feeding 12.5 babies with exclusive human milk feeds will reduce one case of NEC.
- Relative risk reduction = (NEC event rate in pre-term infants not exposed to formula − NEC event rate in preterm infants exposed to formula) ÷ (NEC event rate in preterm infants not exposed to formula) = (0.02 − 0.1) ÷ 0.02 = (−0.08) ÷ 0. = −024
- A negative number indicates relative risk reduction and a positive number indicates relative risk increase.
- Relative risk = (a/[a + b]) ÷ (c/[c + d]) = 0.1 ÷ 0.02 = 5; a formula-fed preterm infant is five times more likely to develop NEC compared with a preterm infant not exposed to formula.
- Relative risk is also known as risk ratio or rate ratio .
- If relative risk = 1, there is no association between exposure and outcome.
- Relative risk >1 indicates a positive association and <1 indicates a negative association between exposure and outcome.
- OR ( Table 3 ) = a/b ÷ c/d = 5/45 ÷ 1/49 = 5.44
- Relative risk is easier to understand than OR
- Permits subgroup analysis
- OR can be adjusted for confounders (such as gestational age)
Case-Control Study
Fifty patients with necrotizing enterocolitis (NEC) are matched with 90 control subjects who do not have NEC. Both groups are evaluated with respect to exposure to formula feeds.
- OR approximates relative risk if the event rates in the whole population are uncommon.
- As the magnitude of risk (event rates) in the unexposed population increases, then OR will NOT approximate relative risk.
- Similar to relative risk, an OR of 1 indicates no association between exposure and outcome. An OR more than 1 indicates positive association and less than 1 indicates negative association.
- Confidence intervals (typically at 95%) for relative risk and OR indicate that the investigator can be 95% confident that the real relative risk or OR in the population lies within this range of values. If the 95% confidence interval crosses 1, the association is not significant. If both the confidence limits exceed 1 (for example, 2.7 to 7.2), there is a significant positive association between exposure and outcome. If both the confidence limits are less than 1, there is a significant negative association between exposure and outcome (for example, 0.6 to 0.92). If the confidence limits cross 1 (for example, 0.98 to 1.75), there is no significance.
- Correlation coefficient can range from −1 to +1. A positive value, 0.78 for example, indicates a positive relationship and a negative value indicates a negative relationship (if one variable increases, the other decreases).
- The regression line is the straight line passing through the data that minimizes the sum of the squared differences between the original data and the fitted points.
- The strength of correlation is dependent on the slope of the regression line. If the value is close to 1 (or −1), the regression line has a steep slope and the correlation is high; if correlation coefficient is 0, the two variables are independent of each other.
- Assumes that each variable is normally distributed (works if one of the variables is binary)
- Measures linear relationship only
- Affected by variances of the variable in addition to association
- Extreme values or pairs are highly influential
- Cannot assess for nonlinear relationships
- Increasing sample size leads to “significance” at lower r values. For example, If n = 40, r > 0.7 may provide significance; but if n > 400, r = 0.15, may provide significance.
- The estimated correlation should only not be extrapolated beyond the observed range of the variables, the relationship may be different outside this region.
- Correlation does not equal causation.
Hazard ratio
- Hazard ratio is a measure of relative risk over time in circumstances in which we are interested not only in the total number of events, but in their timing as well. The timing may be represented as child months or line days and so forth.
- The event of interest may be death or it may be a nonfatal event, such as readmission, line infection, or symptom change.
6. Regression Analysis
Regression analysis is a method used to explore the nature of relationship between two continuous random variables. Regression allows us to estimate the degree of change in one variable ( response variable ) to a unit change in the second variable ( explanatory variable ).
where “y” is the mean head circumference at gestational age of “x” weeks;
β 0 is the y-intercept (mean value of the response y when x = 0, although this is not clinically applicable for the current example);
β 1 is the slope of the line (change in mean value of y that corresponds to a 1 unit increase in x); and
ε is the distance of a given observation from the population regression line (because y is the mean head circumference, each infant’s head circumference will be scattered around the mean and not necessary exactly equal to the mean).
This is the simple linear regression equation and can be used when the relationship between the two variables is roughly linear. Multiple regression involves the linear relationship between one dependent variable, for example, presence or absence of bronchopulmonary dysplasia and multiple explanatory variables (eg, duration of mechanical ventilation, duration of oxygen exposure, infection, nutrition, gestational age). The equation for multiple regression is
- The variables cannot be plotted on a straight line but may have a sigmoid configuration and need logistic transformation (change to an equation to the power of “e,” where “e” is the base of natural logarithm).
The interpretation of β0 and β1 are in the log odds scale.
- The response value of interest is the amount of time from an initial observation to the occurrence of an event. In addition to studies evaluating change in mortality, survival analysis can be used in studies in which time to an event is an outcome; for example, time to relapse after chemotherapy for a malignancy.

Interpretation of a Kaplan-Meier curve (based on Mah D, Singh TP, Thiagarajan RR, et al. Incidence and risk factors for mortality in infants awaiting heart transplantation in the USA. J Heart Lung Transplant . 2009;28(12):1292–1298; ticks/ notches are added to the original curve for educational purposes) ECMO=extracorporeal membrane oxygenation.
- A common method used is the product limit method (also called the Kaplan-Meier method ). This is a nonparametric technique (no assumption about the distribution of population, not smooth) that uses the exact survival time for each subject in a sample (instead of grouping the times into intervals, Fig 8 ).
- Proportional hazards assumption (Cox): In the Cox proportional hazards assumption, curves will not cross; risk of relapse in one group is a fixed proportion of the risk in the other group. Curves are less reliable as the number of subjects decreases (so may cross toward the end even if the proportional hazards assumption is mostly true). The proportional hazards model can assess the effects of multiple covariates on survival.
7. Diagnostic Tests
- Incorporation bias: If any symptoms, signs, or laboratory tests used to diagnose a disease are used as part of the gold standard (such as the Centers for Disease Control and Prevention’s definition of ventilator-associated pneumonia in infants that includes chest X-ray, hypoxia, temperature instability, abnormal white count, and so forth), a study comparing one of these components (such as leukopenia) to that gold standard can make them look falsely good. Hence, it is important to have an independent gold standard while evaluating a diagnostic test.
- If the gold standard is imperfect, it can make a test look either worse or better than it really is.
- If the test has continuous results (like CRP), a cutoff point (such as CRP >10 mg/L) is necessary to define a positive test.
Calculation of Sensitivity, Specificity, PPV, and NPV
- Disease is the first row (on the top) and test is the first column by convention.
- The numerator for predictive values and sensitivity/ specificity is always a TRUE value (TN or TP).
- Sensitivity/specificity are calculated along columns and rows are associated with predictive value calculations (see above).
- A highly sensitive test is used to rule out disease (SnOUT): low false-negative rate.
- A highly specific test is used to rule in disease (SpIN): low false-positive rate.
NPV=negative predictive value, PPV=positive predictive value.

A. Sensitivity, specificity, and positive predictive value (PPV) and negative predictive value (NPV), the gold standard for diagnosis, identifies patients with disease (shown as gray subjects) located in the yellow square. Subjects without disease are shown in white color. The diagnostic test is positive in subjects located inside the pink circle. B. The impact of reduced disease prevalence on PPV and NPV. The number of subjects with the disease (based on the gold standard test) decreases secondary to reduced prevalence. Because of a reduction in the number of true positive (TP) subjects, PPV decreases. NPV increases because of a decrease in false-negative (FN) subjects. Sensitivity and specificity are not influenced by disease prevalence. The effect of increased prevalence is opposite of the change in predictive values associated with reduced disease prevalence and is shown in Table 7 (increased PPV and decreased NPV).
- A highly sensitive test has a low false-negative rate and is good as a screening test and a negative test almost rules out the disease.
- It is calculated as true-positives ÷ (true-positives + false-negatives, ie, all subjects with the disease).
- A highly specific test has a low false-positive rate.
- It is calculated as true-negatives ÷ (false-positives + true-negatives, ie, all subjects without the disease).
- Positive predictive value (PPV) is the proportion of subjects with positive tests who have the disease. It is calculated by true-positives ÷ (true-positives + false-positives, ie, all subjects who tested positive with the test).
- Negative predictive value (NPV) is the proportion of subjects with negative tests who do not have the disease. It is calculated by true-negatives ÷ (false-negative + true-negative).
- Sensitivity and specificity are prevalence-independent test characteristics, as their values are intrinsic to the test and do not depend on the disease prevalence in the population of interest.
Effect of Increased Prevalence of Disease on Sensitivity, Specificity, and Predictive Values
Upper case letters in bold indicate increased number due to increased disease prevalence. NPV, negative predictive value, PPV=positive predictive value.
- Reduced disease prevalence will decrease PPV and increase NPV.

Receiver operator characteristic (ROC) curves for a good test (shown with green dashed line) and a poor/ worthless test (shown with red dashed line). The area under the curve provides a measure of the capability of the test. The area under curve for the good test is shown with green dots and is close to 1.0. The area under the curve for the poor test is close to 0.5 (50% chance of diagnosing the disease) and is shown by a red shade.
- The area under the ROC curve is a useful summary of the overall accuracy of a test.
- The area under the ROC curve ranges from 0.5 (a diagonal from lower-left to upper-right corner and a useless test) to 1.0 (a curve along the left and upper borders for a perfect test).
- For dichotomous tests, the likelihood ratio for a positive test is sensitivity/(1-specificity) and the likelihood ratio of a negative test is (1−sensitivity/ specificity).
- For example, if 19% of neonates with serious bacterial infection and 0.52% of neonates without a serious bacterial infection have a white count less than 5,000/ μ L, the likelihood ratio for serious bacterial infection in a neonate with leukopenia is 19/0.52 = 36. ( 7 )
- The probability of the disease is known from clinical history and status and existing literature before the test is the pretest odds or prior odds . For example, assume that the pre–complete blood count test odds for an African American infant born at 35 weeks by vaginal delivery to have early-onset sepsis is 1/1,000 live births. If a complete blood count is performed at 6 hours after birth and the white blood cell count is less than 5,000/ μ L, the posttest odds or posterior odds of this infant having a serious bacterial infection is 36/1,000 live births.
- Posterior odds (posttest odds) = Prior odds × Likelihood ratio
- The goal is to improve clinical decisions using mathematical methods involving multivariate techniques. Points can be assigned to various risk factors, signs, and symptoms to derive a predictive score.
- An alternative approach is to create a decision tree by using a series of yes/no questions and is called recursive partitioning or classification and regression tree analysis. These techniques are being used to predict the risk of sepsis in neonates more than 34 weeks’ gestation ( 8 )( 9 ) and optimize use of antibiotics for suspected early-onset sepsis in neonates.
- Internal validity (within the study sample) can be tested by dividing the cohort used to derive the clinical prediction rule into derivation (one-half to two-thirds of the sample) and validation data sets. The rule derived from the derivation cohort is then tested in the validation cohort. ( 8 )
- External validity is assessed by prospective validation by testing the rule in different populations.
Conclusions
A basic understanding of biostatistics is necessary for a neonatal practitioner. This is useful in interpretation of studies and journal articles. Clinicians conducting research require a thorough knowledge of biostatistics. This review is intended to provide a quick overview of biostatistics for trainees or as a refresher for neonatologists during preparation for a pediatric subspecialty board certification.
American Board of Pediatrics Neonatal-Perinatal Content Specifications
- Distinguish types of variables (eg, continuous, categorical, ordinal, nominal).
- Understand how the type of variable (eg, continuous, categorical, nominal) affects the choice of statistical test).
- Understand how distribution of data affects the choice of statistical test.
- Differentiate normal from skewed distribution of data.
- Understand the appropriate use of the mean, median, and mode.
- Understand the appropriate use of standard deviation (SD).
- Understand the appropriate use of standard error (SE).
- Distinguish the null hypothesis from an alternative hypothesis.
- Interpret the results of hypothesis testing.
- Understand the appropriate use of the χ 2 test versus a t test.
- Understand the appropriate use of analysis of variance (ANOVA).
- Understand the appropriate use of parametric (eg, t test, ANOVA) versus nonparametric (eg, Mann-Whitney U , Wilcoxon) statistical tests.
- Interpret the results of χ 2 tests.
- Interpret the results of t tests.
- Understand the appropriate use of a paired and nonpaired t test.
- Determine the appropriate use of a one-versus two-tailed test of significance.
- Interpret a P value ( probability of the null hypothesis being true by chance alone).
- Interpret a P value when multiple comparisons have been made.
- Interpret a confidence interval.
- Identify a type I error.
- Identify a type II error.
- Differentiate relative risk reduction from absolute risk reduction.
- Calculate and interpret a relative risk.
- Calculate and interpret an odd ratio (OR).
- Understand the uses and limitations of a correlation coefficient.
- Identify when to apply regression analysis (eg, linear, logistic).
- Interpret a regression analysis (eg, linear, logistic).
- Identify when to apply survival analysis (eg, Kaplan-Meier).
- Interpret a survival analysis (eg, Kaplan-Meier).
- Recognize the importance of an independent “gold standard” in evaluating a diagnostic test.
- Calculate and interpret sensitivity and specificity.
- Calculate and interpret positive predictive values (PPVs) and negative predictive values (NPVs).
- Understand how disease prevalence affects the PPV and NPV of a test.
- Calculate and interpret likelihood ratios.
- Interpret a receiver operator characteristic (ROC) curve.
- Interpret and apply a clinical prediction rule.
Abbreviations
ANOVA | analysis of variance |
CRP | C-reactive protein |
ECMO | extracorporeal membrane oxygenation |
IQR | interquartile range |
NEC | necrotizing enterocolitis |
NPV | negative predictive value |
OR | odds ratio |
PDA | patent ductus arteriosus |
PPV | positive predictive value |
ROC | receiver operator characteristic |
Author Disclosure
Drs Manja and Lakshminrusimha have disclosed funding from 1R01HD072929-0 (SL), and that they are consultants and on the speaker bureau of Ikaria, Inc. This commentary does not contain a discussion of an unapproved/ investigative use of a commercial product/ device.
Suggested Reading
We’re fighting to restore access to 500,000+ books in court this week. Join us!
Internet Archive Audio

- This Just In
- Grateful Dead
- Old Time Radio
- 78 RPMs and Cylinder Recordings
- Audio Books & Poetry
- Computers, Technology and Science
- Music, Arts & Culture
- News & Public Affairs
- Spirituality & Religion
- Radio News Archive
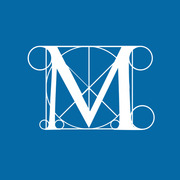
- Flickr Commons
- Occupy Wall Street Flickr
- NASA Images
- Solar System Collection
- Ames Research Center
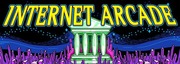
- All Software
- Old School Emulation
- MS-DOS Games
- Historical Software
- Classic PC Games
- Software Library
- Kodi Archive and Support File
- Vintage Software
- CD-ROM Software
- CD-ROM Software Library
- Software Sites
- Tucows Software Library
- Shareware CD-ROMs
- Software Capsules Compilation
- CD-ROM Images
- ZX Spectrum
- DOOM Level CD

- Smithsonian Libraries
- FEDLINK (US)
- Lincoln Collection
- American Libraries
- Canadian Libraries
- Universal Library
- Project Gutenberg
- Children's Library
- Biodiversity Heritage Library
- Books by Language
- Additional Collections

- Prelinger Archives
- Democracy Now!
- Occupy Wall Street
- TV NSA Clip Library
- Animation & Cartoons
- Arts & Music
- Computers & Technology
- Cultural & Academic Films
- Ephemeral Films
- Sports Videos
- Videogame Videos
- Youth Media
Search the history of over 866 billion web pages on the Internet.
Mobile Apps
- Wayback Machine (iOS)
- Wayback Machine (Android)
Browser Extensions
Archive-it subscription.
- Explore the Collections
- Build Collections
Save Page Now
Capture a web page as it appears now for use as a trusted citation in the future.
Please enter a valid web address
- Donate Donate icon An illustration of a heart shape
Biometry; the principles and practice of statistics in biological research
Bookreader item preview, share or embed this item, flag this item for.
- Graphic Violence
- Explicit Sexual Content
- Hate Speech
- Misinformation/Disinformation
- Marketing/Phishing/Advertising
- Misleading/Inaccurate/Missing Metadata
![[WorldCat (this item)] [WorldCat (this item)]](https://archive.org/images/worldcat-small.png)
plus-circle Add Review comment Reviews
189 Previews
4 Favorites
DOWNLOAD OPTIONS
No suitable files to display here.
EPUB and PDF access not available for this item.
IN COLLECTIONS
Uploaded by associate-tara-maharjan on February 11, 2014
SIMILAR ITEMS (based on metadata)

- Department of Genetics
- Vision & Mission
- Contact Details
- Academic Staff
- Technical Staff
- Administrative and Service Staff
- Research Staff
- Post Doctorates
- Plant Breeding
- Postgraduate
- Animal Genetics
- Human Genetics
- Plant Genetics
- Research Facilities
- Recent Publications
- Publications Archive
- Dissertations & Theses
- Social Impact Projects
- School Outreach Programs
- Living Gardens Social Impact Project
- Agricultural Economics
- Animal Sciences
- Conservation Ecology
- Food Science
- Forestry & Wood Science
- Staff - Genetics Students
- Staff - Genetics Personel
- Genetics Homepage Carousel
- Staff & Students
- Publications
- Useful links
- Genetics Department Contact Details
- Postgraduate Students
- Academic Staff (C1)
- Undergraduate
- Horticultural Sciences
- Institute for Plant Biotechnology
- Institute for Wine Biotechnology
- Plant Pathology
- Postharvest Technology
- Soil Science
- Standard Bank Centre
- Viticulture & Oenology
212 (8) Introductory Biometry (2L, 1T / 1P)
Flexible assessment P Mathematics (Bio) 124 or Mathematics 114
Module co-ordinator: Mr S van der Westhuizen
Lecturers: Mr S van der Westhuizen and Ms Samantha Joao
242 (8) Applications in Biometry (2L, 1T / 1P)
Treatment and experimental design; efficiency of estimation; analysis of variance; hypothesis tests for means and differences between means: F-test, t-test, Student’s LSD; confidence intervals; non-parametric tests; multiple linear regression. All data will be analysed using applicable software.
Flexible assessment P Biometry 212
Lecturers: Mr S van der Westhuizen and Ms Samantha Joao
311 (8) Advanced regression and ANOVA (1L, 1P, 1T)
Matrix algebra; generalized linear models; power analysis; simple- and multiple linear regression; polynomial regression; logistical regression; diagnostic tests for influential observations; analysis of covariance; testing model assumptions.
Flexible assessment P Biometry 242
Module Co-ordinator: Mr S van der Westhuizen
Lecturer: Mr S van der Westhuizen
711 (8) and 811 (8) Biometrical applications and data analysis in SAS
Data processing and graphical procedures with SAS Enterprise Guide. Simple descriptive statistics; t-tests for single populations, independent samples t-tests and paired t-tests for two populations; analysis of variance: completely random design, random-blocks design, Latin-square design, cross-classification designs; repeated-measures analysis of variance; multiple comparison procedures. Power analysis. Non-parametric tests: Mann-Whitney, Wilcoxon, Kruskal-Wallis and Friedman; linear regression and correlation; polynomial regression, multiple regression; selection of independent variables with stepwise regression and all-subset regression; covariance analysis; categorical data analyses (Chi-squared tests); logistic regression. This module is presented in two blocks of five half days each in the first semester.
Flexible assessment. P Biometry 212 and 242, 211. Students with different undergraduate Statistics modules must obtain at least 50% for an admission examination.
Module co-ordinator and lecturer: Ms Samantha Joao
721 (8) and 821 (8) Biometrical applications and data analysis in R
Data processing and graphical procedures with R. Simple descriptive statistics; t-tests for single populations, independent samples t-tests and paired t-tests for two populations; analysis of variance: completely random design, random-blocks design, Latin-square design, cross-classification designs; repeated-measures analysis of variance; multiple comparison procedures. Power analysis. Non-parametric tests: Mann-Whitney, Wilcoxon, Kruskal-Wallis and Friedman; linear regression and correlation; polynomial regression, multiple regression; selection of independent variables with stepwise regression and all-subset regression; covariance analysis; categorical data analyses (Chi-squared tests); logistic regression. This module is presented in two blocks of five half days each in the first semester.
Module co-ordinator and lecturer: Mr S van der Westhuizen
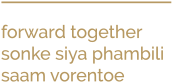
- Careers @ SU
- Lodge a complaint
All rights reserved © 2024 Stellenbosch University Private Bag X1, Matieland, 7602, Stellenbosch, South Africa Tel.: +27 21 808 9111
- DOI: 10.2307/2344763
- Corpus ID: 84065806
Fundamentals of biometry
- L.N.BALAAM , George Allen , Unwin
- Published 1 July 1973
- Biology, Mathematics
22 Citations
A study of atherosclerosis regression in macaca mulatta. i. design of experiment and lesion induction., effect of a respiratory gas collection mask on some measurements of cardiovascular and respiratory function in horses exercising on a treadmill., varied immunological reactivity of factor viii from animal plasmas., spectrophotometric analysis of some biochemical constitutuents of waste muga pupae, using the normal distribution, the ocean abysses witnessed the origin of the genetic code..
The ocean abysses witnessed the origin of the genetic code
Changes in acquisition of a sequence of motor conditioned reflexes by rats after hippocampal lesions, structuring of the genetic code took place at acidic ph., aspirin and tartrazine oral challenge: incidence of adverse response in chronic childhood asthma., 15 references, a theoretical appraisal of the relative merits of 50 % hybrid and synthetic varieties of grasses, the accuracy of a range of capacitance probe methods for estimating pasture yields, a mathematical model of pasture contamination by grazing cattle and the effects on herbage intake, ripening in maize: interrelationships between time, water content and weight of dry material in ripening grain of a flint × dent hybrid (inra 200), effect of rams on the onset of breeding activity in clun forest ewe lambs, ripening of grain maize in england: varietal differences in ripening patterns, copper, molybdenum and sulphur contents of oats and barley in relation to chronic copper poisoning in housed sheep, the effects of the long-term application of a wide range of nitrogen rates on the yields from perennial ryegrass swards with and without white clover, spacing and harvest date experiments with maris peer potatoes, changes in magnesium and calcium in soils of the broadbalk wheat experiment at rothamsted from 1865 to 1966, related papers.
Showing 1 through 3 of 0 Related Papers
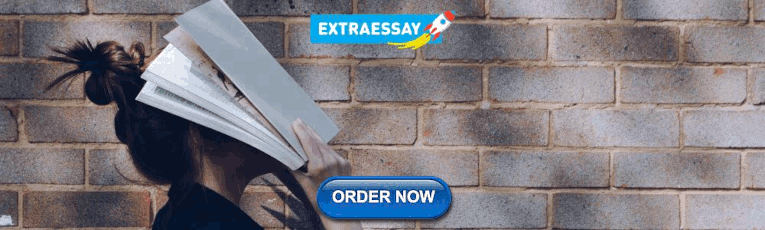
IMAGES
COMMENTS
The Principles and Pr actice of Statistics. in Biological Research. FOURTH EDITION. Robert R. Sokal and F. James Rohlf. Stony Brook University. 3620002FM.indd iii 3620002FM.indd iii 8/5/11 1:07 ...
dokumen.tips_bio-212-research-methods-and-biometry-biometry-biometrics-or-biostatistics-is-the - Free download as PDF File (.pdf), Text File (.txt) or read online for free.
STEP7BY7STEP!ANALYSISOFBIOLOGICALDATA!! 3! Step-by-step analysis of biological data Here I describe how you should determine the best way to analyze your biological experiment. How to determine the appropriate statistical test
The Principles of Biology sequence (BI 211, 212 and 213) introduces biology as a scientific discipline for students planning to major in biology and other science disciplines. Laboratories and classroom activities introduce techniques used to study biological processes and provide opportunities for students to develop their ability to conduct research.
Materials and Methods: Routine parasitological procedures were employed to screen the gills of the fishes for the monogenean infection and standard statistical softwares (IBM SPSS 21.0 version ...
1. Introduction. Biometry is a large and complex field that arises from the application of statistics and mathematics to biology. All phases of research in biology, including design and data collection, analysis, and interpretation of results, depend on statistical principles and statistical methods.
The book will be essential reading for undergraduate and graduate students, professional researchers, and informed managers of natural resources. Marcel Rejmánek, Department of Evolution and Ecology, University of California, Davis, CA, USA. Cambridge University Press 978-1-108-48038-3 — Biostatistics with R Jan Lepš , Petr Šmilauer ...
Neuroimage, Genome Biology I Methods: Biometrics, Annals of Applied Statistics, Biostatistics, Statistics in Medicine, Neuroimage, Genome Biology Modern methods papers use simulation studies to illustrate statistical properties; we will often do the same. Most PhD theses \resemble" methods papers, and contain material similar to that discussed ...
Data in Biology; 3. Computers and Data Analysis; 4. Descriptive Statistics ... Meta-Analysis and Miscellaneous Methods Mathematical Appendix. (source: Nielsen Book Data) Publisher's summary This easily understood but rigorous introduction to biological statistics is a standard text and valuable reference for anyone doing scientific research ...
About this book. Statistical methods are becoming more important in all biological fields of study. Biometry deals with the application of mathematical techniques to the quantitative study of varying characteristics of organisms, populations, species, etc. This book uses examples based on genuine data carefully chosen by the author for their ...
This chapter discusses statistical power and sample size in the Analysis of Variance, as well as meta-Analysis and Miscellaneous Methods, and some of the methods used in meta-analysis. 1. Introduction 2. Data in Biology 3. Computers and Data Analysis 4. Descriptive Statistics 5. Introduction to Probability Distributions 6. The Normal Probability Distribution 7. Hypothesis Testing and Interval ...
Welcome to the third edition of the Handbook of Biological Statistics!This online textbook evolved from a set of notes for my Biological Data Analysis class at the University of Delaware. My main goal in that class is to teach biology students how to choose the appropriate statistical test for a particular experiment, then apply that test and interpret the results.
This book is a reference work, definitively not appropriate for reading it from start to end, and a reader who wants to know more about the recent approaches to the analysis of biological data should consult additional textbooks. This is a classical textbook of biometry, one of the best available. Written for the biologists, it is, in fact, much more readable than what one would expect from ...
Improvisation in Biology teaching Methods/strategies of teaching Biology e.g. discussion, lecture+, demonstration, small group ... BIO 212 COURSE TITLE: Research Methods and Biometry COURSE OUTLINE - Meaning, Purpose and relevance of Research and Biometry - Types of Research (Experimental, Survey, Case Study etc.) - Choice of Research Topic
Bibliography Includes bibliographical references (p. 850-857) and index. Contents. Introduction - Data in Biology - The Handling of Data - Descriptive Statistics - Introduction to Probability Distributions: Binomial and Poisson - The Normal Probability Distribution - Estimation and Hypothesis Testing - Introduction to Analysis of Variance - Single Classification Analysis of Variance - Nested ...
Abstract. Collecting, analyzing, and interpreting data are essential components of biomedical research and require biostatistics. Doing various statistical tests has been made easy by sophisticated computer software. It is important for the investigator and the interpreting clinician to understand the basics of biostatistics for two reasons.
Access-restricted-item true Addeddate 2014-02-11 17:29:59.564729 Associated-names Rohlf, F. James, 1936- Bookplateleaf
Biostatistics is a branch of applied statistics with applications in many areas of biology including epidemiology, medical sciences, health sciences, educational research and environmental sciences. The principles and methods of statistics, which is the science that deals with the collection, classification, analysis, and interpretation of ...
Introduction. Bioinformatics, Biometrics, and Biostatistics are well- known broad concepts in the field of analytics for life sciences. Bioinformatics is frequently linked to Quantitative Biology or Computational Biology [1]. Bioinformatics is the journal of the International Society of Computational Biology, which demonstrates the former link.
Biometry. 212 (8) Introductory Biometry (2L, 1T / 1P) Role of statistics in research; methods of tabulation and graphical representation of data; descriptive measures of locality, variation and association; the elementary principles of estimation, sampling, randomization, unbiasedness and distributions; simple linear and non-linear regression ...
• Identify appropriate statistical methods to be applied in a given research setting, apply these methods, and acknowledge the limitations of those methods; ... Biostatistics Department, 722 W 168th Street, 6th floor, rm 635 Email: [email protected] or [email protected] Phone: (212) 305-9405 (I prefer the use of email) Fax: (212) 305-9408
Fundamentals of biometry. L.N.BALAAM, George Allen, Unwin. Published 1 July 1973. Biology, Mathematics. TLDR. A fundamental course in biometry, an essential part of the training of all biological scientists, cannot be presented without the necessary mathematical tools, and these are introduced gradually within the text. Expand.
ESSENTIALS OF BIOSTATISTICS & RESEARCH METHODOLOGY - 3rd Edition. October 2020. Edition: Third Edition. Publisher: Academic Publishers. ISBN: 9789387162662. Authors: Indranil Saha. ICMR-Centre for ...
The effects of the culture method and harvest time (month) on meat yield of the mussels were tested with generalized linear model (GLM, identity link function). The main effects and interactions of the culture method (pipe and buoy) and harvest time (12 months) were specified. Additionally, mussel size was used as covaries in the model.